一、zeppelin的安装
zeppelin解压后进入到conf配置文件界面。
修改zeppelin-site.xml
[root@hadoop02 conf]# cp zeppelin-site.xml.template zeppelin-site.xml
[root@hadoop02 conf]# vim zeppelin-site.xml
将IP地址和端口号设置成自己的
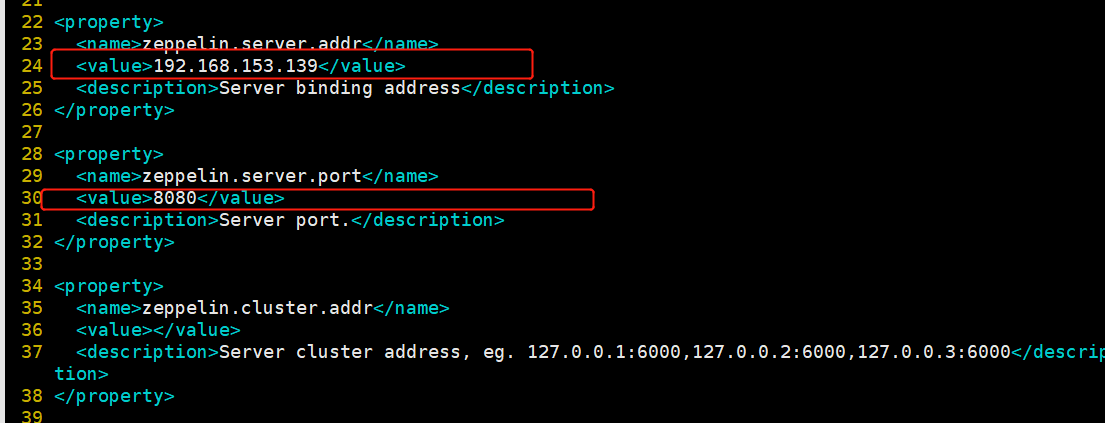
修改 zeppelin-env.sh
export JAVA HOME=/opt/soft/jdk180
export HADOOP HOME=/opt/soft/hadoop313
export HADOOP CONF DIR=/opt/soft/hadoop313/etc/hadoop
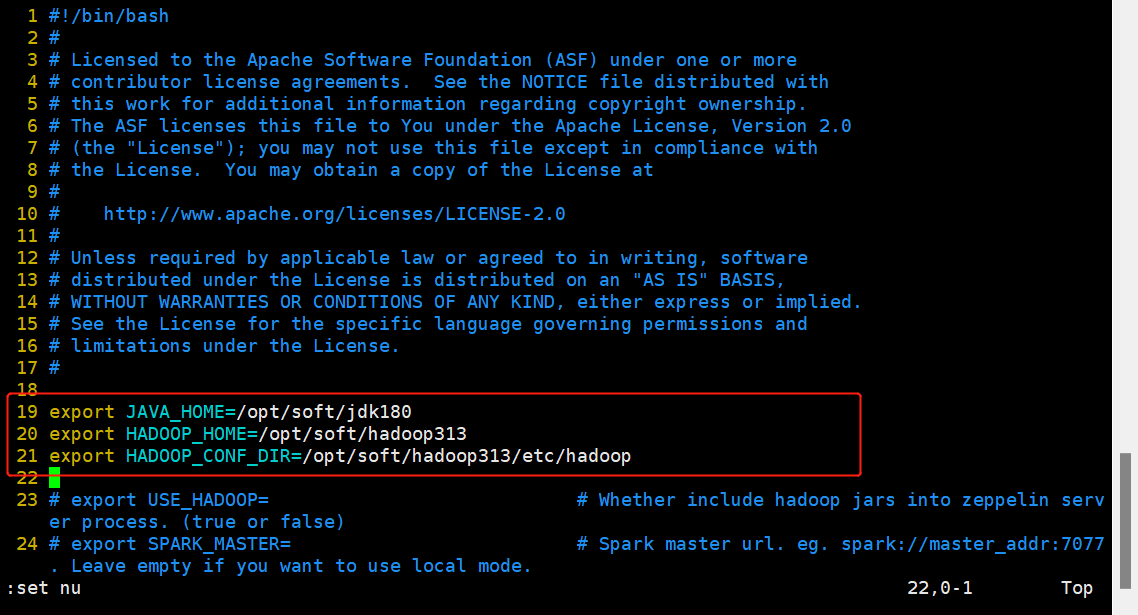
将hive-site.xml拷贝到zeppelin中
[root@hadoop02 conf]# cp /opt/soft/hive312/conf/hive-site.xml /opt/soft/zeppelin/conf
添加jar包
路径切换到/opt/soft/zeppelin/interpreter/jdbc下,添加如下jar包:
[root@hadoop02 jdbc]# cp /opt/soft/hadoop313/share/hadoop/common/hadoop/common-3.1.3.jar ./
[root@hadoop02 jdbc]# cp /opt/soft/hive312/lib/curator-client-2.12.0.jar ./
[root@hadoop02 jdbc]# cp /opt/soft/hive312/lib/guava-27.0-jre.jar ./
[root@hadoop02 jdbc]# cp /opt/soft/hive312/lib/hive-jdbc-3.1.2.jar ./
[root@hadoop02 jdbc]# cp /opt/soft/hive312/lib/hive-serde-3.1.2.jar ./
[root@hadoop02 jdbc]# cp /opt/soft/hive312/lib/hive-service-3.1.2.jar ./
[root@hadoop02 jdbc]# cp /opt/soft/hive312/lib/hive-service-rpc-3.1.2.jar ./
[root@hadoop02 jdbc]# cp /opt/soft/hive312/lib/libthrift-0.9.3.jar ./
[root@hadoop02 jdbc]# cp /opt/soft/hive312/lib/protobuf-java-2.5.0jar ./
添加外部jar包:

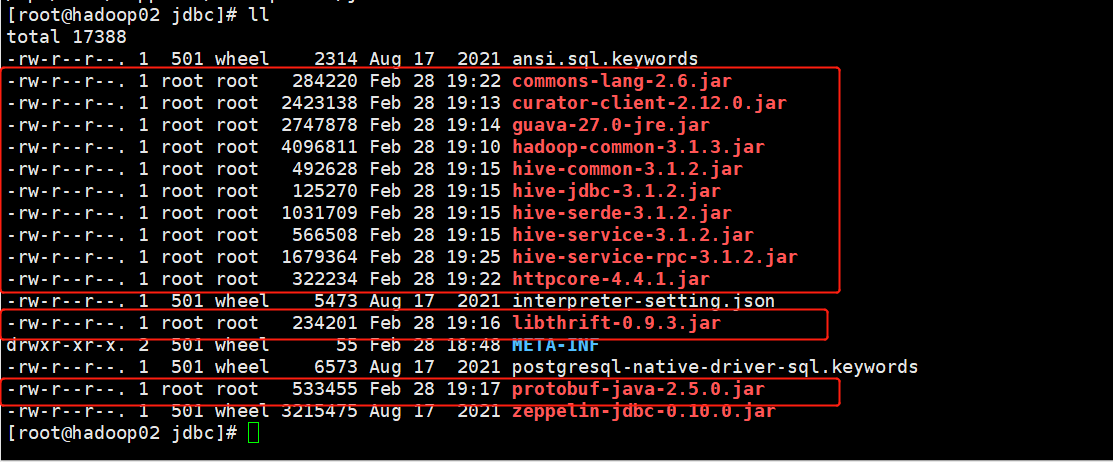
配置profile文件
# ZEPPELIN_HOME
export ZEPPELIN_HOME=/opt/soft/zeppelin
export PATH=$PATH:$ZEPPELIN_HOME/bin
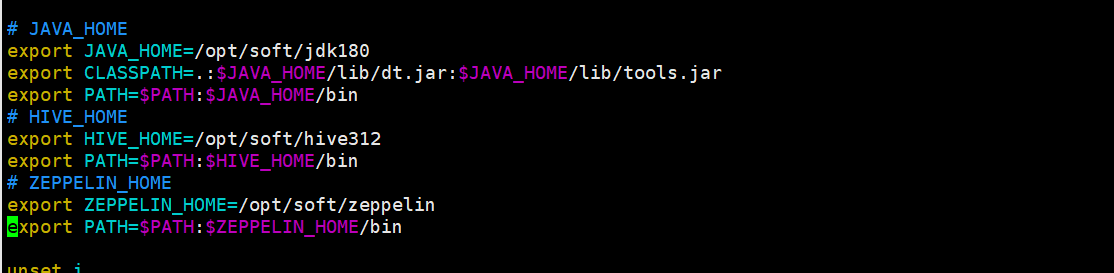
启动zeppelin
[root@hadoop02 jdbc]# zeppelin-daemon.sh start
打开浏览器
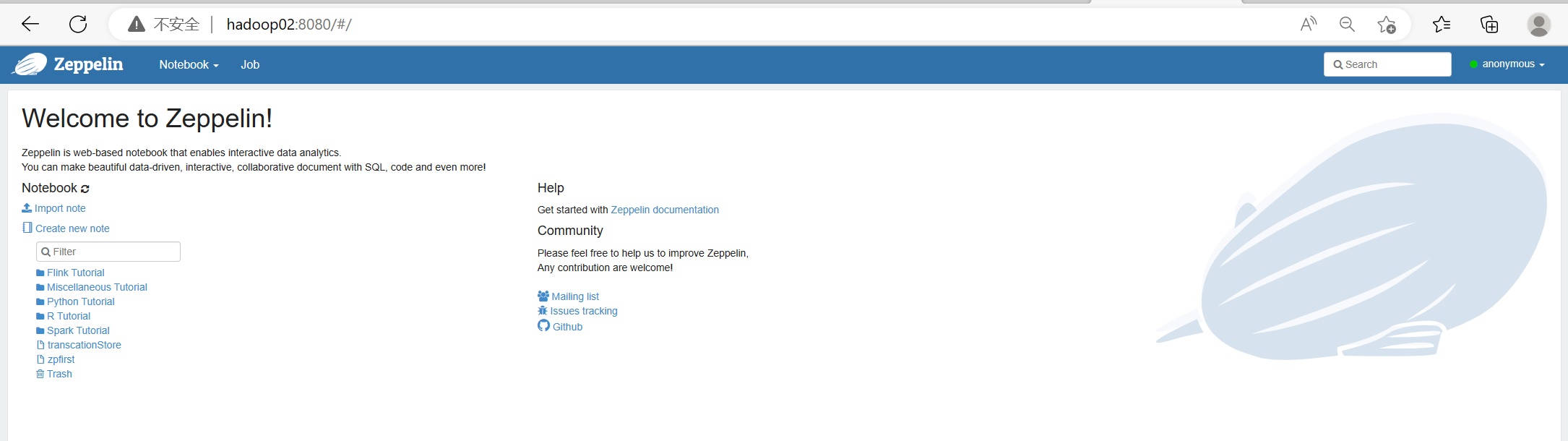
二、数据结构
数据准备
表数据存放在本地的/opt/stufile/storetransaction中。

1. 检查行数
[root@hadoop02 storetransaction]# wc -l customer_details.csv
501 customer_details.csv
2. 查看文件header行
[root@hadoop02 storetransaction]# head -n 2 customer_details.csv
