Tokenizer
nlp任务的输入都是raw text,model的输入需要是inputs id,所以tokenzier将句子转换成inputs id,怎么转换呢,有3种方式:
word-based
split the text:
按照空格来区分
按照标点来区分
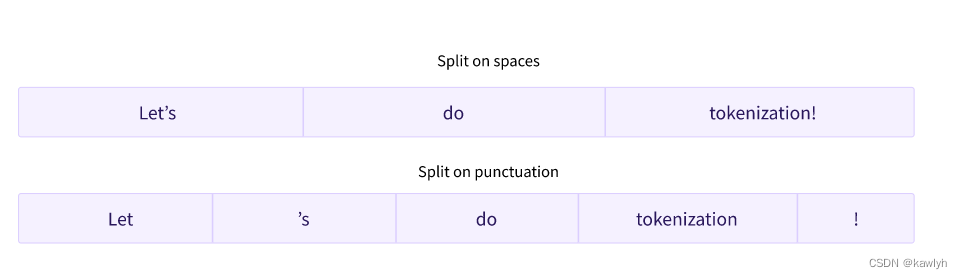
我们会得到一个非常大的词表,Each word gets assigned an ID, starting from 0 and going up to the size of the vocabulary.
问题1:词表太大了,负担过重
问题2:相似词没有做区分,例如dog与dogs他们的标号不一样
我们可以限制词表为最常出现的10000个词组成,若不在词表中的词可以用[UNK]或者 表示。
问题1:如果词表设置的太小的话,那么会有太多[UNK]词,显然会影响训练效果
character-based
将text划分为字母
好处:
词表变小了:例如只有26个字母和一些特殊字符
没有未知词[UNK]了
坏处:
每个字符没有啥意义(但是因语言而异,例如中文汉字比拉丁字符的意义多)
每个单词的tokenizer数目变多了,例如good采用word-base时只有一个tokenizer,但是使用character-based时有4个tokenizer
Subword tokenization(推荐)
频繁使用的单词不应该被拆分成较小的子单词,而罕见的单词应该被分解成有意义的子单词。

这样词表小了,[UNK]词也少了,而且近似词之间还有联系
补充
Byte-level BPE, as used in GPT-2
WordPiece, as used in BERT
SentencePiece or Unigram, as used in several multilingual models
创建tokenizer
特定类型的创建
from transformers import BertTokenizer
tokenizer = BertTokenizer.from_pretrained("bert-base-cased")
自动创建(推荐)
from transformers import AutoTokenizer
tokenizer = AutoTokenizer.from_pretrained("bert-base-cased")
例子
tokenizer("Using a Transformer network is simple")
{'input_ids': [101, 7993, 170, 11303, 1200, 2443, 1110, 3014, 102],
'token_type_ids': [0, 0, 0, 0, 0, 0, 0, 0, 0],
'attention_mask': [1, 1, 1, 1, 1, 1, 1, 1, 1]}
保存tokenizer
tokenizer.save_pretrained("directory_on_my_computer")
encoding
把text变为input ids就是encoding,步骤:
split the text into words,也叫tokens
from transformers import AutoTokenizer
tokenizer = AutoTokenizer.from_pretrained("bert-base-cased")
sequence = "Using a Transformer network is simple"
tokens = tokenizer.tokenize(sequence)
print(tokens)
['Using', 'a', 'transform', '##er', 'network', 'is', 'simple']
convert those tokens into numbers,使用我们from_pretrained下载的词汇表
ids = tokenizer.convert_tokens_to_ids(tokens)
print(ids)
[7993, 170, 11303, 1200, 2443, 1110, 3014]
decoding
把tokens变成text
decoded_string = tokenizer.decode([7993, 170, 11303, 1200, 2443, 1110, 3014])
print(decoded_string)
'Using a Transformer network is simple'