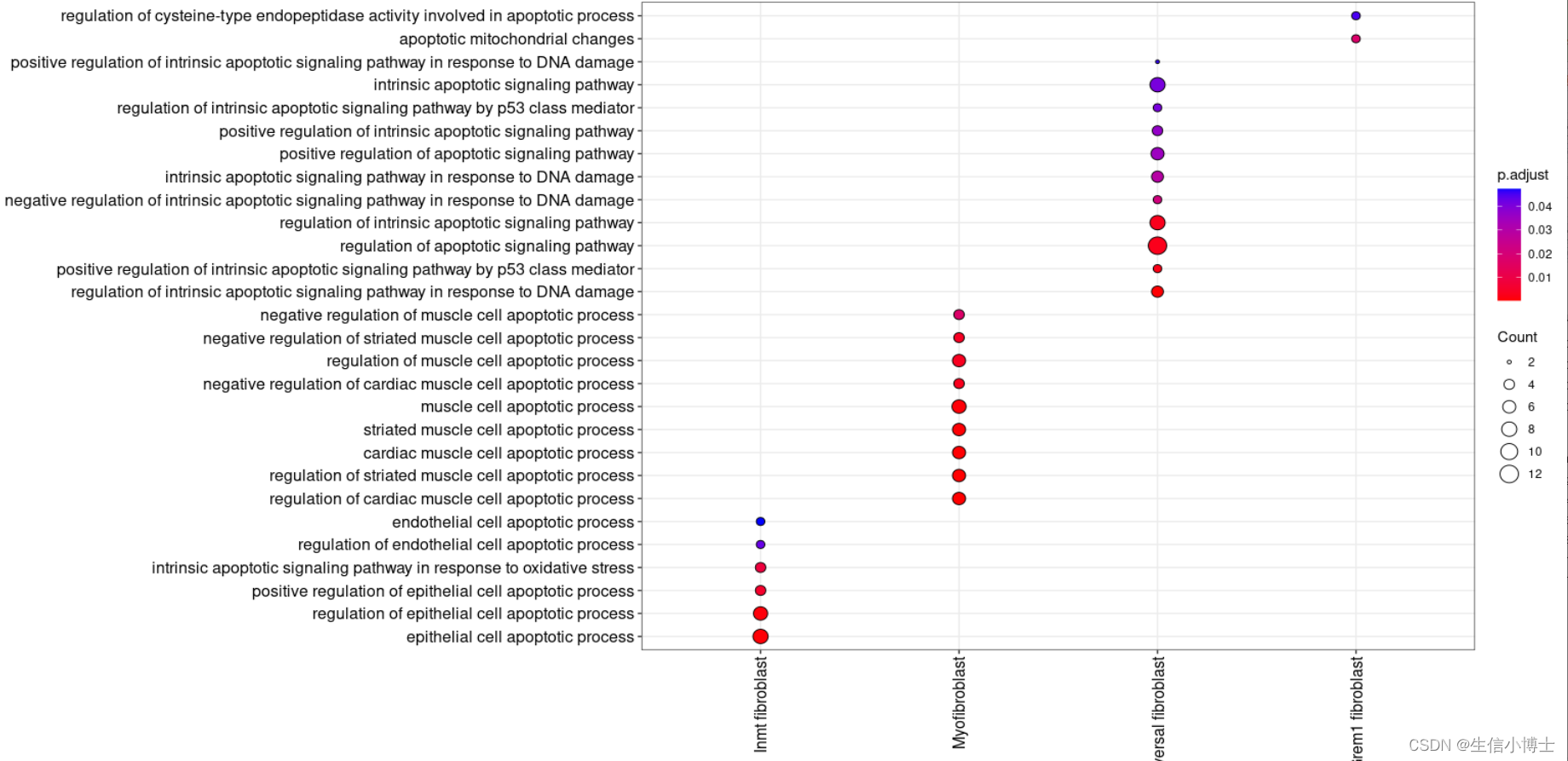
#2.2挑选term---
selected_clusterenrich=enrichmets[grepl(pattern = "cilium|matrix|excular|BMP|inflamm|development|muscle|
vaso|pulmonary|alveoli",
x = enrichmets$Description),]
head(selected_clusterenrich)
distinct(selected_clusterenrich)
# remove duplicate rows based on Description 并且保留其他所有变量
distinct_df <- distinct(enrichmets, Description,.keep_all = TRUE)
library(ggplot2)
ggplot( distinct_df %>%
dplyr::filter(stringr::str_detect(pattern = "cilium|matrix|excular|BMP|inflamm|development|muscle",Description)) %>%
group_by(Description) %>%
add_count() %>%
dplyr::arrange(dplyr::desc(n),dplyr::desc(Description)) %>%
mutate(Description =forcats:: fct_inorder(Description))
, #fibri|matrix|colla
aes(Cluster, Description)) +
geom_point(aes(fill=p.adjust, size=Count), shape=21)+
theme_bw()+
theme(axis.text.x=element_text(angle=90,hjust = 1,vjust=0.5),
axis.text.y=element_text(size = 12),
axis.text = element_text(color = 'black', size = 12)
)+
scale_fill_gradient(low="red",high="blue")+
labs(x=NULL,y=NULL)
# coord_flip()
head(enrichmets)
ggplot( distinct(enrichmets,Description,.keep_all=TRUE) %>%
# dplyr::mutate(Cluster = factor(Cluster, levels = unique(.$Cluster))) %>%
dplyr::mutate(Description = factor(Description, levels = unique(.$Description))) %>%
# dplyr::group_by(Cluster) %>%
dplyr::filter(stringr::str_detect(pattern = "cilium organization|motile cilium|cilium movemen|cilium assembly|
cell-matrix adhesion|extracellular matrix organization|regulation of acute inflammatory response to antigenic stimulus|
collagen-containing extracellular matrix|negative regulation of BMP signaling pathway|
extracellular matrix structural constituent|extracellular matrix binding|fibroblast proliferation|
collagen biosynthetic process|collagen trimer|fibrillar collagen trimer|inflammatory response to antigenic stimulus|
chemokine activity|chemokine production|cell chemotaxis|chemoattractant activity|
NLRP3 inflammasome complex assembly|inflammatory response to wounding|Wnt signaling pathway|response to oxidative stress|
regulation of vascular associated smooth muscle cell proliferation|
venous blood vessel development|regulation of developmental growth|lung alveolus development|myofibril assembly|
blood vessel diameter maintenance|
gas transport|cell maturation|regionalization|oxygen carrier activity|oxygen binding|
vascular associated smooth muscle cell proliferation",Description)) %>%
# group_by(Description) %>%
add_count() %>%
dplyr::arrange(dplyr::desc(n),dplyr::desc(Description)) %>%
mutate(Description =forcats:: fct_inorder(Description))
, #fibri|matrix|colla
aes(Cluster, y = Description)) + #stringr:: str_wrap
geom_point(aes(fill=p.adjust, size=Count), shape=21)+
