导语
T_T之前似乎发过类似的文章,那时候是用Keras实现的,现在用的PyTorch,而且那时候发的内容感觉有些水,于是我决定。。。
好吧我确实只是为了写点PyTorch练手然后顺便过来水一篇美文~~~
利用Python实现图像风格的迁移!!!
不喜欢过程同学的依旧可以直接下拉到最后看结果~
Let's Go!
参考资料
链接:
http://pytorch.org/tutorials/advanced/neural_style_tutorial.html#
是的,这又是来自于PyTorch官网的一个教程。
在相关文件中我依旧提供了我翻译好的版本~~~
以及涉及到的论文~~~
相关文件
网盘下载链接: https://pan.baidu.com/s/1eDOTzd0uzNzzQDRbpDEd2A
密码: tv5i
开发工具
Python版本:3.6.4
相关模块:torch模块;PIL模块;matplotlib模块;torchvision模块;以及一些Python自带的模块。
torch版本:0.3.0
环境搭建
安装Python并添加到环境变量,pip安装需要的相关模块即可。
补充说明:
PyTorch暂时不支持直接pip安装。
有两个选择:
(1)安装anaconda3后在anaconda3的环境下安装(直接pip安装即可);
(2)使用编译好的whl文件安装,下载链接为:
https://pan.baidu.com/s/1dF6ayLr#list/path=%2Fpytorch
额外说明
T_T相关文件中提供了所需的预训练模型,若嫌弃官网的下载速度,可以下载我的。
下载之后放到类似下图路径的文件夹中:
