文章目录
- Transformer 的基础结构
- NLP Structure
- VIT
- SWIN
- DERT
- Transformer 常用terms
- 分块的batch-size自动计算
- Batch norm
- Layer norm
- Multihead Self Attention
- GELU/ELU/RELU
- Transformer Vs CNN
- 每个模型的详细笔记
- Vit
- 图片分割
- 自己的思考
- 计算过程
- Segmenter
- 运行 Train
- Train 里的结构
- DERT
- DERT 步骤
- segmentation数据
- segmentaion 快速测试 webcam
- DERT segmentaion 测试 webcam 结果
- Detectron 2
- DETECTRON 2 测试 webcam 结果
- 个人思考
Transformer 的基础结构
NLP Structure
VIT
SWIN
DERT
Transformer 常用terms
https://www.pinecone.io/learn/batch-layer-normalization/
https://wandb.ai/wandb_fc/LayerNorm/reports/Layer-Normalization-in-Pytorch-With-Examples—VmlldzoxMjk5MTk1
https://towardsdatascience.com/different-normalization-layers-in-deep-learning-1a7214ff71d6
https://neuralthreads.medium.com/layer-normalization-and-how-to-compute-its-jacobian-for-backpropagation-55a549d5936f
分块的batch-size自动计算
目标:图片大小84*84,求有哪些分块数量可以用?
简化:求84所有的divisors, N=84
结果:84 的 divisors 有:[1, 84, 2, 42, 3, 28, 4, 21, 6, 14, 7,12]
for loop:–>
i = 1, j=84, [1, 84]
i = 2, j =42 [1, 84, 2, 42]
i = 3, j = 28 [1, 84, 2, 42, 3, 28]
i = 4, j = 21 [1, 84, 2, 42, 3, 28, 4, 21]
i = 5, j = 21 [1, 84, 2, 42, 3, 28, 4, 21]
i = 6, j = 14 [1, 84, 2, 42, 3, 28, 4, 21, 6, 14]
i = 7, j = 12 [1, 84, 2, 42, 3, 28, 4, 21, 6, 14, 7,12]
i = 8, j = 12
i = 9, j = 12
i = 10, j = 12
i = 11, j = 12
i = 12, j = 12 结束
程序大概逻辑:
从 i= 1,j = N 开始。 i + 1: 如果 N % ( i + 1 ) = = 0 N\%(i+1)==0 N%(i+1)==0, j = N j = N j=N;如果 N % ( i + 1 ) ! = 0 N\%(i+1)!=0 N%(i+1)!=0, j = j l a s t j = j_{last} j=jlast
结束condition:i == j
Time complexity ~= O ( N ) O(\sqrt {N}) O(N)~= O ( 84 ) O(\sqrt {84}) O(84)
Batch norm
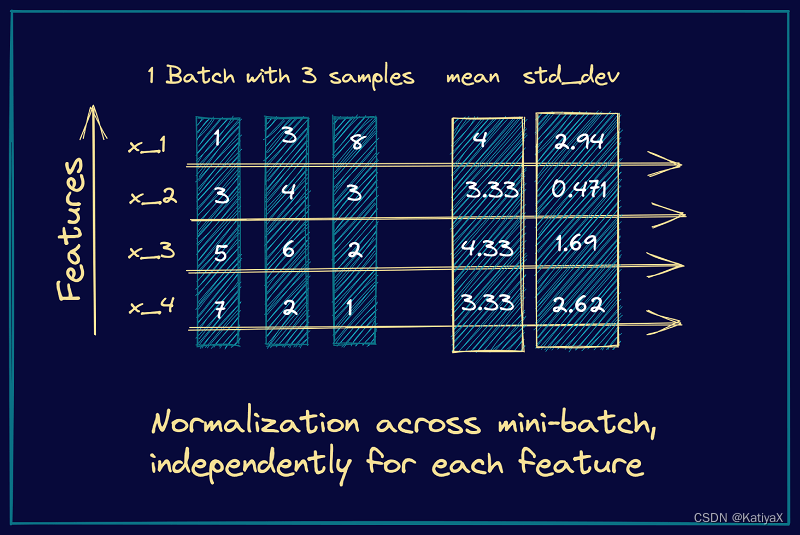
对sequence的data不好,因为sequence的长度不一,那么batch norm用的mean 和 std 就不能很好的将每个feature的分布准确算出。
在NLP类似Sequence的问题上,batch norm 只适用于长度一样的数据。不然Activate function没办法让gradient converge,有时还会有死掉的状况。
例如有数据:
随着像这样pattern的数据放进模型训练,上面算式里求平均数的
μ
\mu
μ会因为长度各种变化,导致得到的mean其实不能代表所有数据,让模型变得不准确。
feature0 | feature1 | feature2 | feature3 | feature4 |
---|---|---|---|---|
A | A | A | A | A |
A | B | A | C | A |
A | C | B | D | A |
A | C | |||
B | C | |||
D |
就算给他们padding了 0,会因为引入太多0,最后结果可能会死掉
feature0 | feature1 | feature2 | feature3 | feature4 |
---|---|---|---|---|
A | A | A | A | A |
A | B | A | C | A |
A | C | B | D | A |
A | C | 0 | 0 | 0 |
B | C | 0 | 0 | 0 |
D | 0 | 0 | 0 | 0 |
Transformer 也不适用batch norm,Batch由GPU操作,但是一般的GPU顶多2个Batch,按照上一个内容,计算出12,这样的Batch size,对GPU来说为难了。因此Transformer应该选用Layer Norm去让模型达到一个加快Converge的操作。
Layer norm
一般用于任何和RNN有关的网络结构。对Sequence data 友好。对大Batch size的Transformer友好。
就是平分一个layer上,各个hidden unit的值。
Multihead Self Attention
Q: Query 当作 一个 submmit button (选红色和绿色球)
K: Key 是训练过程中的字典,当作备选项 (各种颜色的球)
V: 用于训练过程中的更新
以上三个都是linear layer代替
训练时候,第一次,Decoder Input 的 Q,K使用 Encoder 输出的Q,K。 第n次,Decoder 的 Input用 n-1 次的Q,K, V
同时,从Encoder 输出的Q,K需要传到 Decoder, 更新 Decoder 这边第二个Multi-Head Attention的 Q,K。
Filtered image = Attention Filter(来自Transfomer Decoder的输出) * Original Image
GELU/ELU/RELU
使用了Gaussian Distribution. 数据计算用到了distribution的面积计算,即CFD。中间步骤有用到 Gauss Error Function.