theme_bw()+
theme(axis.text.x=element_text(angle=90,hjust = 1,vjust=0.5),
axis.text.y=element_text(size = 12),
axis.text = element_text(color = 'black', size = 12)
)+
scale_fill_gradient(low="red",high="blue")+
labs(x=NULL,y=NULL)
# coord_flip()
print(getwd())
p=ggplot( distinct(enrichmets,Description,.keep_all=TRUE) %>%
dplyr::mutate(Description = factor(Description, levels = unique(.$Description))) %>% #调整terms显示顺序
dplyr::filter(stringr::str_detect(pattern = "cilium organization|motile cilium|cilium movemen|cilium assembly|
cell-matrix adhesion|extracellular matrix organization|regulation of acute inflammatory response to antigenic stimulus|
collagen-containing extracellular matrix|negative regulation of BMP signaling pathway|
extracellular matrix structural constituent|extracellular matrix binding|fibroblast proliferation|
collagen biosynthetic process|collagen trimer|fibrillar collagen trimer|inflammatory response to antigenic stimulus|
chemokine activity|chemokine production|cell chemotaxis|chemoattractant activity|
NLRP3 inflammasome complex assembly|inflammatory response to wounding|Wnt signaling pathway|response to oxidative stress|
regulation of vascular associated smooth muscle cell proliferation|
venous blood vessel development|regulation of developmental growth|lung alveolus development|myofibril assembly|
blood vessel diameter maintenance|
gas transport|cell maturation|regionalization|oxygen carrier activity|oxygen binding|
vascular associated smooth muscle cell proliferation",Description)) %>%
group_by(Description) %>%
add_count() %>%
dplyr::arrange(dplyr::desc(n),dplyr::desc(Description)) %>%
mutate(Description =forcats:: fct_inorder(Description))
, #fibri|matrix|colla
aes(Cluster, y = Description)) + #stringr:: str_wrap
#scale_y_discrete(labels = function(x) stringr::str_wrap(x, width = 60)) + #调整terms长度
geom_point(aes(fill=p.adjust, size=Count), shape=21)+
theme_bw()+
theme(axis.text.x=element_text(angle=90,hjust = 1,vjust=0.5),
axis.text.y=element_text(size = 12),
axis.text = element_text(color = 'black', size = 12)
)+
scale_fill_gradient(low="red",high="blue")+
labs(x=NULL,y=NULL)
# coord_flip()
print(getwd())
ggsave(filename ="~/silicosis/spatial/sp_cluster_rigions_after_harmony/enrichents12.pdf",plot = p,
width = 10,height = 12,limitsize = FALSE)
######展示term内所有基因,用热图展示-------
#提取画图的数据
p$data
#提取图形中的所有基因-----
mygenes= p$data $geneID %>% stringr::str_split(.,"/",simplify = TRUE) %>%as.vector() %>%unique()
frame_for_genes=p$data %>%as.data.frame() %>% dplyr::group_by(Cluster) #后面使用split的话,必须按照分组排序
head(frame_for_genes)
my_genelist= split(frame_for_genes, frame_for_genes$Cluster, drop = TRUE) %>% #注意drop参数的理解