customer_id,first_name,last_name,email,gender,address,country,language,job,credit_type,credit_no
1,Spencer,Raffeorty,sraffeorty0@dropbox.com,Male,9274 Lyons Court,China,Khmer,Safety Technician III,jcb,3589373385487669
创建对应目录
1. 创建数据库
drop database if exists shopping cascade;
create database if not exists shopping;
2. hdfs中创建目录,用于保存数据表
hdfs dfs -mkdir -p /shopping/data/customer
hdfs dfs -mkdir -p /shopping/data/store
hdfs dfs -mkdir -p /shopping/data/review
hdfs dfs -mkdir -p /shopping/data/transcation
3. 将本地数据上传到hdfs
hdfs dfs -put ./customer_details.csv /shopping/data/customer
hdfs dfs -put ./store_details.csv /shopping/data/store
hdfs dfs -put ./store_review.csv /shopping/data/review
hdfs dfs -put ./transaction_details.csv /shopping/data/transcation
4. 查看hdfs目录中保存的文件
hdfs dfs -ls /shopping/data/customer
hdfs dfs -ls /shopping/data/review
hdfs dfs -ls /shopping/data/store
hdfs dfs -ls /shopping/data/transcation
建表语句
创建外部表,表相关信息存储在hdfs中。
1. 顾客详细表
create external table if not exists ext_customer_details(
customer_id string,
first_name string,
last_name string,
email string,
gender string,
address string,
country string,
language string,
job string,
credit_type string,
credit_no string
)
row format serde 'org.apache.hadoop.hive.serde2.OpenCSVSerde'
location '/shopping/data/customer'
tblproperties('skip.header.line.count'='1');
2. 交易信息表
create external table if not exists ext_transaction_details(
transaction_id string,
customer_id string,
store_id string,
price decimal(8,2),
product string,
purchase_date string,
purchase_time string
)
row format serde 'org.apache.hadoop.hive.serde2.OpenCSVSerde'
location '/shopping/data/transcation'
tblproperties('skip.header.line.count'='1');
3. 店铺信息表
create external table if not exists ext_store_details(
