cpp的例子
device_malloc
- cpp没有用具体数值初始化 float *d_from_tensor = NULL;device_malloc(&d_from_tensor, batch_size * seq_len * hidden_dim);
- https://github1s.com/NVIDIA/FasterTransformer/blob/v1.0/sample/cpp/transformer_fp32.cc#L35-L38 直接用的cudaMalloc
void device_malloc(float** ptr, int size) // cudaMalloc函数为什么是二级指针的解释https://blog.csdn.net/CaiYuxingzzz/article/details/121112273
{
cudaMalloc((void**)ptr, sizeof(float) * size);
}
allocator
- allocator用于分配attr_out_buf_
https://github1s.com/NVIDIA/FasterTransformer/blob/v1.0/fastertransformer/bert_encoder_transformer.h#L131-L135
buf_ = reinterpret_cast<DataType_*>(allocator_.malloc(sizeof(DataType_) * buf_size * 6));
- 然后将这些参数和encoder_param打包成multi_head_init_param
在初始化(encoder_transformer_->initialize)时传给attention_->initialize(multi_head_init_param);
attention_->initialize则只需将传入的参数初始化给attention对象的参数,等forward时调用自己的参数
接口包含两个方法malloc,free
class IAllocator{
public:
virtual void* malloc(size_t size) const = 0;
virtual void free(void* ptr) const = 0;
};
//AllocatorTypeyouenum class AllocatorType{CUDA, TF}; 用的应该是CUDA的
template<>
class Allocator<AllocatorType::CUDA> : public IAllocator{
const int device_id_;
public:
Allocator(int device_id): device_id_(device_id){}
void* malloc(size_t size) const {
void* ptr = nullptr;
int o_device = 0;
check_cuda_error(get_set_device(device_id_, &o_device));
check_cuda_error(cudaMalloc(&ptr, size));
check_cuda_error(get_set_device(o_device));
return ptr;
}
void free(void* ptr) const {
int o_device = 0;
check_cuda_error(get_set_device(device_id_, &o_device));
check_cuda_error(cudaFree(ptr));
check_cuda_error(get_set_device(o_device));
return;
}
};
fastertransformer::Allocator<AllocatorType::CUDA> allocator(0); // 0是device_id_
encoder_param
- EncoderInitParam encoder_param; //init param here 包含参数的结构体,成员记录了GPU数据的地址
initialize
BertEncoderTransformer<EncoderTraits_> *encoder_transformer_ = new
BertEncoderTransformer<EncoderTraits_>(allocator, batch_size, from_seq_len, to_seq_len, head_num, size_per_head);
encoder_transformer_->initialize(encoder_param);
trt_plugin的例子
将数值放入vector
- https://github1s.com/NVIDIA/FasterTransformer/blob/v1.0/sample/tensorRT/transformer_trt.cc#L108-L136
- 先分配地址
host_malloc(&h_attr_kernel_Q, hidden_dim * hidden_dim); - 然后进行赋值
h_attr_kernel_Q[i] = 0.001f;
std::vector<T* > layer_param;
layer_param.push_back(h_attr_kernel_Q);
将值打包
params.push_back(layer_param);
}
cudaStream_t stream;
cudaStreamCreate(&stream);
TRT_Transformer<T>* trt_transformer = new TRT_Transformer<T>(batch_size, seq_len, head_num, hidden_dim, layers);
trt_transformer->build_engine(params);
trt_transformer->do_inference(batch_size, h_from_tensor, h_attr_mask, h_transformer_out, stream);
delete trt_transformer;
- 构建TRT_Transformer时会调用算子插件,权重在void build_engine(std::vector<std::vector<T* > > &weights)时传入
https://github1s.com/NVIDIA/FasterTransformer/blob/v1.0/fastertransformer/trt_plugin/trt_model.h#L75-L77
auto plugin = new TransformerPlugin<T>(
hidden_dim_, head_num_, seq_len_, batch_size_,
point2weight(weights[i][0], hidden_dim_ * hidden_dim_),
- 创建TransformerPlugin实例时会传入权重
TransformerPlugin(
int hidden_dim, int head_num, int seq_len, int max_batch_size,
const nvinfer1::Weights &w_attr_kernel_Q,...