lapply(function(x) select(x, geneID));my_genelist
my_genelist= split(frame_for_genes, frame_for_genes$Cluster, drop = TRUE) %>% #注意drop参数的理解
lapply(function(x) x$geneID);my_genelist
mygenes=my_genelist %>% lapply( function(x) {stringr::str_split(x,"/",simplify = TRUE) %>%as.vector() %>%unique()} )
#准备画热图,加载seurat对象
load("/home/data/t040413/silicosis/spatial_transcriptomics/silicosis_ST_harmony_SCT_r0.5.rds")
{dim(d.all)
DefaultAssay(d.all)="Spatial"
#visium_slides=SplitObject(object = d.all,split.by = "stim")
names(d.all);dim(d.all)
d.all@meta.data %>%head()
head(colnames(d.all))
#1 给d.all 添加meta信息------
adata_obs=read.csv("~/silicosis/spatial/adata_obs.csv")
head(adata_obs)
mymeta= paste0(d.all@meta.data$orig.ident,"_",colnames(d.all)) %>% gsub("-.*","",.) # %>% head()
head(mymeta)
tail(mymeta)
#掉-及其之后内容
adata_obs$col= adata_obs$spot_id %>% gsub("-.*","",.) # %>% head()
head(adata_obs)
rownames(adata_obs)=adata_obs$col
adata_obs=adata_obs[mymeta,]
head(adata_obs)
identical(mymeta,adata_obs$col)
d.all=AddMetaData(d.all,metadata = adata_obs)
head(d.all@meta.data)}
##构建画热图对象---
Idents(d.all)=d.all$clusters
a=AverageExpression(d.all,return.seurat = TRUE)
a$orig.ident=rownames(a@meta.data)
head(a@meta.data)
head(markers)
rownames(a) %>%head()
head(mygenes)
table(mygenes %in% rownames(a))
DoHeatmap(a,draw.lines = FALSE, slot = 'scale.data', group.by = 'orig.ident',
features = mygenes ) +
ggplot2:: scale_color_discrete(name = "Identity", labels = unique(a$orig.ident) %>%sort() )
##doheatmap做出来的图不好调整,换成heatmap自己调整
p=DoHeatmap(a,draw.lines = FALSE, slot = 'scale.data', group.by = 'orig.ident',
features = mygenes ) +
ggplot2:: scale_color_discrete( labels = unique(a$orig.ident) %>%sort() ) #name = "Identity",
p$data %>%head()
##########这种方式容易出现bug,不建议------
if (F) {
wide_data <- p$data %>% .[,-4] %>%
tidyr:: pivot_wider(names_from = Cell, values_from = Expression)
print(wide_data)
mydata= wide_data %>%
dplyr:: select(-Feature) %>%
as.matrix()
head(mydata)
rownames(mydata)=wide_data$Feature
mydata=mydata[,c("Bronchial zone", "Fibrogenic zone", "Interstitial zone", "Inflammatory zone","Vascular zone" )]
p2=pheatmap:: pheatmap(mydata, fontsize_row = 2,
clustering_method = "ward.D2",
# annotation_col = wide_data$Feature,
annotation_colors = c("Interstitial zone" = "red", "Bronchial zone" = "blue", "Fibrogenic zone" = "green", "Vascular zone" = "purple") ,
cluster_cols = FALSE,
column_order = c("Inflammatory zone", "Vascular zone" ,"Bronchial zone", "Fibrogenic zone" )
)
getwd()
ggplot2::ggsave(filename = "~/silicosis/spatial/sp_cluster_rigions_after_harmony/heatmap_usingpheatmap.pdf",width = 8,height = 10,limitsize = FALSE,plot = p2)
}
##########建议如下方式画热图------
a$orig.ident=a@meta.data %>%rownames()