store_id string,
store_name string,
employee_number string
)
row format serde 'org.apache.hadoop.hive.serde2.OpenCSVSerde'
location '/shopping/data/store'
tblproperties('skip.header.line.count'='1');
4. 评分表
create external table if not exists ext_review_details(
transaction_id string,
store_id string,
review_score string
)
row format serde 'org.apache.hadoop.hive.serde2.OpenCSVSerde'
location '/shopping/data/review'
tblproperties('skip.header.line.count'='1');
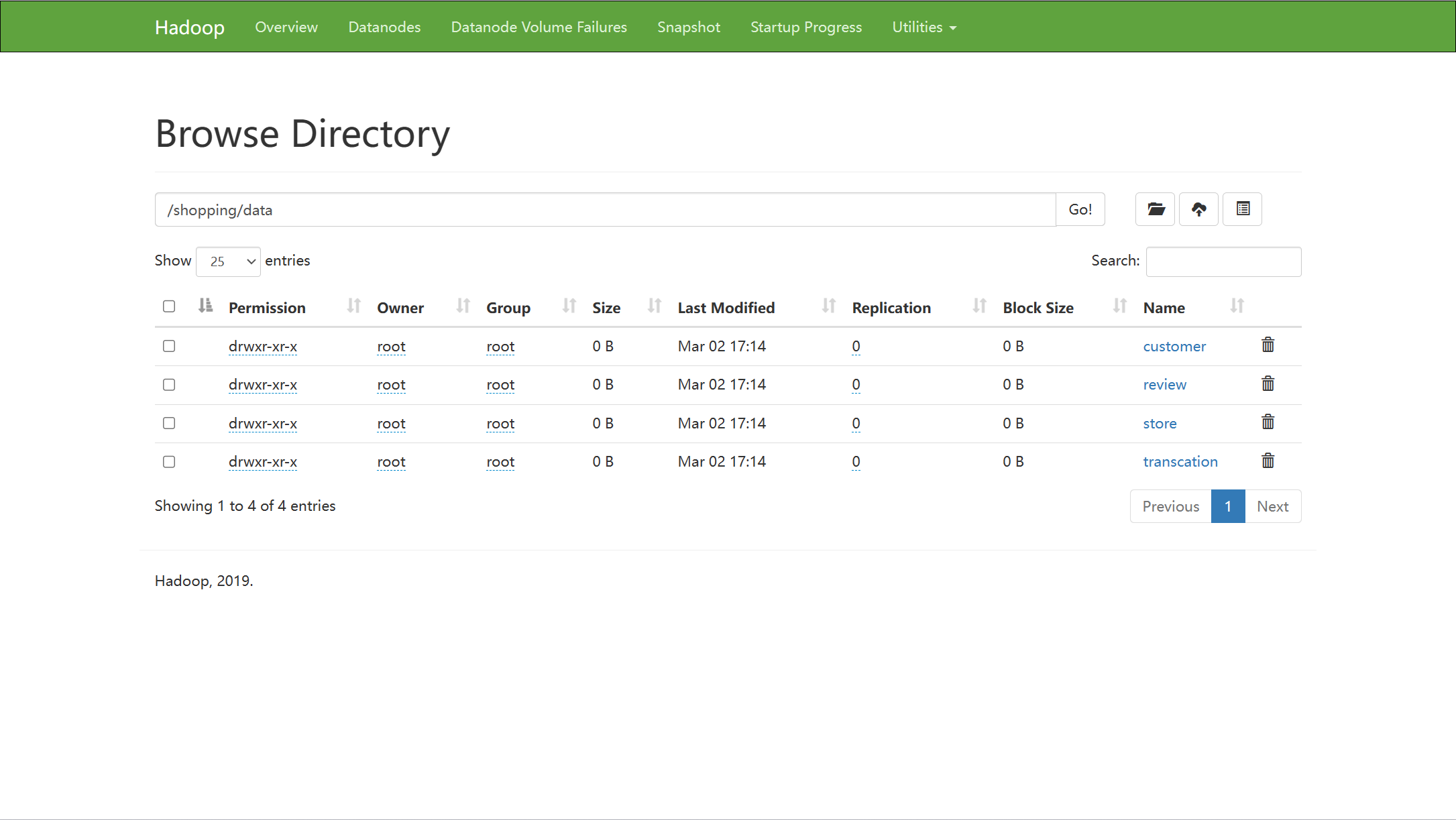
三、数据清洗
数据脱敏
在customer表中,email字段、address字段、credit_no字段不希望被显示为明文,需要对其进行加密。
drop view if exists vw_customer_details;
create view if not exists vw_customer_details as
select
customer_id,first_name,unbase64(last_name) as last_name,
unbase64(email) as email, gender,unbase64(address) as address,
country,job,credit_type,
unbase64(concat(unbase64(credit_no),'hello')) as credit_no
from ext_customer_details;
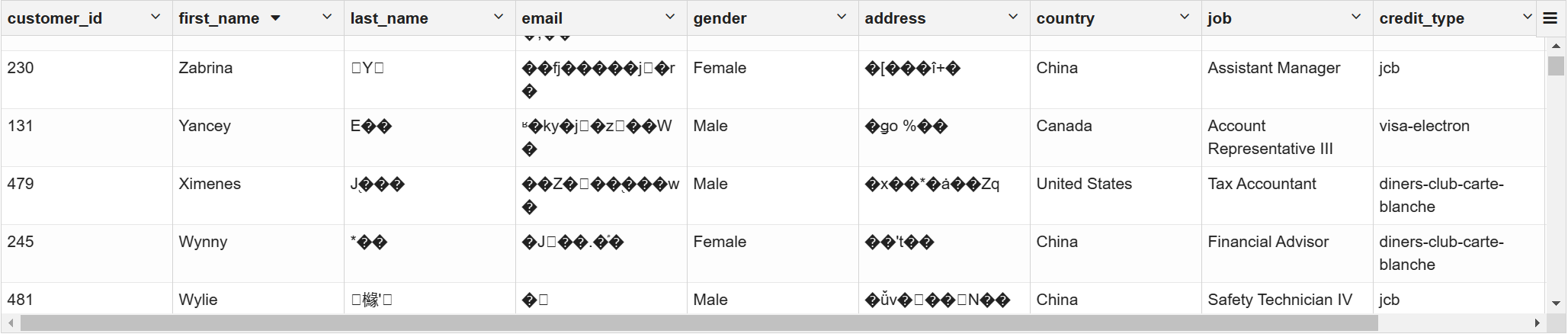
去除重复值
with
basetb as (select row_number()over(partition by transaction_id) as rn,
transaction_id,customer_id,store_id,price,product,purchase_date,purchase_time,
substr(purchase_date,0,7) purchase_month from ext_transaction_details),
basetb2 as (select if(rn=1,transaction_id,concat(transaction_id,'_fix',rn)) transaction_id ,
customer_id,store_id,price,product,purchase_date,purchase_time,purchase_month from basetb)
select * from basetb2 where transaction_id like '%fix%' limit 100;
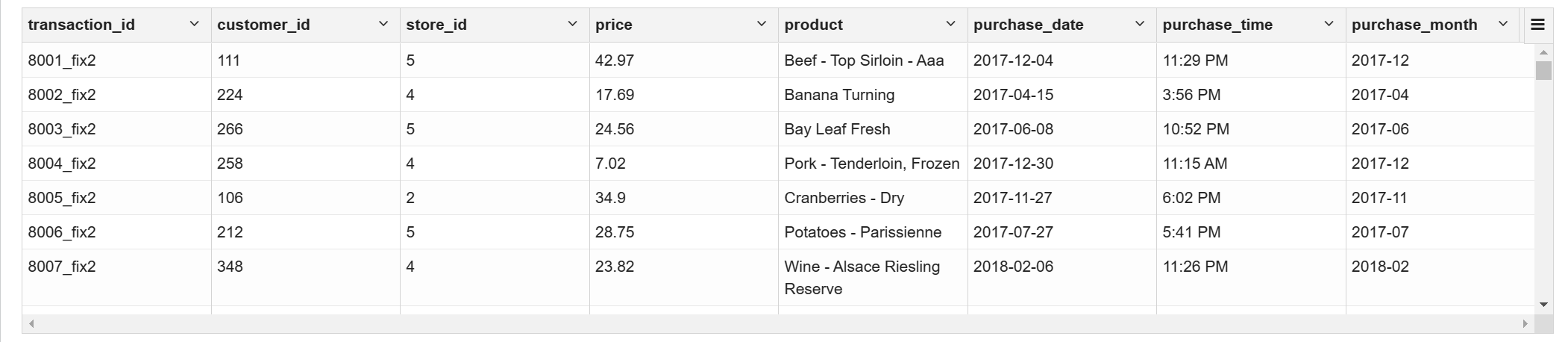
过滤掉缺失内容
create view if not exists vm_store_review as
select * from ext_store_review where review_score <> '';
四、数据分析
Customer分析
1.1 找出顾客最常用的信用卡
select
credit_type, count(customer_id)
from ext_customer_details group by credit_type;
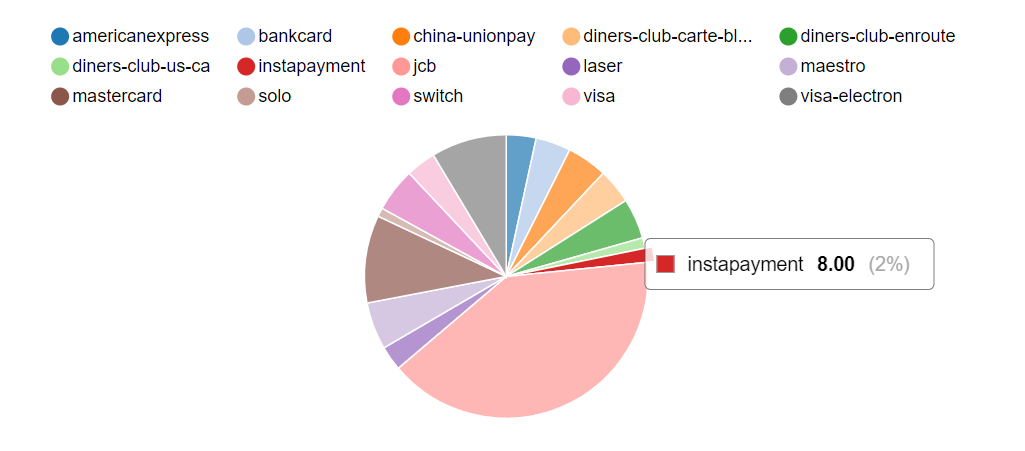
1.2 找出客户资料中排名前五的职位名称
select