- 这里就是和cpp例子的不同了,其使用权重w_attr_kernel_Q
- https://github1s.com/NVIDIA/FasterTransformer/blob/v1.0/fastertransformer/trt_plugin/bert_transformer_plugin.h#L103
cudaMallocAndCopy(d_attr_kernel_Q_, w_attr_kernel_Q, hidden_dim * hidden_dim);
- cudaMallocAndCopy定义在https://github1s.com/NVIDIA/FasterTransformer/blob/v1.0/fastertransformer/trt_plugin/bert_transformer_plugin.h#L338-L352
static void cudaMallocAndCopy(T *&dpWeight, const nvinfer1::Weights &w, int nValue)
{
assert(w.count == nValue);
check_cuda_error(cudaMalloc(&dpWeight, nValue * sizeof(T)));
check_cuda_error(cudaMemcpy(dpWeight, w.values, nValue * sizeof(T), cudaMemcpyHostToDevice));
T* data = (T*)malloc(sizeof(T) * nValue);
cudaMemcpy(data, dpWeight, sizeof(T) * nValue, cudaMemcpyDeviceToHost);
}
static void cudaMallocAndCopy(T*&dpWeight, const T *&dpWeightOld, int nValue)
{
check_cuda_error(cudaMalloc(&dpWeight, nValue * sizeof(T)));
check_cuda_error(cudaMemcpy(dpWeight, dpWeightOld, nValue * sizeof(T), cudaMemcpyDeviceToDevice));
}
cg
- https://github.com/NVIDIA/TensorRT/blob/release/8.5/demo/Diffusion/models.py
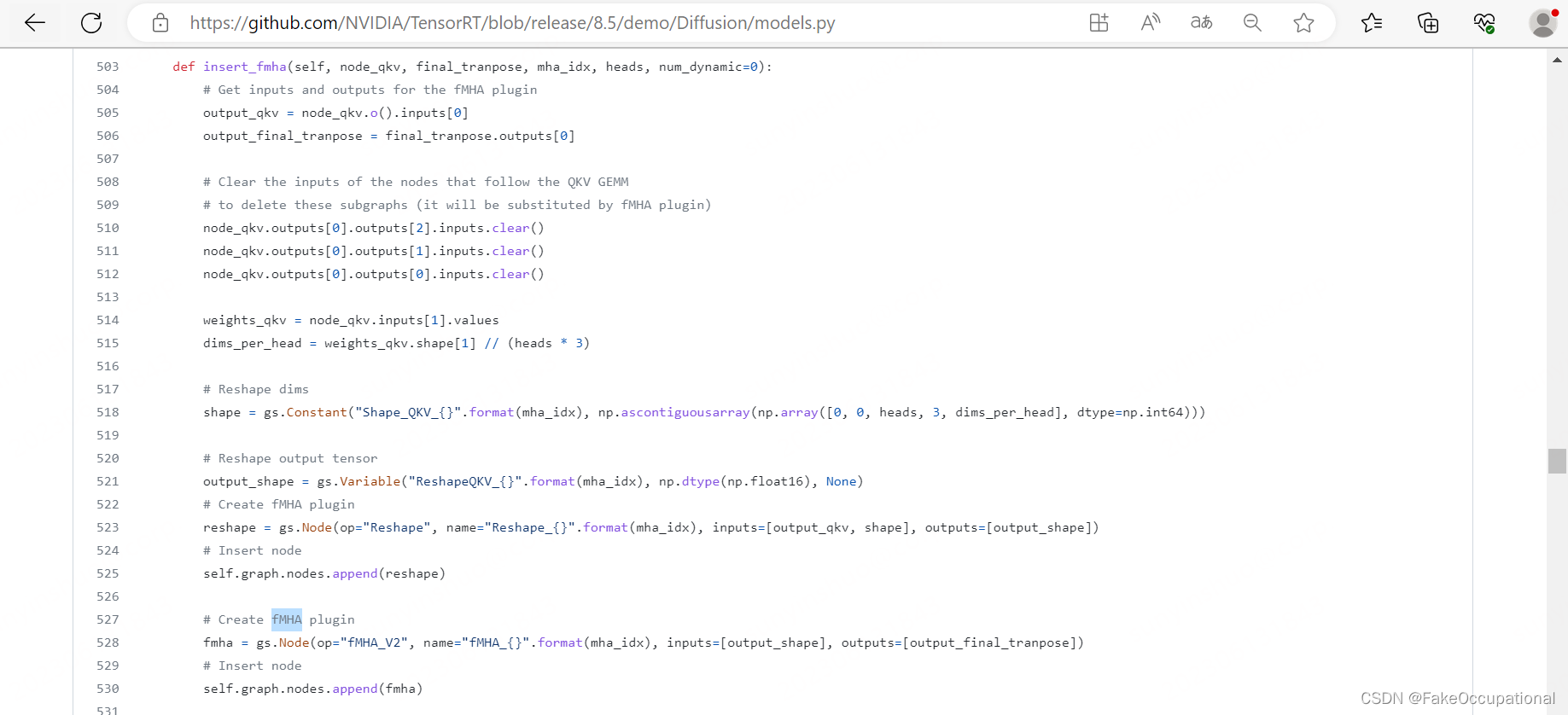