a@meta.data %>%head()
Idents(a)=a$orig.ident
a@assays$Spatial@scale.data %>%head()
mydata=a@assays$Spatial@scale.data
mydata=mydata[rownames(mydata) %in% (mygenes %>%unlist() %>%unique()) ,]
mydata= mydata[,c( "Fibrogenic zone", "Inflammatory zone", "Bronchial zone","Interstitial zone","Vascular zone" )]
head(mydata)
p3=pheatmap:: pheatmap(mydata, fontsize_row = 2,
clustering_method = "ward.D2",
# annotation_col = wide_data$Feature,
annotation_colors = c("Interstitial zone" = "red", "Bronchial zone" = "blue", "Fibrogenic zone" = "green", "Vascular zone" = "purple") ,
cluster_cols = FALSE,
column_order = c("Inflammatory zone", "Vascular zone" ,"Bronchial zone", "Fibrogenic zone" )
)
getwd()
ggplot2::ggsave(filename = "~/silicosis/spatial/sp_cluster_rigions_after_harmony/heatmap_usingpheatmap2.pdf",width = 8,height = 10,limitsize = FALSE,plot = p3)
#########单独画出炎症区和纤维化区---------
a@assays$Spatial@scale.data %>%head()
mydata=a@assays$Spatial@scale.data
mygenes2= my_genelist[c('Inflammatory zone','Fibrogenic zone')] %>% unlist() %>% stringr::str_split("/",simplify = TRUE)
mydata2=mydata[rownames(mydata) %in% ( mygenes2 %>%unlist() %>%unique()) ,]
mydata2= mydata2[,c( "Fibrogenic zone", "Inflammatory zone" )]
head(mydata2)
p3=pheatmap:: pheatmap(mydata2, fontsize_row = 5, #scale = 'row',
clustering_method = "ward.D2",
# annotation_col = wide_data$Feature,
annotation_colors = c("Interstitial zone" = "red", "Bronchial zone" = "blue", "Fibrogenic zone" = "green", "Vascular zone" = "purple") ,
cluster_cols = FALSE,
column_order = c("Inflammatory zone", "Vascular zone" ,"Bronchial zone", "Fibrogenic zone" )
)
getwd()
ggplot2::ggsave(filename = "~/silicosis/spatial/sp_cluster_rigions_after_harmony/heatmap_usingpheatmap3.pdf",width = 4,height = 8,limitsize = FALSE,plot = p3)
.libPaths(c("/home/data/t040413/R/x86_64-pc-linux-gnu-library/4.2",
"/home/data/t040413/R/yll/usr/local/lib/R/site-library",
"/usr/local/lib/R/library",
"/refdir/Rlib/"))
library(Seurat)
library(ggplot2)
library(dplyr)
load('/home/data/t040413/silicosis/fibroblast_myofibroblast2/subset_data_fibroblast_myofibroblast2.rds')
print(getwd())
dir.create("~/silicosis/fibroblast_myofibroblast2/slilica_fibroblast_enrichments")
setwd("~/silicosis/fibroblast_myofibroblast2/slilica_fibroblast_enrichments")
getwd()
DimPlot(subset_data,label = TRUE)
markers_foreach=FindAllMarkers(subset_data,only.pos = TRUE,densify = TRUE)
head(markers_foreach)
all_degs=markers_foreach
markers=markers_foreach
#install.packages("AnnotationDbi")
#BiocManager::install(version = '3.18')
#BiocManager::install('AnnotationDbi',version = '3.18')
{
##########################---------------------批量富集分析findallmarkers-enrichment analysis==================================================
