2.3、Spark Streaming
2.3.0、Overview
Spark Streaming 是核心 Spark API 的扩展,它支持实时数据流的可扩展、高吞吐量、容错流处理。数据可以从许多来源(如 Kafka、Kinesis 或 TCP 套接字)获取,并且可以使用复杂的算法进行处理,这些算法由 map、reduce、join 和 window 等高级函数表示。最后,可以将处理后的数据推送到文件系统、数据库和实时仪表板。当然也可以在数据流上应用机器学习和图处理。
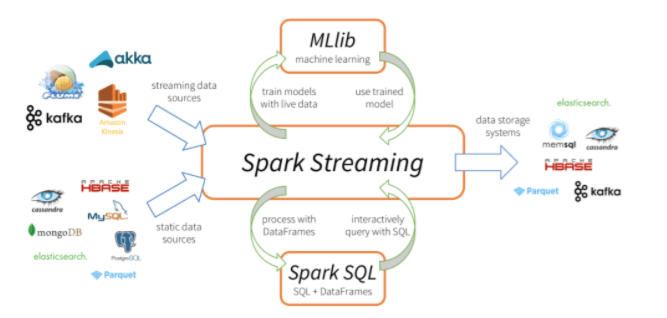
工作原理如下:Spark Streaming 接收实时输入的数据流,并将数据分成批处理,然后由 Spark 引擎处理以批处理生成最终的结果流。其中SparkStreaming提供了一种离散流或DStream的高级抽象来代表一个连续的数据流,底层就是由一系列RDD来表示。

DStream 中的每个 RDD 都包含来自某个区间的数据,如下图:
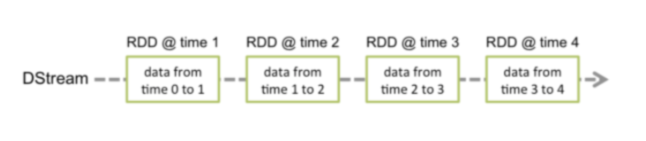
2.3.0.1、Example
import org.apache.spark._
import org.apache .spark.streaming._
import org.apache.spark.streaming.StreamingContext_ // not necessary since Spark 1.3
// Create a local StreamingContext with two working thread and batch interval of 1 second.
// The master requires 2 cores to prevent a starvation scenario.
val conf = new SparkConf().setMaster("local[2]").setAppName("NetworkWordCount")
val ssc = new streamingContext(conf, Seconds(1))
// Create a Dstream that will connect to hostname:port, like localhost:9999
val lines = ssc.socketTextstream("localhost", 9999)
// Split each line into words
val words = lines.flatMap(_.split(”"))
// Count each word in each batch
val pairs = words.map(word => (word, 1))
val wordCounts = pairs.reduceByKey(_ + _)
// Print the first ten elements of each RDD generated in this Dstream to the console
wordCounts.print()
ssc.start() // start the computation
ssc.awaitTermination() // Wait for the computation to terminate
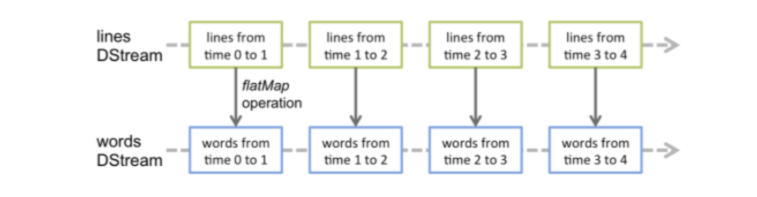
如上面的demo所示,每个输入流都会和一个Receiver对象相关联,该对象用来接收数据并将其存储在Spark内存中进行下一步的处理。因此如果你想要在流应用程序中并行接收多个数据流的话,那么就得需要创建多个Receiver对象用来接收数据。同时也需要记住的是SparkStreaming应用程序是属于常驻的,而且也是Spark程序,那么Worker/Executor也会占用一部分资源,所以为了能够保障运行Receiver以及正常处理数据,那么就需要申请到足够的资源,所以其分配的核数一定要大于receivers的个数。
2.3.0.2、Points To Remember
1、一旦Context启动之后,就不能增加或者设置新的流计算
2、一旦Context停止后,就无法重新启动。这里说的是容错方面。
3、同一时间一个JVM内只能有一个StreamingContext。
4、在StreamingContext上调用stop()方法,同时也会把SparkContext给停止;如果只是想停止StreamingContext,那么可以在调用stop()方法的时候指定stopSparkContext=false。
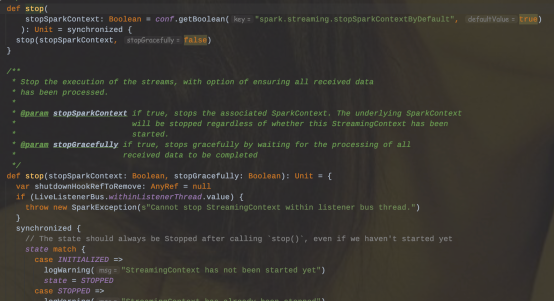
5、一个SparkContext可以被复用创建多个StreamingContext(即在下一个StreamingContext被创建之前停止上一个StreamingContext,且不停止SparkContext)
2.3.1、Receiver
SparkStreaming可以从任意的数据源来接收数据并处理,目前内置的数据源包括Kafka、File、Socket等等。当然目前Spark内置支持的数据源可以满足日常大部分的场景,但有些时候仍然需要自定义Receiver来定制接收数据源。这小节将来讲述如何实现一个自定义的Receiver。首先要继承Receiver,然后重写onStart和onStop方法。onStart()方法会在启动的时候负责接收数据;onStop()方法将停止这些接收数据的线程,当然还可以使用isStopped()方法来检查它们是否停止接收数据。
