摄影:Fauzan Saari on Unsplash
一、说明
这是我们对世界杯推特数据分析的第3部分,我们放弃了。我们将对我们的数据进行情绪分析,以了解人们对卡塔尔世界杯的感受。我将在这里介绍的一个功能强大的工具包是Hugging Face,您可以在其中找到各种模型,任务,数据集,它还为刚开始学习机器学习的人提供课程。在这篇文章中,我们将使用一个情绪分析模型和拥抱面孔令牌来完成我们的任务。
二、情绪分析
情感分析是使用自然语言处理(NLP)来识别,提取和研究情感状态和主观信息。我们可以将这种技术应用于客户评论和调查响应,以对我对产品或服务的意见。
让我们看几个例子:
- 我喜欢今天的天气!标记: 正面
- 天气预报说明天会多云。标签: 中性
- 雨不会停。野餐计划被推迟了。无赖。。。标签: 负面
上面的例子清楚地显示了推文的极性,因为文本的结构很简单。以下是一些难以轻易发现情绪的挑战性案例。
- 我不喜欢下雨天。(否定)
- 我喜欢在刮风的时候跑步,但不会推荐给我的朋友。(有条件的积极情绪,难以分类)
- 一杯好咖啡真的需要时间,因为它让我等了30分钟才喝一口。(讽刺)
现在我们已经介绍了什么是情绪分析以及如何应用这种技术,让我们学习如何在我们的 Twitter 数据上实现这种方法。
遇见“推特-罗伯塔-基地-情绪-最新"
用于情绪分析的 Twitter-roBERTa-base 是一个 RoBERTa-base 模型,从 124 年 2018 月到 2021 年 <> 月在 ~<>M 条推文上训练,并使用 TweetEval 基准对情绪分析进行了微调。我不会深入探讨 RoBERTa-base 模型的细节,但简单地说,RoBERTa 从预训练过程中删除了下一句预测 (NSP) 任务,并引入了动态掩码,因此掩码标记在训练期间会发生变化。对于更详细的评论,我建议阅读Suleiman Khan的文章和Chandan Durgia的文章。
要启动此模型,我们可以使用由Hugging Face团队创建的推理API。推理 API 允许您对 NLP、音频和计算机视觉中的任务进行文本文本和评估 80, 000 多个机器学习模型。查看此处以获取详细文档。它很容易使用推理API,您只需这样做即可获得您的拥抱脸令牌(它是免费的)。首先,您应该创建一个拥抱脸帐户并注册。然后,单击您的个人资料并转到设置。
- 读取:如果您只需要从拥抱人脸中心读取内容(例如,在下载私有模型或进行推理时),请使用此角色。
- 写入:如果需要创建内容或将内容推送到存储库(例如,在训练模型或修改模型卡时),请使用此令牌。
由作者创建
User access tokens
现在我们有了我们需要的一切,让我们做一些分析!
三、准备我们的数据
首先,我们需要导入一些依赖项并加载数据。对我们拥有的 10, 000 条推文运行情绪分析需要一些时间。出于演示目的,我们将从池中随机抽取 300 条推文。
import pandas as pd
import pickle
import requests
import random
with open('world_cup_tweets.pkl', 'rb') as f:
data = pickle.load(f)
tweets = data.Tweet_processed.to_list()
tweets = random.sample(tweets, 300)
四、运行分析
然后我们将语言模型和我们的拥抱脸令牌分别传递给变量。
model = "cardiffnlp/twitter-roberta-base-sentiment-latest"
hf_token = "YOUR OWN TOKEN"
我们定义了一个采用单个参数的分析函数:数据。此函数将我们的 Twitter 数据转换为 JSON 格式,其中包含对传递给函数的输入数据的模型推理结果。
API_URL = "https://api-inference.huggingface.co/models/" + model
headers = {"Authorization": "Bearer %s" % (hf_token)}
def analysis(data):
payload = dict(inputs=data, options=dict(wait_for_model=True))
response = requests.post(API_URL, headers=headers, json=payload)
return response.json()
我们初始化一个空列表,以存储每条推文的情绪分析结果。我们对列表中的每条推文使用循环。然后我们使用 try-except 块技术:
- 对于可以分析的推文,我们调用我们定义的函数分析,将当前推文作为输入,并从返回的列表中检索第一个结果。此结果应为字典列表,每个词典都包含情绪标签和分数。我们使用内置的 max 函数在情绪结果中查找得分最高的字典。我们将一个新词典附加到tweets_analysis列表中,其中包含推文及其相应的标签,其中包含得分最高的情绪。
- 对于无法分析的推文,我们使用 except 块,该块捕获 try 块中发生的任何异常并打印错误消息。情绪分析功能可能无法分析某些推文,因此包含此块以处理这些情况。
tweets_analysis = []
for tweet in tweets:
try:
sentiment_result = analysis(tweet)[0]
top_sentiment = max(sentiment_result, key=lambda x: x['score']) # Get the sentiment with the higher score