#https://mp.weixin.qq.com/s/WyT-7yKB9YKkZjjyraZdPg
df=all_degs
##筛选阈值确定:p<0.05,|log2FC|>1
p_val_adj = 0.05
# avg_log2FC = 0.1
fc=seq(0.8,1.4,0.2)
print(getwd())
#setwd("../")
for (avg_log2FC in fc) {
# avg_log2FC=0.5
dir.create(paste0(avg_log2FC,"/"))
setwd(paste0(avg_log2FC,"/"))
print(getwd())
print(paste0("Start----",avg_log2FC))
head(all_degs)
#根据阈值添加上下调分组标签:
df$direction <- case_when(
df$avg_log2FC > avg_log2FC & df$p_val_adj < p_val_adj ~ "up",
df$avg_log2FC < -avg_log2FC & df$p_val_adj < p_val_adj ~ "down",
TRUE ~ 'none'
)
head(df)
df=df[df$direction!="none",]
head(df)
dim(df)
df$mygroup=paste(df$group,df$direction,sep = "_")
head(df)
dim(df)
##########################----------------------enrichment analysis=
#https://mp.weixin.qq.com/s/WyT-7yKB9YKkZjjyraZdPg
{
library(clusterProfiler)
library(org.Hs.eg.db) #人
library(org.Mm.eg.db) #鼠
library(ggplot2)
# degs_for_nlung_vs_tlung$gene=rownames(degs_for_nlung_vs_tlung)
head(markers)
df=markers %>%dplyr::group_by(cluster)%>%
filter(p_val_adj <0.05
)
sce.markers=df
head(sce.markers)
print(getwd())
ids <- suppressWarnings(bitr(sce.markers$gene, 'SYMBOL', 'ENTREZID', 'org.Mm.eg.db'))
head(ids)
head(sce.markers)
tail(sce.markers)
dim(sce.markers)
sce.markers=merge(sce.markers,ids,by.x='gene',by.y='SYMBOL')
head(sce.markers)
dim(sce.markers)
sce.markers$group=sce.markers$cluster
sce.markers=sce.markers[sce.markers$group!="none",]
dim(sce.markers)
head(sce.markers)
getwd()
# sce.markers=openxlsx::read.xlsx("/home/data/t040413/silicosis/fibroblast_myofibroblast/group_enrichments/")
#sce.markers$cluster=sce.markers$mygroup
dim(sce.markers)
head(sce.markers)
gcSample=split(sce.markers$ENTREZID, sce.markers$cluster)
library(clusterProfiler)
gcSample # entrez id , compareCluster
print("===========开始go= All ont===========")
xx <- compareCluster(gcSample, fun="enrichGO",OrgDb="org.Mm.eg.db" , #'org.Hs.eg.db',
pvalueCutoff=0.05) #organism="hsa",
xx.BP <- compareCluster(gcSample, fun="enrichGO",OrgDb="org.Mm.eg.db" , #'org.Hs.eg.db',
pvalueCutoff=0.05,readable=TRUE,
ont="BP") #organism="hsa",
p=clusterProfiler::dotplot(object = xx.BP,showCategory = 20,
label_format =60)
p=p+ theme(axis.text.x = element_text(angle = 90,
vjust = 0.5, hjust=0.5))
p
ggsave(paste0(avg_log2FC,'_degs_compareCluster-BP_enrichment--3.pdf'),plot = p,width = 13,height = 40,limitsize = F)
ggsave(paste0(avg_log2FC,'_degs_compareCluster-BP_enrichment--3.pdf'),plot = p,width = 13,height = 40,limitsize = F)
xx.CC <- compareCluster(gcSample, fun="enrichGO",OrgDb="org.Mm.eg.db" , #'org.Hs.eg.db',
pvalueCutoff=0.05,readable=TRUE,
ont="CC") #organism="hsa",
p=clusterProfiler::dotplot(object = xx.CC,showCategory = 20,