credit_type,count(credit_type) count_credit_type
from vw_customer_details group by credit_type order by credit_type having gender='female';
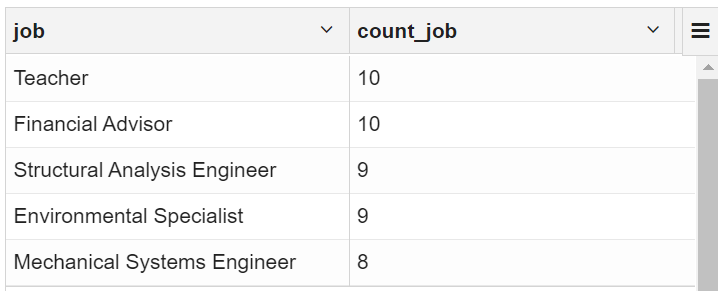
1.3 在美国女性最常用的信用卡
select
credit_type,count(credit_type) count_credit_type
from vw_customer_details
where gender='Female' and country='United States'
group by credit_type
order by count_credit_type desc limit 3 ;
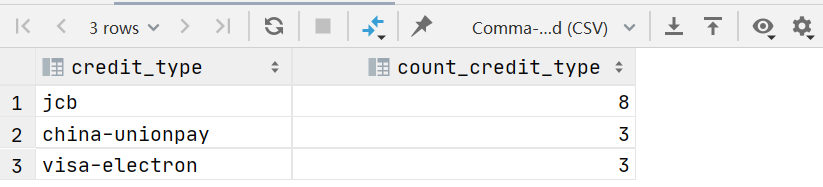
1.4 按性别和国家进行客户统计
select
gender,country,count(*)
from vw_customer_details group by gender,country;
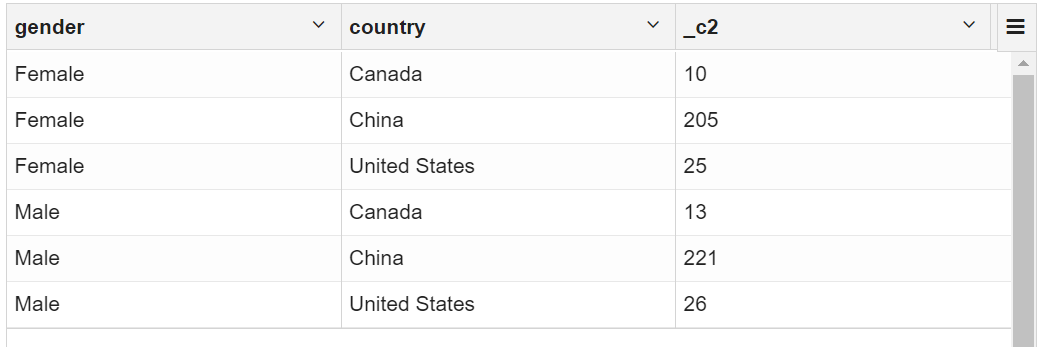
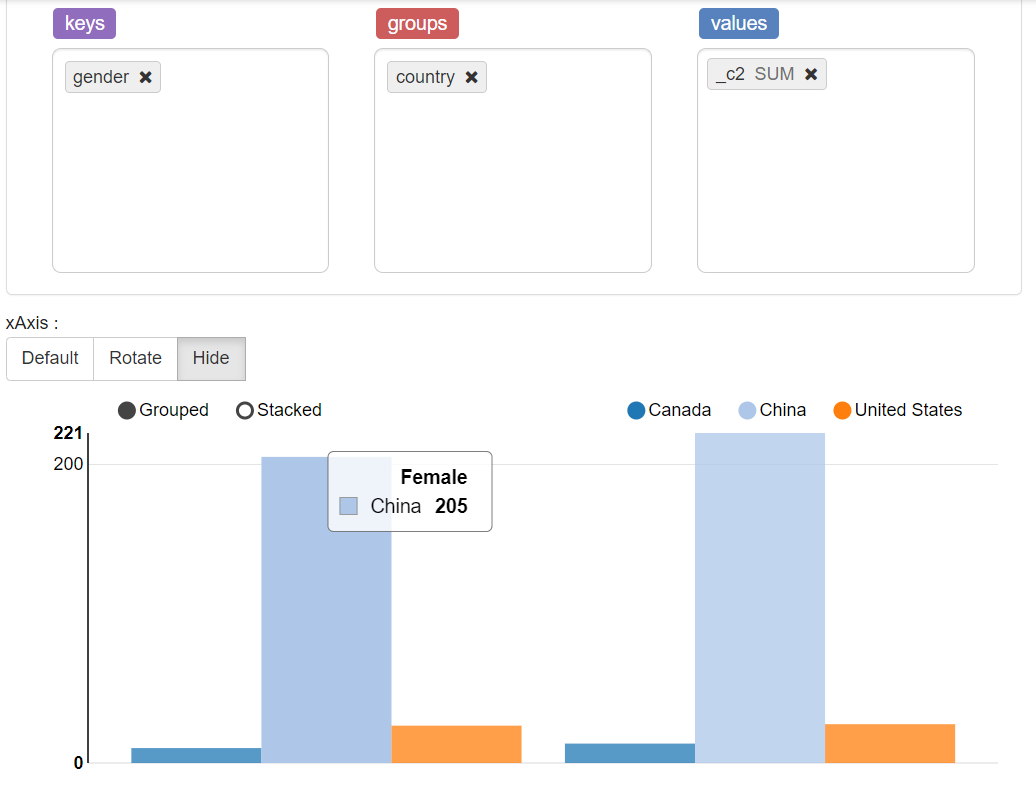
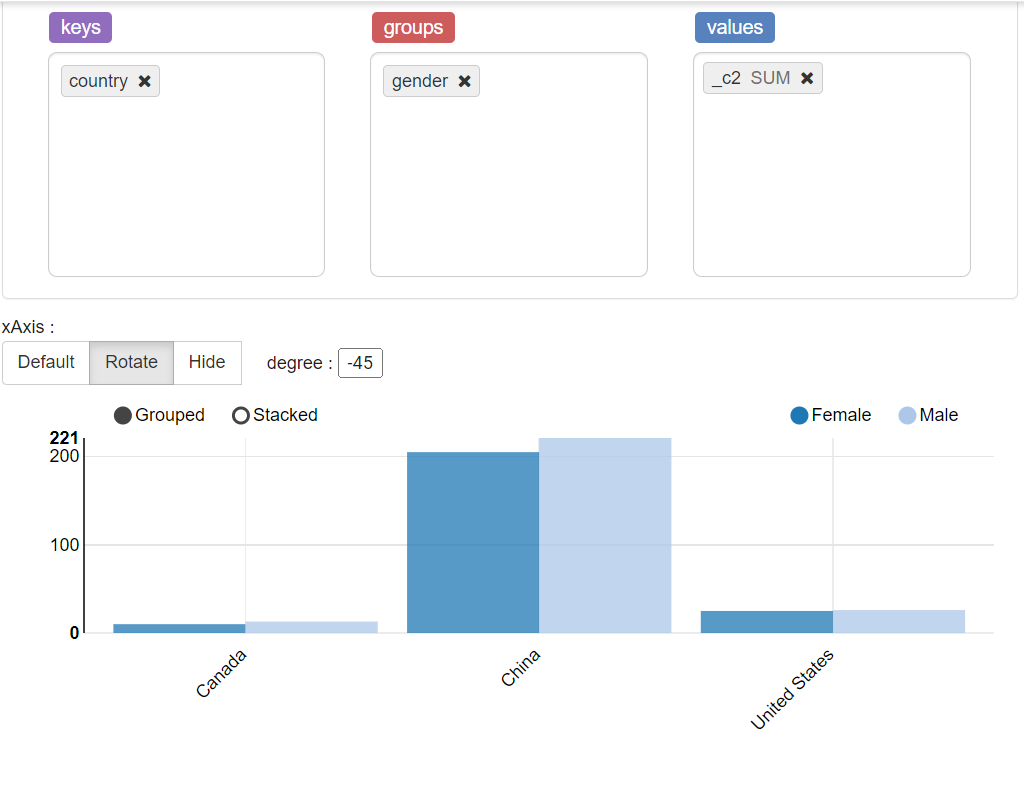
Transaction分析
2.1 计算每月总收入
select
purchase_month,sum(price)
from transaction_details group by purchase_month;
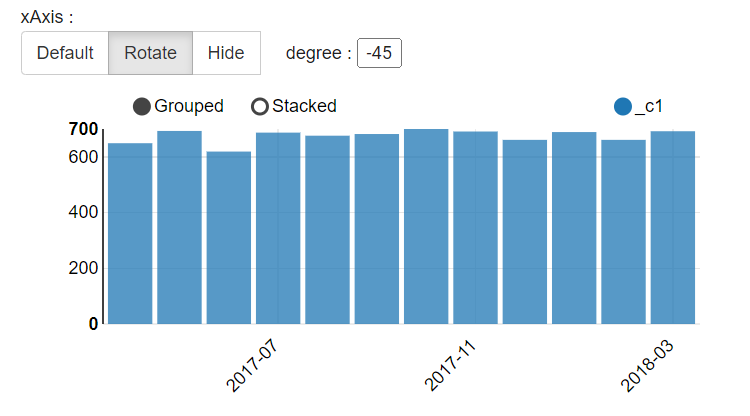
2.2 计算每个季度的总收入
with t2 as (
select concat_ws('-',cast(year(purchase_date) as string),cast(ceil(month(purchase_date)/3) as string)) as concat_quarter1,price
from transaction_details)
select concat_quarter1,sum(price) from t2 group by concat_quarter1;
或者直接使用季度函数
with t2 as (
select concat_ws('-',cast(year(purchase_date) as string),cast(ceil(month(purchase_date)/3) as string)) as concat_quarter1,price
from transaction_details)
select concat_quarter1,sum(price) from t2 group by concat_quarter1;

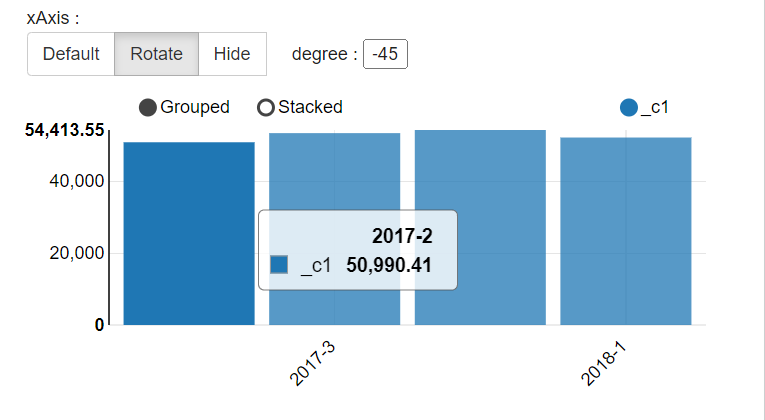
2.3 按年计算总收入
select
year(purchase_date),sum(price)
from transaction_details group by year(purchase_date);

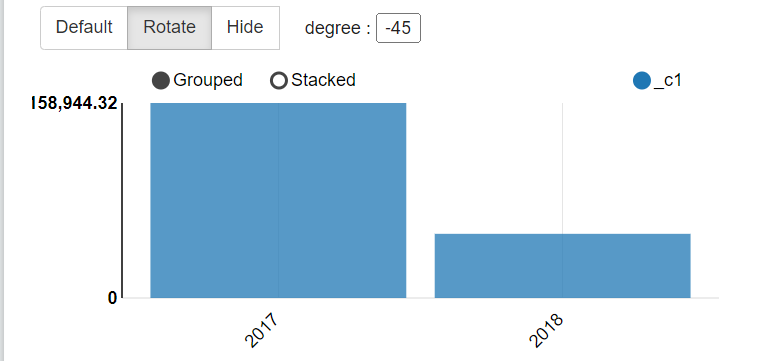
2.4 按工作日计算总收入
select
dayofweek(purchase_date) week_day,sum(price)
from transaction_details
where dayofweek(purchase_date) between 1 and 5
group by dayofweek(purchase_date);
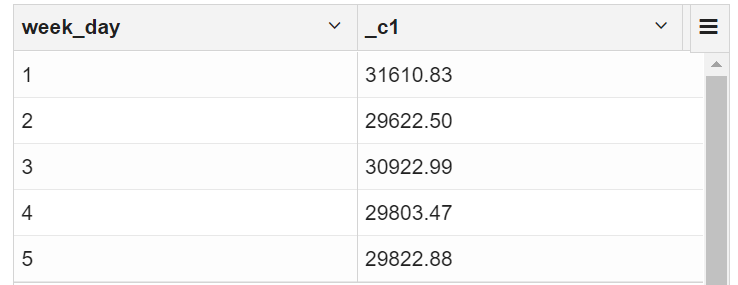
按照工作日、月、季度、年计算总收入
with basetb as(
SELECT
price,
dayofweek(purchase_date) as weekday,