以上是数学里的计算方式,但是在代码里,以上步骤不好操作,因此,目前的GELU是通过sigmoid 或者 Tahn 估计出来的。
Transformer Vs CNN
Transformer能够得到整张图上的cross information,但是CNN不行。因为CNN只是按照sliding Windows一步一步的滑动,没有让每步sliding Windows与走过的sliding Windows作相关性的计算。而Transformer因为有分块的设计,并且会计算每个分块与其它各个分块的相似度,使得模型有了cross information。
CNN 是 Transformer的一个子集
每个模型的详细笔记
Vit
图片分割
def img_to_patch(x, patch_size, flatten_channels=True):
"""
Inputs:
x - torch.Tensor representing the image of shape [B, C, H, W]
patch_size - Number of pixels per dimension of the patches (integer)
flatten_channels - If True, the patches will be returned in a flattened format
as a feature vector instead of a image grid.
"""
B, C, H, W = x.shape
x = x.reshape(B, C, H//patch_size, patch_size, W//patch_size, patch_size)
x = x.permute(0, 2, 4, 1, 3, 5) # [B, H', W', C, p_H, p_W]
x = x.flatten(1,2) # [B, H'*W', C, p_H, p_W]
if flatten_channels:
x = x.flatten(2,4) # [B, H'*W', C*p_H*p_W]
return x
x 为输入的数据,例如有4 张 RGB的图片,每张图片都是32x32
那么x.shape = [4,3,32,32]
假设patch_size = 4, 那么这4张RGB会被分割成64份,因为:
份数结果 =
o
l
d
W
patch size for W
∗
o
l
d
H
patch size for H
\frac{old W}{\text{patch size for W}}*\frac{old H}{\text{patch size for H}}
patch size for WoldW∗patch size for HoldH
因此当W,H都有patch size = 4,份数结果=16
因此当W,H都有patch size = 16,份数结果=4
这里的patch size 得确保能被整除。
自己的思考
分割的份数对Transformer有什么影响?
好处:
由于,后续在Transofomer的encoder中会计算每个分块与其它所有分块的关系,也许对于画面细节的对应上会更优秀。而分的大块可能会错失细节信息。
比CNN好在它可以和画面其它部分作关联。
坏处:
分割的越多,computation time complexity越大
计算过程
Segmenter
运行 Train
Steps:
- 按照readme 把路径和数据下载好
- 把Script里带segm的import 引用找到,并删掉前缀
比如:from segm.utils.distributed import sync_model
改成from utils.distributed import sync_model
- 找到train.py
@click.option("--log-dir", type=str, help="logging directory",default="seg_tiny_mask")
@click.option("--dataset", type=str,default="ade20k")
@click.option("--backbone", default="vit_tiny_patch16_384", type=str)
@click.option("--decoder", default="mask_transformer", type=str)
- 如果用的Windows电脑,会报错:
Windows RuntimeError: Distributed package doesn‘t have NCCL built in
因此要改代码,把backend赋值为gloo
dist.init_process_group(backend='gloo')
#这里是报错的地方,但是源代码的错误根本在main function
找到train.py:
main function 里的第一行(line 71)更改为distributed.init_process(backend='gloo')
- 如果是单片GPU,建议将batch_size 换成 1.