label_format =60)
p=p+ theme(axis.text.x = element_text(angle = 90,
vjust = 0.5, hjust=0.5))
p
ggsave(paste0(avg_log2FC,'_degs_compareCluster-CC_enrichment--3.pdf'),plot = p,width = 13,height = 40,limitsize = F)
ggsave(paste0(avg_log2FC,'_degs_compareCluster-CC_enrichment--3.pdf'),plot = p,width = 13,height = 40,limitsize = F)
xx.MF <- compareCluster(gcSample, fun="enrichGO",OrgDb="org.Mm.eg.db" , #'org.Hs.eg.db',
pvalueCutoff=0.05,readable=TRUE,
ont="MF") #organism="hsa",
p=clusterProfiler::dotplot(object = xx.MF,showCategory = 20,
label_format =60)
p=p+ theme(axis.text.x = element_text(angle = 90,
vjust = 0.5, hjust=0.5))
p
ggsave(paste0(avg_log2FC,'_degs_compareCluster-MF_enrichment--3.pdf'),plot = p,width = 13,height = 40,limitsize = F)
ggsave(paste0(avg_log2FC,'_degs_compareCluster-MF_enrichment--3.pdf'),plot = p,width = 13,height = 40,limitsize = F)
print(getwd())
.libPaths()
print("===========开始 kegg All ont============")
gg<-clusterProfiler::compareCluster(gcSample,fun = "enrichKEGG", #readable=TRUE,
keyType = 'kegg', #KEGG 富集
organism='mmu',#"rno",
pvalueCutoff = 0.05 #指定 p 值阈值(可指定 1 以输出全部
)
p=clusterProfiler::dotplot(object = xx,showCategory = 20,
label_format =100)
p=p+ theme(axis.text.x = element_text(angle = 90,
vjust = 0.5, hjust=0.5))
p
ggsave(paste0(avg_log2FC,'_degs_compareCluster-GO_enrichment--3.pdf'),plot = p,width = 13,height = 40,limitsize = F)
ggsave(paste0(avg_log2FC,'_degs_compareCluster-GO_enrichment--3.pdf'),plot = p,width = 13,height = 40,limitsize = F)
xx
write.csv(xx,file = paste0(avg_log2FC,"compareCluster-GO_enrichment.csv"))
p=clusterProfiler::dotplot(gg,showCategory = 20,
label_format = 40)
p4=p+ theme(axis.text.x = element_text(angle = 90,
vjust = 0.5, hjust=0.5))
p4
print(paste("保存位置",getwd(),sep = " : "))
ggsave(paste0(avg_log2FC,'_degs_compareCluster-KEGG_enrichment-2.pdf'),plot = p4,width = 13,height = 25,limitsize = F)
ggsave(paste0(avg_log2FC,'_degs_compareCluster-KEGG_enrichment-2.pdf'),plot = p4,width = 13,height = 25,limitsize = F)
gg
openxlsx::write.xlsx(gg,file = paste0(avg_log2FC,"_compareCluster-KEGG_enrichment.xlsx"))
getwd()
openxlsx::write.xlsx(sce.markers,file = paste0(avg_log2FC,"_sce.markers_for_each_clusterfor_enrichment.xlsx"))
##放大图片
{
getwd()
#bp
p=clusterProfiler::dotplot(object = xx.BP,showCategory = 100,
label_format =60)
p=p+ theme(axis.text.x = element_text(angle = 90,
vjust = 0.5, hjust=0.5))
p
ggsave(paste0(avg_log2FC,'_degs_compareCluster-BP_enrichment-100terms-3.pdf'),plot = p,width = 13,height = 100,limitsize = F)
ggsave(paste0(avg_log2FC,'_degs_compareCluster-BP_enrichment-100terms-3.pdf'),plot = p,width = 13,height = 100,limitsize = F)
print((getwd()))
#cc
p=clusterProfiler::dotplot(object = xx.CC,showCategory = 100,