month(purchase_date) as month,
concat_ws('-',cast(year(purchase_date) as string),cast(ceil(month(purchase_date)/3) as string)) as quarter,
year(purchase_date) as year
from transaction_details)
select sum(price) as sumMoney,weekday,month,quarter,year from basetb group by weekday,month,quarter,year
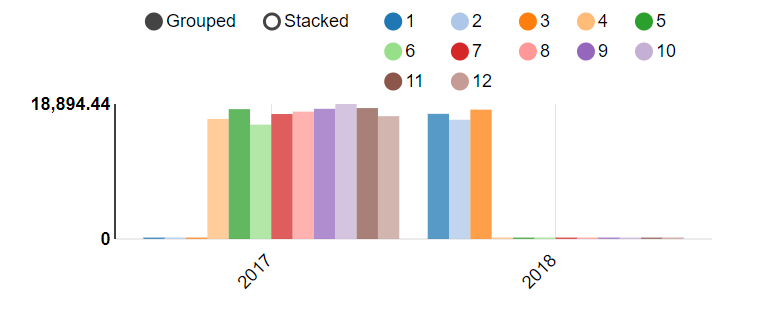
2.5 按时间段计算总收入(需要清理数据)
这里的时间格式不统一,有24时记时,也有12时记时,需要对数据进行整合。
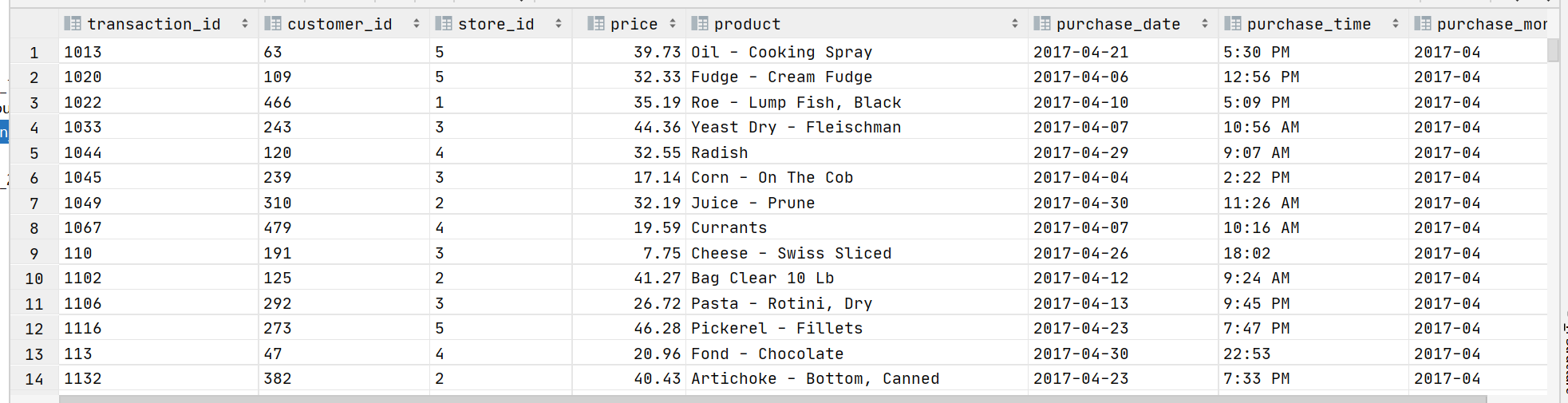
解体思路:
按时间段计算总收入
early morning(5:00-8:00)
morning(8:00-11:00)
noon(11:00-13:00)
afternoon(13:00-18:00)
evening(18:00-22:00)
night(22:00-5:00)
1. 将12时转换为24时
select
if(purchase_time like '%M',
from_unixtime(unix_timestamp(purchase_time,'hh:mm aa'),'HH:mm'),
purchase_time) as time_form
from transaction_details;
2.分段进行分析
with
basetb as (
select
price,purchase_time,
if(purchase_time like '%M',
from_unixtime(unix_timestamp(purchase_time,'hh:mm aa'),'HH:mm'),
purchase_time) as time_format
from transaction_details
),
basetb2 as (
select price,purchase_time,time_format,
cast(split(time_format,':')[0] as decimal(4,2)) +
cast(split(time_format,':')[1] as decimal(4,2))/60 as `purchase_time_num`
from basetb
)
select price,purchase_time,
if(purchase_time_num>5 and purchase_time_num<=8,'early morning',
if(purchase_time_num>8 and purchase_time_num<=11,'morning',
if(purchase_time_num>11 and purchase_time_num<=13,'noon',
if(purchase_time_num>13 and purchase_time_num<=18,'afternoon',
if(purchase_time_num>18 and purchase_time_num<=22,'evening',
'night'))))) as time_bucket
from basetb2;
3. 对数据进行分析
select time_bucket1,sum(price) from basetb3
group by time_bucket1;
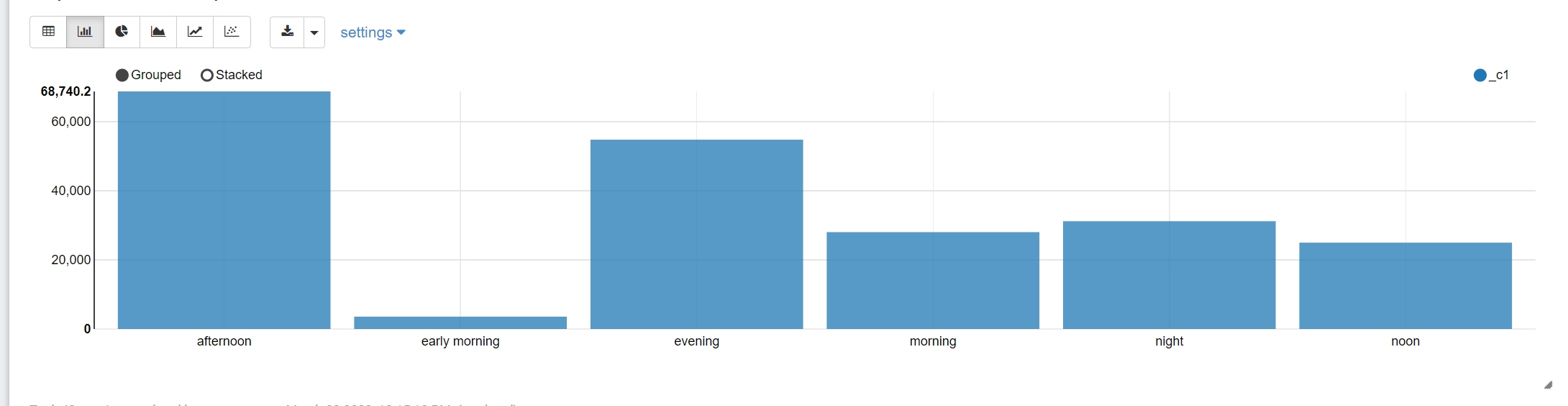
2.6 按时间段计算平均消费
select time_bucket1,avg(price) from basetb3
group by time_bucket1;
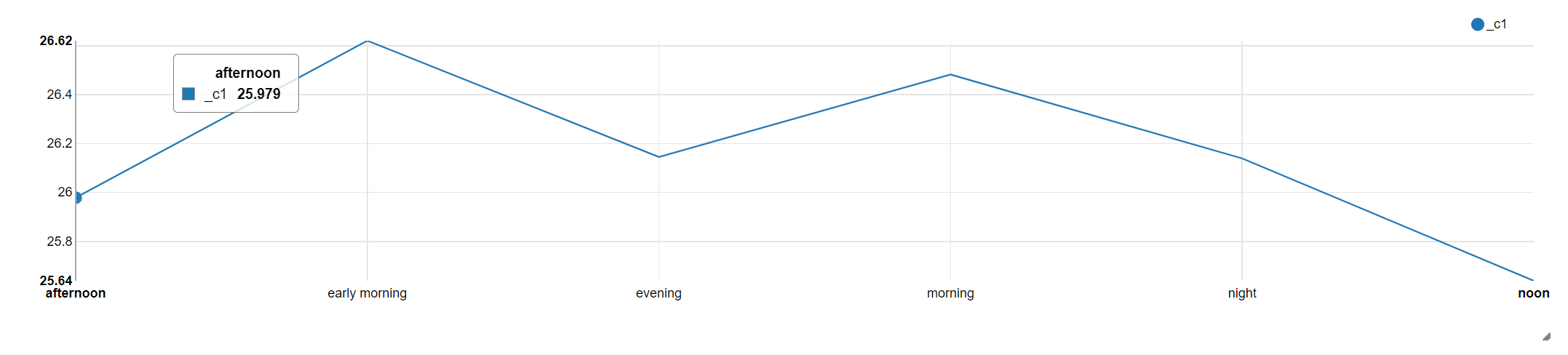
2.7 按工作日计算平均消费
select