tweets_analysis.append({'tweet': tweet, 'sentiment': top_sentiment['label']})
except Exception as e:
print(e)
然后我们可以将数据加载到数据框中并查看一些初步结果。
# Load the data in a dataframe
df = pd.DataFrame(tweets_analysis)
# Show a tweet for each sentiment
print("Positive tweet:")
print(df[df['sentiment'] == 'positive']['tweet'].iloc[0])
print("\nNeutral tweet:")
print(df[df['sentiment'] == 'neutral']['tweet'].iloc[0])
print("\nNegative tweet:")
print(df[df['sentiment'] == 'negative']['tweet'].iloc[0])
# Outputs: (edited by author to remove vulgarity)
Positive tweet:
Messi, you finally get this World Cup trophy. Happy ending and you are officially called球王
Neutral tweet:
Nicholas the Dolphin picks 2022 World Cup Final winner
Negative tweet:
Yall XXXX and this XXXX world cup omg who XXXX CARESSS
我们还应该使用 groupby 函数来查看样本中有多少推文是正数或负数。
sentiment_counts = df.groupby(['sentiment']).size()
print(sentiment_counts)
# Outputs:
sentiment
negative 46
neutral 63
positive 166
dtype: int64
既然我们在这里,为什么不使用饼图来可视化结果:
import matplotlib.pyplot as plt
fig = plt.figure(figsize=(6,6), dpi=100)
ax = plt.subplot(111)
sentiment_counts.plot.pie(ax=ax, autopct='%1.1f%%', startangle=270, fontsize=12, label="")
由作者创建
似乎大多数人对卡塔尔世界杯感到满意。伟大!
人们在正面和负面推文中谈论什么?我们可以使用词云来显示这些组中的关键字。
# pip install first if you have not installed wordcloud in your environment
from wordcloud import WordCloud
from wordcloud import STOPWORDS
# Wordcloud with positive tweets
positive_tweets = df[df['sentiment'] == 'positive']['tweet']
stop_words = ["https", "co", "RT"] + list(STOPWORDS)
positive_wordcloud = WordCloud(max_font_size=50, max_words=50, background_color="white", stopwords = stop_words).generate(str(positive_tweets))
plt.figure()
plt.title("Positive Tweets - Wordcloud")
plt.imshow(positive_wordcloud, interpolation="bilinear")
plt.axis("off")
plt.show()
# Wordcloud with negative tweets
negative_tweets = df[df['sentiment'] == 'negative']['tweet']
stop_words = ["https", "co", "RT"] + list(STOPWORDS)
negative_wordcloud = WordCloud(max_font_size=50, max_words=50, background_color="white", stopwords = stop_words).generate(str(negative_tweets))
plt.figure()
plt.title("Negative Tweets - Wordcloud")
plt.imshow(negative_wordcloud, interpolation="bilinear")
plt.axis("off")
plt.show()
由作者创建
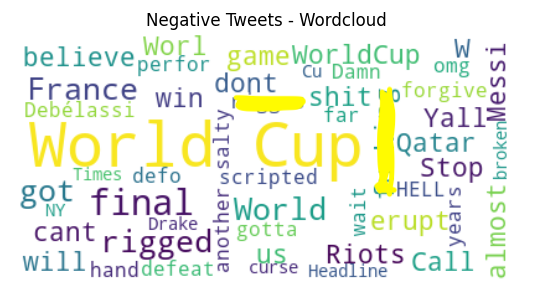
五、总结
现在我希望你已经学会了如何在拥抱脸中使用推理 API 对推文进行情感分析。这是一个功能强大的工具,高度适用于各个领域。关注我以获取更多想法和技术。