label_format =60)
p=p+ theme(axis.text.x = element_text(angle = 90,
vjust = 0.5, hjust=0.5))
p
ggsave(paste0(avg_log2FC,'_degs_compareCluster-CC_enrichment-100terms-3.pdf'),plot = p,width = 13,height = 100,limitsize = F)
ggsave(paste0(avg_log2FC,'_degs_compareCluster-CC_enrichment-100terms-3.pdf'),plot = p,width = 13,height = 100,limitsize = F)
print((getwd()))
#MF
p=clusterProfiler::dotplot(object = xx.MF,showCategory = 100,
label_format =60)
p=p+ theme(axis.text.x = element_text(angle = 90,
vjust = 0.5, hjust=0.5))
p
ggsave(paste0(avg_log2FC,'_degs_compareCluster-MF_enrichment-100terms-3.pdf'),plot = p,width = 13,height = 100,limitsize = F)
ggsave(paste0(avg_log2FC,'_degs_compareCluster-MF_enrichment-100terms-3.pdf'),plot = p,width = 13,height = 100,limitsize = F)
print((getwd()))
#KEGG
p=clusterProfiler::dotplot(object = gg,showCategory = 100,
label_format =60)
p=p+ theme(axis.text.x = element_text(angle = 90,
vjust = 0.5, hjust=0.5))
p
ggsave(paste0(avg_log2FC,'_degs_compareCluster-KEGG_enrichment-100terms-3.pdf'),plot = p,width = 13,height = 100,limitsize = F)
ggsave(paste0(avg_log2FC,'_degs_compareCluster-KEGG_enrichment-100terms-3.pdf'),plot = p,width = 13,height = 100,limitsize = F)
print((getwd()))
}
# save(xx.BP,xx.CC,xx.MF, gg, file = "~/silicosis/spatial_transcriptomicsharmony_cluster_0.5res_gsea/xx.Rdata")
xx.BP@compareClusterResult$oncology="BP"
xx.CC@compareClusterResult$oncology="CC"
xx.MF@compareClusterResult$oncology="MF"
xx.all=do.call(rbind,list(xx.BP@compareClusterResult,
xx.CC@compareClusterResult,
xx.MF@compareClusterResult))
head(xx.all)
openxlsx::write.xlsx(xx.all,file = paste0(avg_log2FC,"_enrichments_all.xlsx"))
# save(xx.BP,xx.CC,xx.MF,xx.all,sce.markers,merged_degs, gg, file = "./xx.Rdata")
result <- tryCatch({
save(xx.BP,xx.CC,xx.MF,xx.all,sce.markers,merged_degs, gg, file = paste0(avg_log2FC,"_xx.Rdata"))
# 在这里添加你希望在没有报错时执行的代码
print("没有报错")
}, error = function(e) {
print(getwd()) # 在报错时执行的代码
# 在这里添加你希望在报错时执行的额外代码
save(xx.BP,xx.CC,xx.MF,xx.all,sce.markers, gg, file = paste0(avg_log2FC,"_xx_all.Rdata"))
})
# print(result)
print(getwd())
if (F) {
#大鼠
print("===========开始go============")
xx <-clusterProfiler::compareCluster(gcSample, fun="enrichGO",OrgDb="org.Rn.eg.db",
readable=TRUE,
ont = 'ALL', #GO Ontology,可选 BP、MF、CC,也可以指定 ALL 同时计算 3 者
pvalueCutoff=0.05) #organism="hsa", #'org.Hs.eg.db',
print("===========开始 kegg============")
gg<-clusterProfiler::compareCluster(gcSample,fun = "enrichKEGG",
keyType = 'kegg', #KEGG 富集
organism="rno",
pvalueCutoff = 0.05 #指定 p 值阈值(可指定 1 以输出全部
)
p=dotplot(xx)
p2=p+ theme(axis.text.x = element_text(angle = 90,
vjust = 0.5, hjust=0.5))
p2