dayofweek(purchase_date),avg(price)
from transaction_details
where dayofweek(purchase_date) between 1 and 5
group by dayofweek(purchase_date)
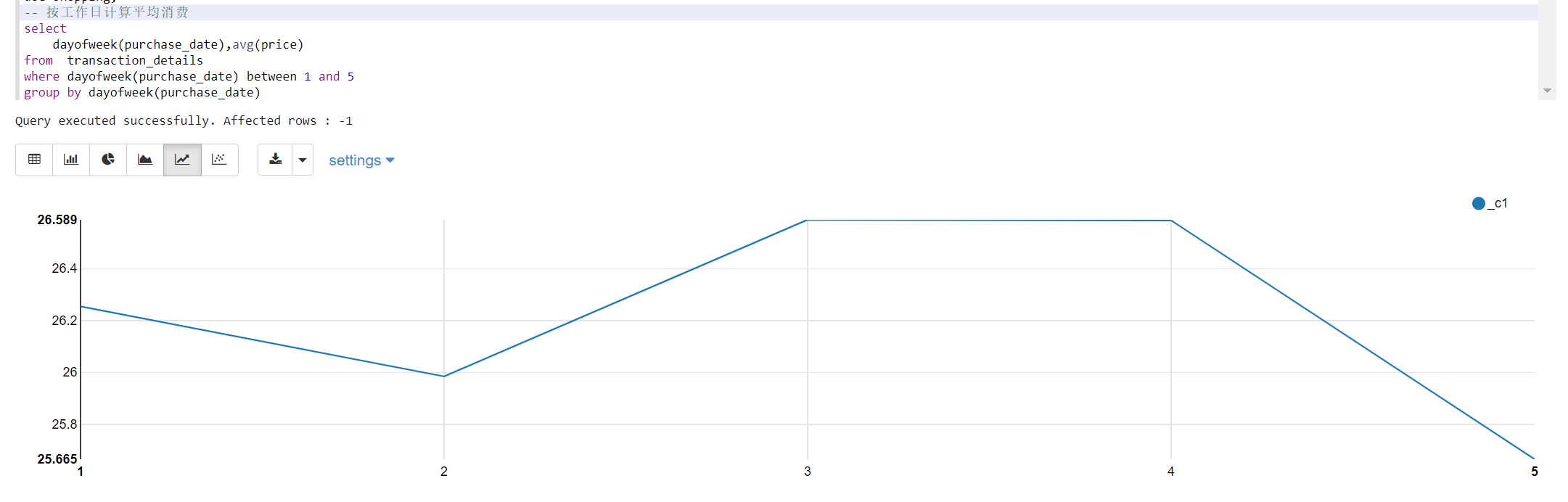
2.8 计算年、月、日的交易总数
select
count(1) over(partition by year(purchase_date)) year,
count(1) over(partition by year(purchase_date),month(purchase_date)) month,
count(1) over(partition by year(purchase_date),month(purchase_date),day(purchase_date)) day
from transaction_details
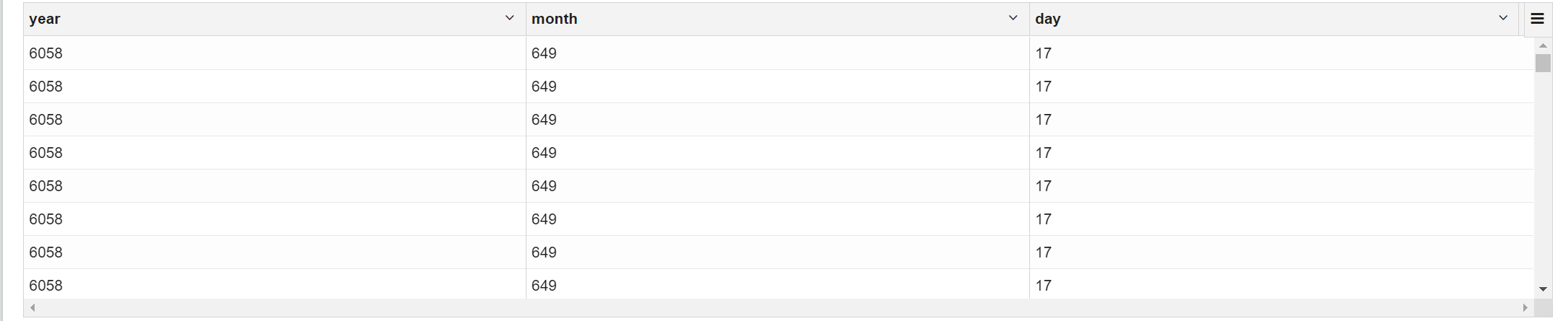
2.9 找出交易量最大的10个客户
select
customer_id,count(1) a
from transaction_details group by customer_id order by a desc limit 10
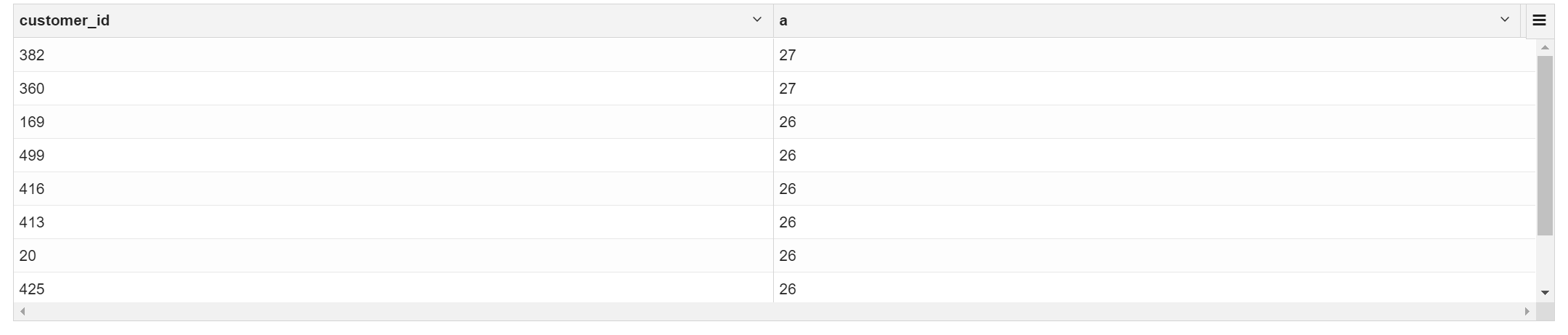
2.10 找出消费最多的前10位顾客
select
customer_id,sum(price) a
from transaction_details group by customer_id order by a desc limit 10
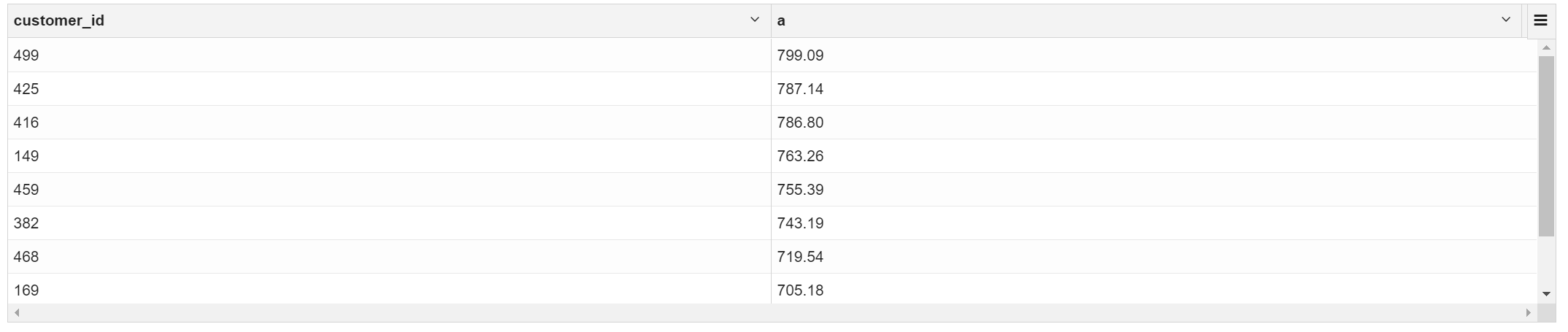
2.11 统计该期间交易数量最少的用户
select
customer_id,count(1) a
from transaction_details group by customer_id order by a asc limit 5
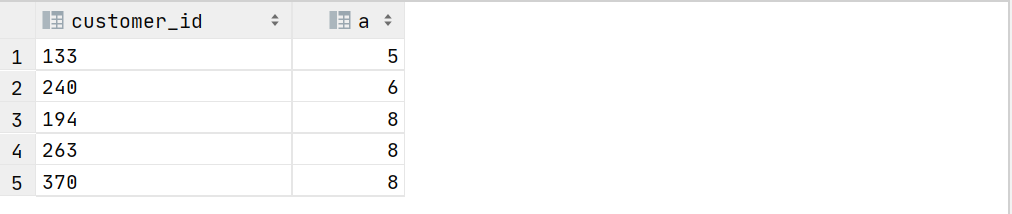
2.12 计算每个季度的独立客户总数
1. 每个季度的用户总数
select count(1),year(purchase_date),quarter(purchase_date)
from transaction_details group by year(purchase_date),quarter(purchase_date)
2. 去除掉重复的用户
select count(1),year(purchase_date),quarter(purchase_date)
from
(
select row_number() over (partition by customer_id,year(purchase_date),quarter(purchase_date)) rm1 ,* from transaction_details
) t1
where t1.rm1=1 group by year(purchase_date),quarter(purchase_date)



2.13 计算每周的独立客户总数
select count(1),weekofyear(purchase_date)
from
(
select row_number() over (partition by customer_id,year(purchase_date),quarter(purchase_date)) rm1 ,* from transaction_details
) t1
where t1.rm1=1 group by weekofyear(purchase_date)
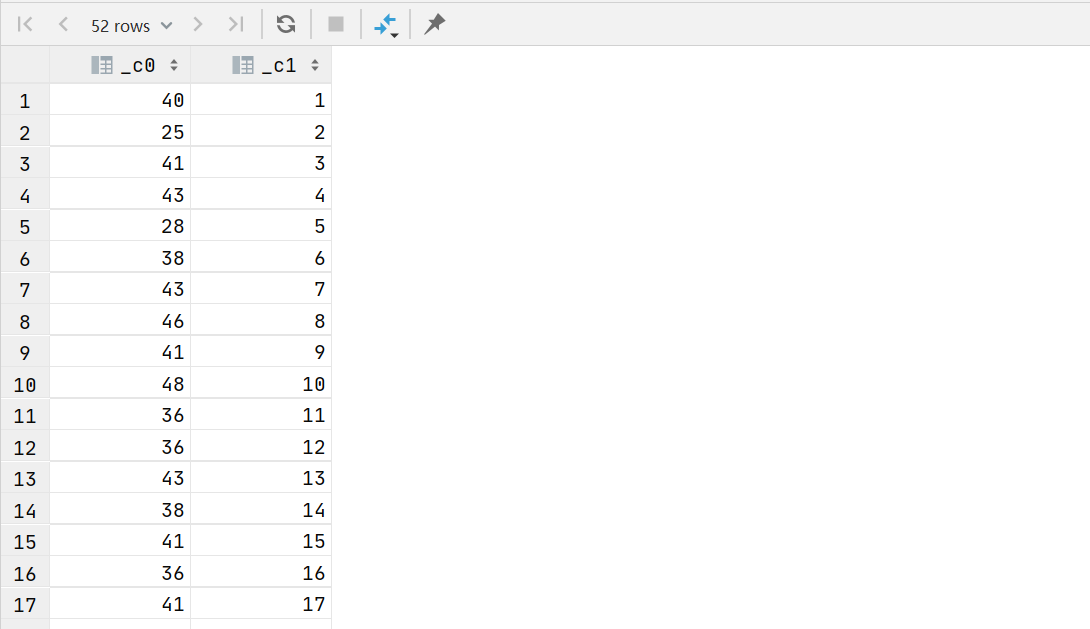
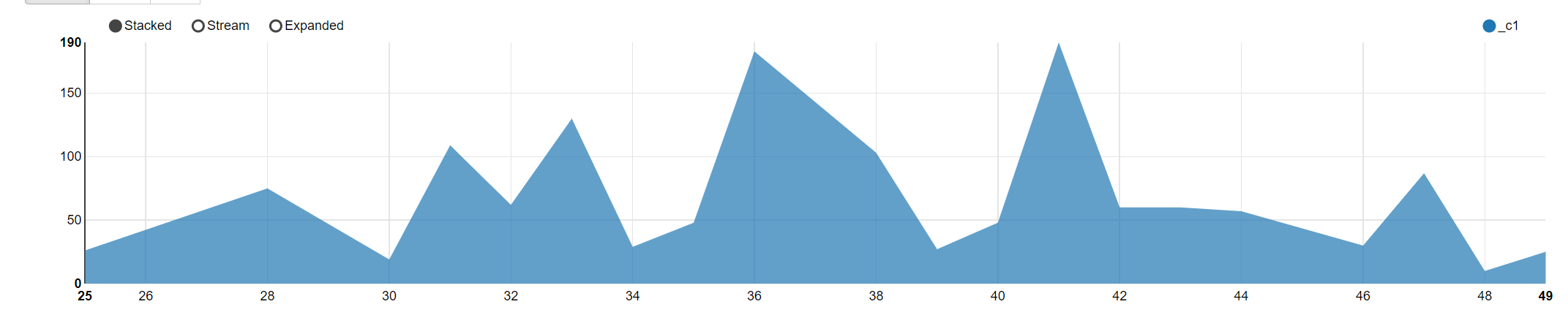
2.14 计算整个活动客户平均花费的最大值