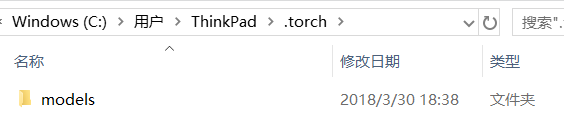
原理简介
作为一个纯正的非艺术生,抱歉我真的没法解释什么叫图像的艺术风格。反正齐白石和梵高的画肯定不是一个style的就是了。
那么我来尝试解释一下风格迁移吧:
风格迁移的实质是保留原画内容的基础上,用另外一种style来呈现原画。
那么如何量化呢?
简单而言大概是这样的:
利用CNN逐层提取图像的特征(层越靠后提取出的特征越高级和稳定,即更能表现图片的高级语义信息),并且将某一层或某几层输出的Gram矩阵作为损失函数,来衡量两幅图像之间的内容/风格差异(T_T就是两幅图像分别经过相同的卷积神经网络,比较某一层或者某几层输出的Gram矩阵的差异)。
Gram矩阵是啥???
具体而言,其计算方式为:
我们都知道,一张图片在某个卷积层的输出特征为一个形如(batch_size, channels, width, height)的四阶张量,显然batch_size为1。
我们将类似下图的特征:
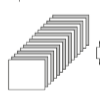
转换为(batch_size*channels, width*height)大小的矩阵,这个矩阵和它的转置相乘就可以得到一个大小为(batch_size*channels, batch_size*channels)的矩阵,这个矩阵即为Gram矩阵。
其实这就算是定义了图像风格和图像内容这两个概念了,接下来我们就可以实现风格迁移了。
其流程大概是这样的:
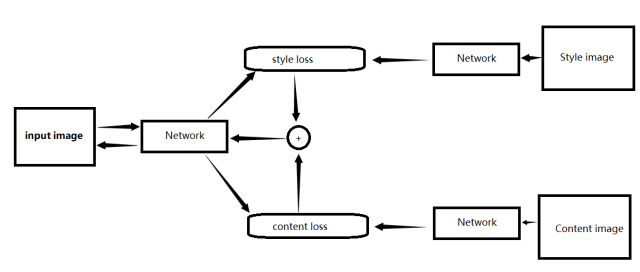
具体的实现细节详见源代码吧~~~