ggsave('degs_compareCluster-GO_enrichment-2.pdf',plot = p2,width = 6,height = 20,limitsize = F)
xx
openxlsx::write.xlsx(xx,file = "compareCluster-GO_enrichment.xlsx")
#
p=dotplot(gg)
p4=p+ theme(axis.text.x = element_text(angle = 90,
vjust = 0.5, hjust=0.5))
p4
print(paste("保存位置",getwd(),sep = " : "))
ggsave('degs_compareCluster-KEGG_enrichment-2.pdf',plot = p4,width = 6,height = 12,limitsize = F)
gg
openxlsx::write.xlsx(gg,file = "compareCluster-KEGG_enrichment.xlsx")
}
setwd("../")
}
}
}
print(getwd())
#setwd('../')
load("~/silicosis/fibroblast_myofibroblast2/slilica_fibroblast_enrichments/1/1_xx_all.Rdata")
# remove duplicate rows based on Description 并且保留其他所有变量
xx.all<- distinct(xx.all, Description,.keep_all = TRUE)
enrichmets=xx.all
#2.2挑选term---
selected_clusterenrich=enrichmets[grepl(pattern = "lung alveolus developmen|extracellular matrix organization|extracellular structure organization|response to toxic substance|blood vessel endothelial cell migration
regulation of blood vessel endothelial cell migration|lung development|respiratory tube development|detoxification|
cellular response to toxic substance|endothelial cell proliferation|cellular response to chemical stress|
Wnt|infla|fibrob|myeloid",
x = enrichmets$Description) &
stringr::str_detect(pattern = "muscle|fibroblast migration|positive regulation of endothelial cell proliferation|
inflammatory response to wounding|wound healing involved in inflammatory response|fibronectin binding|fibronectin binding|inflammatory response to wounding|fibronectin binding", negate = TRUE, string = enrichmets$Description)
,]
head(selected_clusterenrich)
distinct(selected_clusterenrich)
# remove duplicate rows based on Description 并且保留其他所有变量
distinct_df <- distinct(selected_clusterenrich, Description,.keep_all = TRUE)
ggplot( distinct(selected_clusterenrich,Description,.keep_all=TRUE) %>%
# dplyr::mutate(Cluster = factor(Cluster, levels = unique(.$Cluster))) %>%
dplyr::mutate(Description = factor(Description, levels = unique(.$Description))) %>%
# dplyr::group_by(Cluster) %>%
dplyr::filter(stringr::str_detect(pattern = "muscle", negate = TRUE,Description)) %>%
add_count() %>%
dplyr::arrange(dplyr::desc(n),dplyr::desc(Description)) %>%
mutate(Description =forcats:: fct_inorder(Description))
, #fibri|matrix|colla
aes(Cluster, y = Description)) + #stringr:: str_wrap
scale_y_discrete(labels = function(x) stringr::str_wrap(x, width = 58)) + #调整terms长度 字符太长
geom_point(aes(fill=p.adjust, size=Count), shape=21)+
theme_bw()+
theme(axis.text.x=element_text(angle=90,hjust = 1,vjust=0.5),
axis.text.y=element_text(size = 12),
axis.text = element_text(color = 'black', size = 12)
)+
scale_fill_gradient(low="red",high="blue")+
labs(x=NULL,y=NULL)
# coord_flip()
print(getwd())