select customer_id,max(tb.avg1) from
(select customer_id,avg(price) avg1
from transaction_details group by customer_id) tb;

2.15 统计每月花费最多的客户
with t1 as ( select
purchase_month,customer_id,sum(price) sp
from transaction_details group by purchase_month,customer_id )
select purchase_month,max(sp) from t1 group by purchase_month
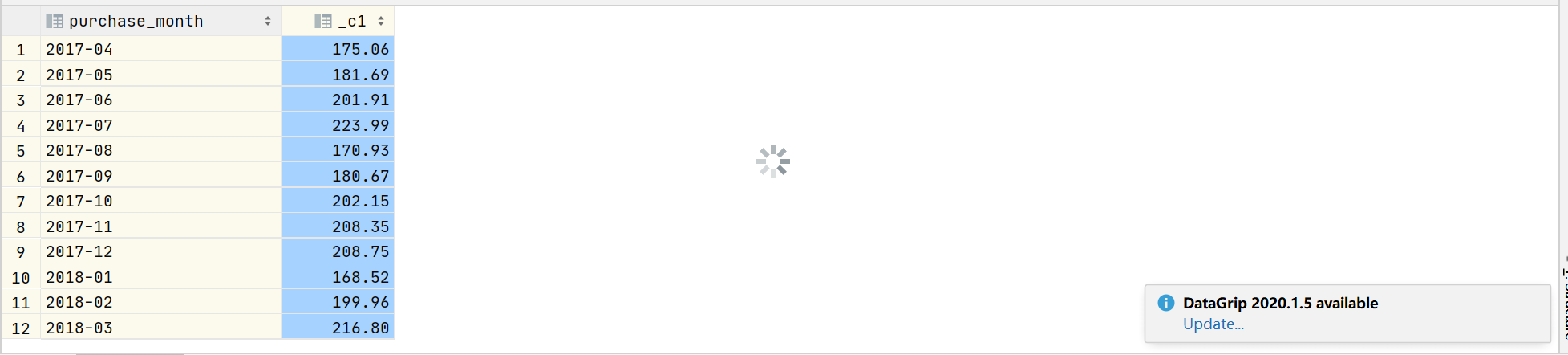
2.16 统计每月访问次数最多的客户
with t1 as (
select
purchase_month,customer_id,count(price) cp,
rank() over (partition by purchase_month order by count(price) desc) rk
from transaction_details group by purchase_month,customer_id
)
select purchase_month,customer_id,cp from t1 where rk=1;
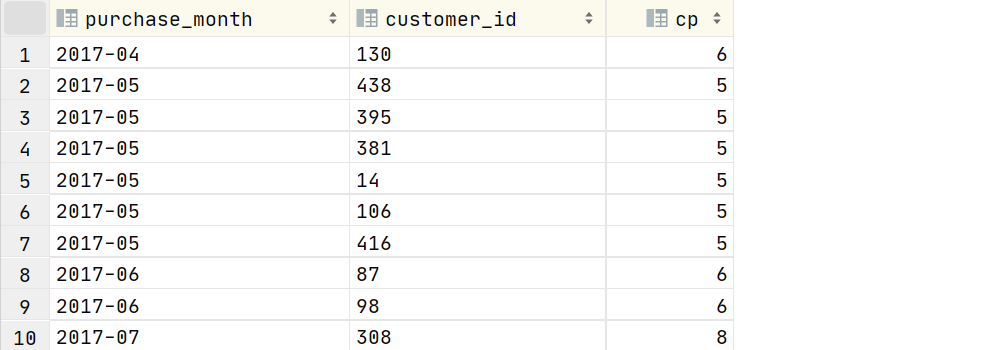
2.17 按总价找出最受欢迎的5种产品
select product,sum(price) sp from transaction_details
group by product
order by sp desc limit 5;

2.18根据购买频率找出最畅销的5种产品
select product,count(price) cp from transaction_details
group by product
order by cp desc limit 5;

2.19根据客户数量找出最受欢迎的5种产品
select product,count(distinct customer_id) cp from transaction_details
group by product
order by cp desc limit 5;

2.20 验证前5个details
Store分析
3.1 按客流量找出最受欢迎的商店
select
store_name,count(distinct customer_id) as visit
from transaction_details td join ext_store_details sd on td.store_id=sd.store_id
group by store_name order by visit
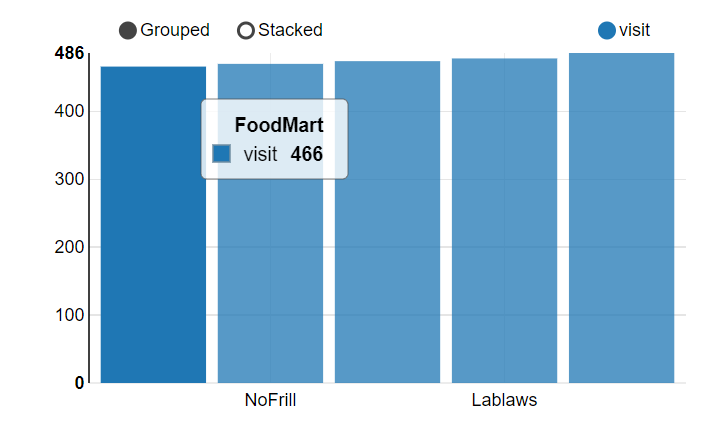
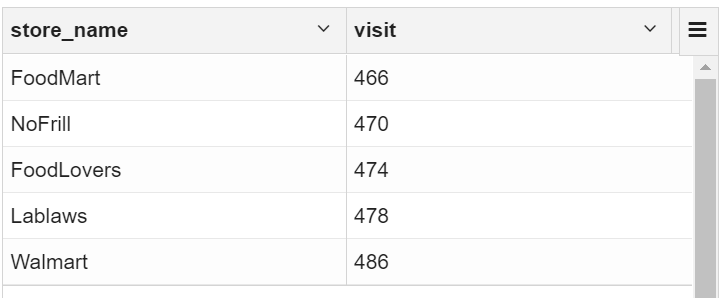
3.2 根据顾客消费价格找出最受欢迎的商店
select
store_name,sum(price) as visit
from transaction_details td join ext_store_details sd on td.store_id=sd.store_id
group by store_name order by visit

3.3 根据顾客交易情况找出最受欢迎的商店
select
store_name,count(customer_id) as c
from transaction_details td join ext_store_details sd on td.store_id=sd.store_id
group by store_name order by c desc
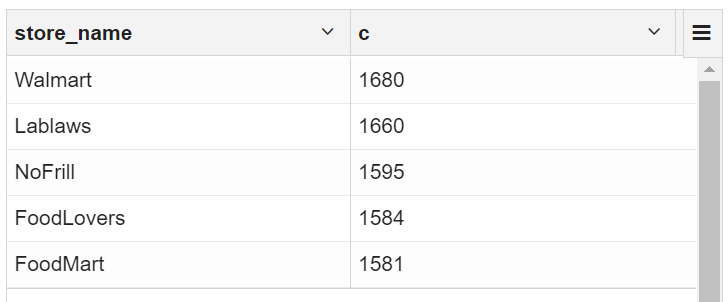
3.4 根据商店和唯一的顾客id获取最受欢迎的产品
with t1 as(
select