在 Spark Streaming 中,当一个 Receiver 启动时,每隔 spark.streaming.blockInterval 毫秒就会产生一个新的块,每个块都会变成 RDD 的一个分区,最终由 DStream 创建。例如,由 KafkaInputDStream 创建的 RDD 中的分区数由 batchInterval / spark.streaming.blockInterval 确定,其中 batchInterval 是将流数据分成批次的时间间隔(通过 StreamingContext 的构造函数参数设置)。例如,如果批处理间隔为 2 秒(默认),块间隔为 200 毫秒(默认),则RDD 将包含 10 个分区,还有一个流程路径涉及从迭代器接收数据,由 ReceivedBlockHandler 表示。创建 RDD 后,驱动程序的 JobScheduler 可以将其处理安排为作业。在 Spark Streaming 的当前实现和默认配置下,任何时间点只有一个作业处于活动状态(即正在执行)。因此,如果一个批次的处理时间比批次间隔长,那么下一个批次的作业将保持排队,将其设置为 1 的原因是并发作业可能会导致奇怪的资源共享,并且可能难以调试系统中是否有足够的资源来足够快地处理摄取的数据,当然可以通过实验性 Spark 属性 spark.streaming.concurrentJobs 进行更改,默认情况下设置为 1。一次只运行一个作业,不难看出,如果批处理时间小于批处理间隔,那么系统将是稳定的。
Receiver一旦接收到数据后,那么就会调用store(data)方法进行存储,这里有两种处理方式来保障Receiver是否可靠:
1、来一条存储一条,这种可靠性较差
2、存储整个对象/序列化集合。(阻塞的方式存储)
其自定义实现store()方法会影响到整体的容错和可靠。当应用程序发生了异常时应该要有捕获机制,并要有重试机制。
如果应用程序发生重启的时候,那么会调用Receiver类中的restart()方法,其内部会异步调用onStop方法并隔一定延迟后调用onStart()方法完成重启动作。
public class JavaCustomReceiver extends Receiver<String> {
String host = null;
int port = -1;
public JavaCustomReceiver(String host_ , int port_) {
super(storageLevel.MEMORY_AND_DISK_2());
host = host_;
port = port_;
}
@Override
public void onstart() {
// Start the thread that receives data over a connection
new Thread(this::receive).start();
}
@override
public void onstop() {
// There is nothing much to do as the thread calling receive()
// is designed to stop by itself if isStopped() returns false
}
/** Create a socket connection and receive data until receiver is stopped */
private void receive() {
Socket socket = nul1;
String userInput = null;
try {
// connect to the server
socket = new Socket(host, port);
BufferedReader reader = new BufferedReader(
new InputstreamReader(socket.getInputstream(), StandardCharsets.UTF 8))
// Until stopped or connection broken continue reading
while (!isStopped() && (userInput = reader.readLine()) != null) {
System.out.println("Received data "" + userInput + "");
store(userInput);
}
reader.close();
socket.close();
// Restart in an attempt to connect again when server is active again
restart("Trying to connect again");
} catch(ConnectException ce) {
// restart if could not connect to server
restart("Could not connect", ce);
} catch(Throwable t) f
// restart if there is any other error
restart("Error receiving data", t);
}
}
}
// 调用自定义Receiver:
// Assuming ssc is the JavastreamingContext
JavaDStream<String> customReceiverstream = ssc.receiverstream(
new JavaCustomReceiver(host, port));
JavaDstream<String> words = customReceiverstream.flatMap(s -> ...);
...