相关文件中也有官网教程的个人翻译版以及相关的论文供感兴趣者参考。
使用方式
修改下图所示处的图片路径为自己的图片路径:

在cmd窗口运行Neural_Transfer.py文件即可。
由于资源和时间有限,下面展示的结果我都只跑了几百轮左右,图像大小为256*256。
梵高笔下的皮卡丘
原图像:
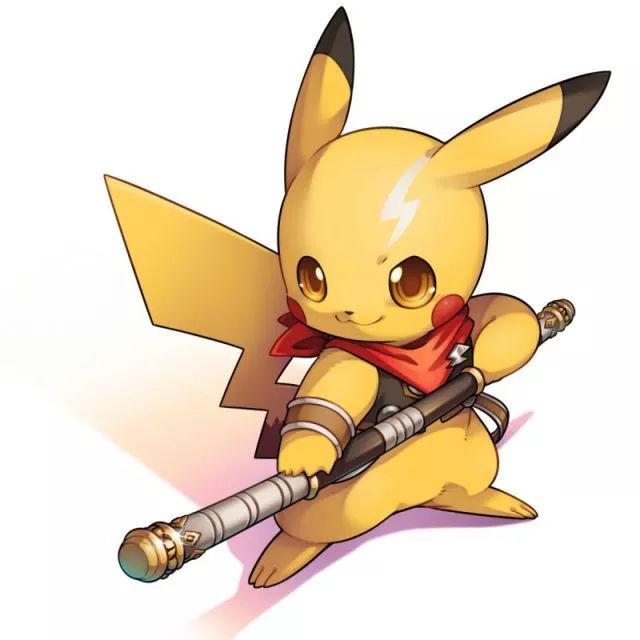
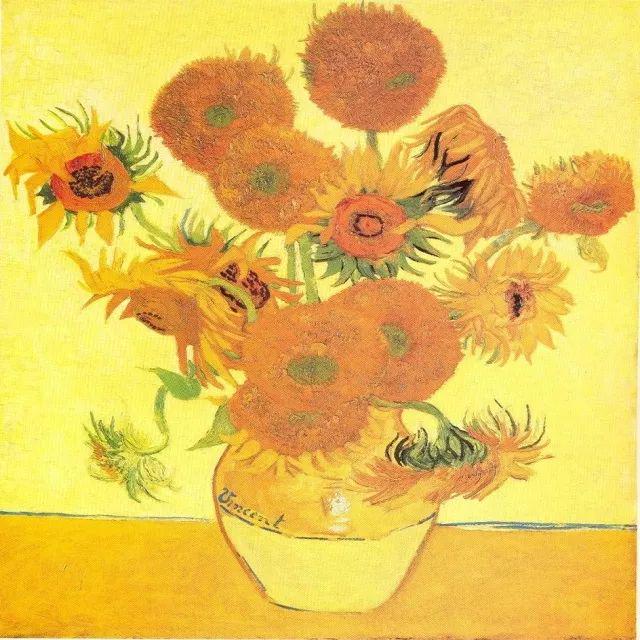
生成的图像:
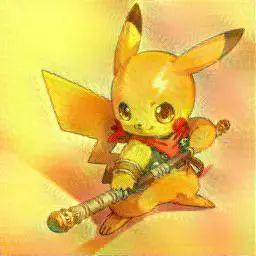
梵高笔下的老北京
原图像:
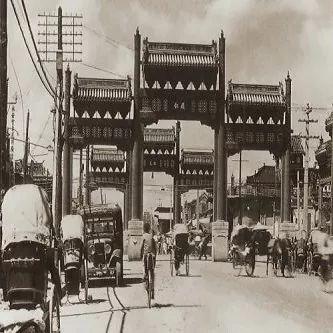
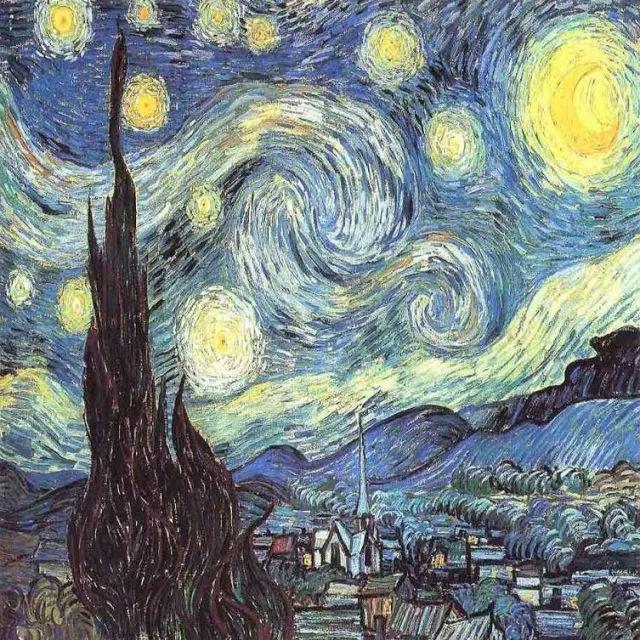
生成的图像:
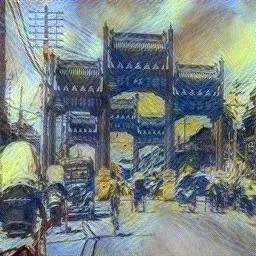
毕加索笔下的爱因斯坦
原图像:
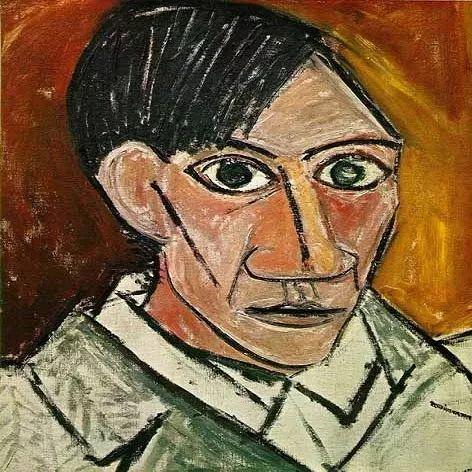
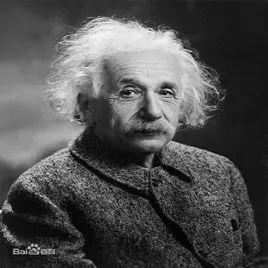
生成的图像:
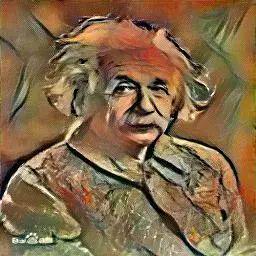
齐白石笔下的西湖
原图像:
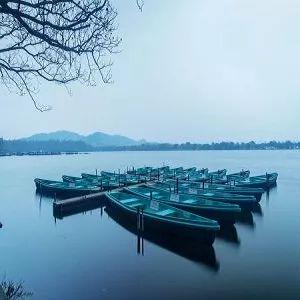
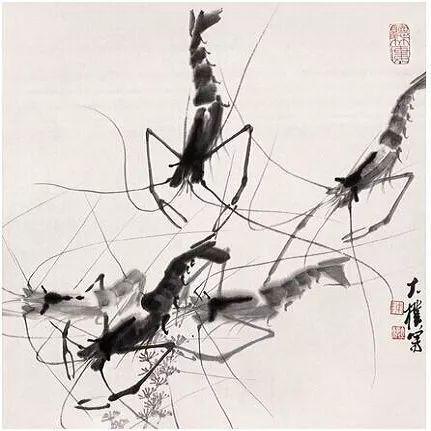
生成的图像:
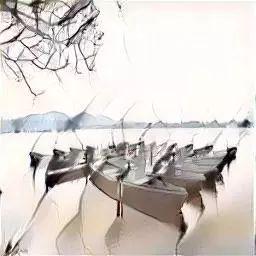