- 然后可以train了。
Train 里的结构
DERT
DERT 步骤
segmentation数据
下载:
wget http://images.cocodataset.org/annotations/panoptic_annotations_trainval2017.zip
下好的包裹里有以下文件的ZIP,ZIP打开后,将文件夹按一下排列:
- coco
- panoptic_trainval2017
- annotations
- panoptic_train2017.json
- panoptic_val2017.json
- panoptic_train2017
- panoptic_val2017
–coco_path :E:\coco
–coco_panoptic_path :E:\coco\panoptic_trainval2017
–dataset_file: panoptic_trainval2017 (不太确定)
–output_dir: /output/path/box_model
segmentaion 快速测试 webcam
import io
from PIL import Image
import numpy
import torch
from typing import List
import cv2
import torchvision.transforms as T
import panopticapi
from panopticapi.utils import id2rgb, rgb2id
import itertools
import seaborn as sns
palette = itertools.cycle(sns.color_palette())
if __name__ == '__main__':
# standard PyTorch mean-std input image normalization
transform = T.Compose([
T.ToTensor(),
T.Normalize([0.485, 0.456, 0.406], [0.229, 0.224, 0.225])
])
# model, postprocessor = detr_resnet50_panoptic(pretrained=False,return_postprocessor=True,).eval()
model, postprocessor = torch.hub.load('facebookresearch/detr', 'detr_resnet101_panoptic', pretrained=True, return_postprocessor=True, num_classes=250)
model.eval()
ret = False
cap = cv2.VideoCapture(0)
while True:
ret, im = cap.read()
if ret == False:
break
cv2.imshow('',im)
im = Image.fromarray(im)
img = transform(im).unsqueeze(0)
out = model(img)
result = postprocessor(out, torch.as_tensor(img.shape[-2:]).unsqueeze(0))[0]
panoptic_seg = Image.open(io.BytesIO(result['png_string']))
panoptic_seg = numpy.array(panoptic_seg, dtype=numpy.uint8).copy()
panoptic_seg_id = rgb2id(panoptic_seg)
panoptic_seg[:, :, :] = 0
for id in range(panoptic_seg_id.max() + 1):
panoptic_seg[panoptic_seg_id == id] = numpy.asarray(next(palette)) * 255
cv2.imshow('seg',panoptic_seg)
key = cv2.waitKey(1)
if key == ord('q'):
break
DERT segmentaion 测试 webcam 结果
在我1个GPU的机子上测试:
Inference 耗时:0.08(平均)
从Inference的结果组合结果耗时:0.0145 (平均)
Detectron 2
import io
from PIL import Image
import numpy
import torch
from typing import List
from detectron2.config import get_cfg
from detectron2.utils.visualizer import Visualizer
from detectron2.data import MetadataCatalog
from copy import deepcopy
import cv2
import torchvision.transforms as T
import panopticapi
from panopticapi.utils import id2rgb, rgb2id
import itertools
import seaborn as sns
palette = itertools.cycle(sns.color_palette())
import time
if __name__ == '__main__':
# standard PyTorch mean-std input image normalization
transform = T.Compose([
T.ToTensor(),
T.Normalize([0.485, 0.456, 0.406], [0.229, 0.224, 0.225])
])
# model, postprocessor = detr_resnet50_panoptic(pretrained=False,return_postprocessor=True,).eval()
model, postprocessor = torch.hub.load('facebookresearch/detr', 'detr_resnet101_panoptic', pretrained=True, return_postprocessor=True, num_classes=250)
model.eval()
ret = False
cap = cv2.VideoCapture(0)
while True:
ret, im = cap.read()
if ret == False:
break
cv2.imshow('',im)
im = Image.fromarray(im)
img = transform(im).unsqueeze(0)
out = model(img)
start_time = time.time()
result = postprocessor(out, torch.as_tensor(img.shape[-2:]).unsqueeze(0))[0]
print("--- %s seconds 1 ---" % (time.time() - start_time))
start_time = time.time()
segments_info = deepcopy(result["segments_info"])
panoptic_seg = Image.open(io.BytesIO(result['png_string']))
final_w, final_h = panoptic_seg.size
panoptic_seg = numpy.array(panoptic_seg, dtype=numpy.uint8)
panoptic_seg = torch.from_numpy(rgb2id(panoptic_seg))
meta = MetadataCatalog.get("coco_2017_val_panoptic_separated")
for i in range(len(segments_info)):
c = segments_info[i]["category_id"]
segments_info[i]["category_id"] = meta.thing_dataset_id_to_contiguous_id[c] if segments_info[i]["isthing"] else meta.stuff_dataset_id_to_contiguous_id[c]
v = Visualizer(numpy.array(im.copy().resize((final_w, final_h)))[:, :, ::-1], meta, scale=1.0)
v._default_font_size = 20
v = v.draw_panoptic_seg_predictions(panoptic_seg, segments_info, area_threshold=0)
print("--- %s seconds 2 ---" % (time.time() - start_time))
cv2.imshow('seg',v.get_image())
key = cv2.waitKey(1)
if key == ord('q'):
break
DETECTRON 2 测试 webcam 结果
这里和之前DERT不同于,这里加入了meta的语义信息,速度其实和之前的相差不大。
在我1个GPU的机子上测试:
Inference 耗时:0.08(平均)
从Inference的结果组合结果耗时:0.0145 (平均)
个人思考
因为这里测试的都是全景的panopticap, Inference的耗时会大一些,如果有指定训练的几个类别的instance segmentation, Inference 应该会和检测差不多,个人保守估计(乐观估计?)0.03 每个frame.