store_name,product,count(distinct customer_id) cdc
from transaction_details td join ext_store_details sd on td.store_id=sd.store_id
group by store_name,product having cdc>3 order by cdc desc
),
t2 as(
select store_name,product,cdc,
row_number() over (partition by store_name order by cdc desc ) rn from t1
)
select store_name,product,cdc from t2 where rn=1;
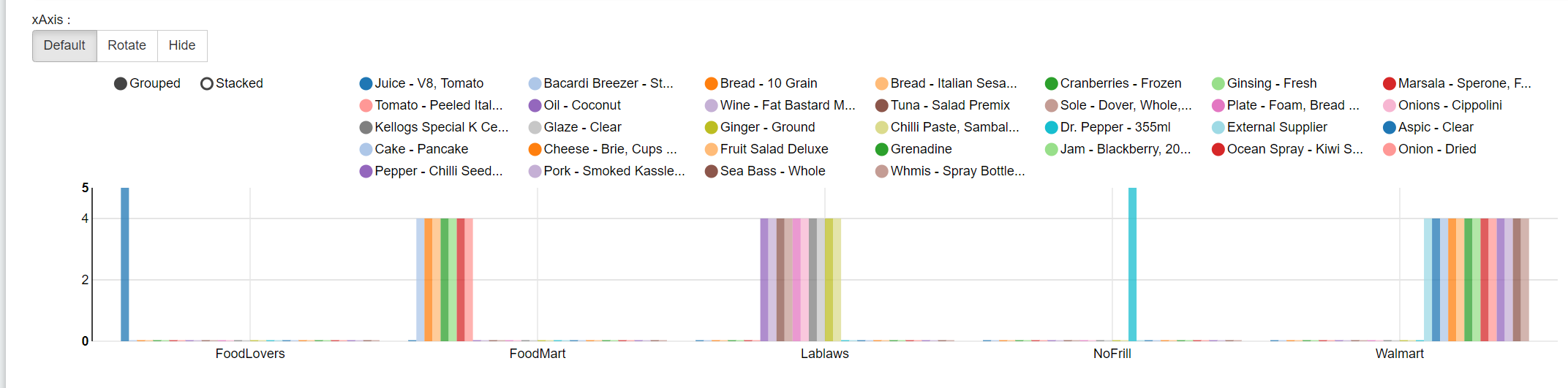
3.5 获取每个商店的员工与顾客比
with
t1 as ( select count(1) c1,store_id
from transaction_details td
group by td.store_id )
select concat(substring(cast(esd.employee_number/t1.c1 as decimal(9,8))*100.0,0,4),'%')
,t1.store_id,esd.store_name from t1
join shopping.ext_store_details esd on t1.store_id=esd.store_id

3.6 按年和月计算每家店的收入
with t1 as (
select year(purchase_date) year, month(purchase_date) month, store_id si, sum(price) sp
from transaction_details
group by year(purchase_date), month(purchase_date), store_id
)
select esd.store_id,esd.store_name,t1.year,t1.month,t1.sp from t1
join shopping.ext_store_details esd on t1.si=esd.store_id
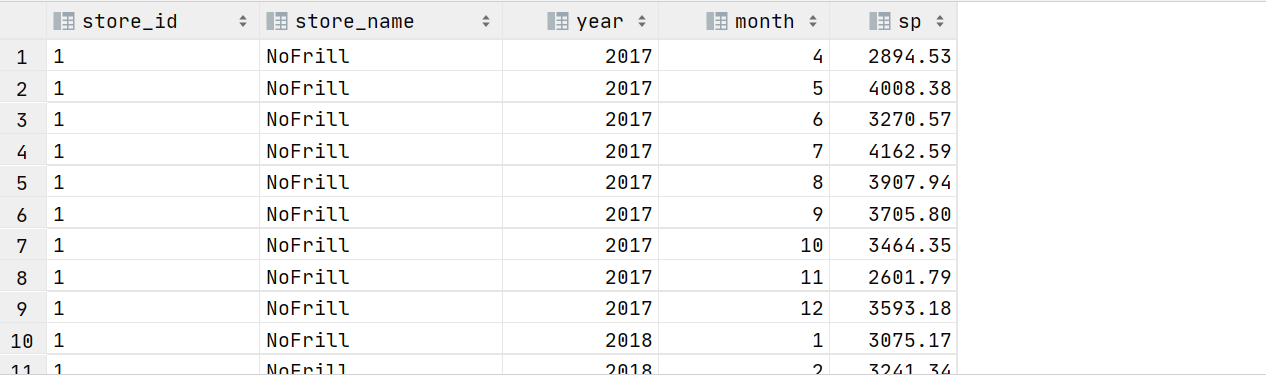
3.7 按店铺制作总收益饼图
select
store_id,sum(price)
from transaction_details group by store_id
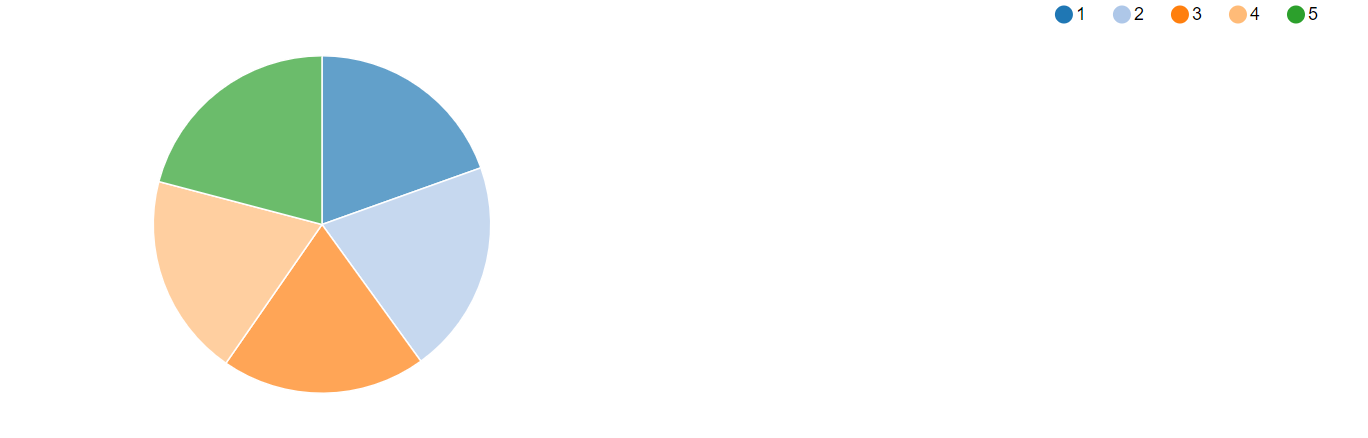
3.8 找出每个商店最繁忙的时间段
with t1 as(
select
price,purchase_time,store_id,
if(purchase_time like '%M',
from_unixtime(unix_timestamp(purchase_time,'hh:mm aa'),'HH:mm'),
from_unixtime(unix_timestamp(purchase_time,'HH:mm'),'HH:mm')) time1
from transaction_details
),
t2 as (
select
price,purchase_time,store_id,
time1,
cast(split(time1,':')[0] as int)+cast(split(time1,':')[1] as decimal(4,2))/60 time2
from t1
),
t3 as (
select
price,purchase_time,store_id,time1,
case when time2>5 and time2<=8 then 'early morning'
when time2<=11 and time2>8 then 'morning'
when time2<=13 and time2>11 then 'noon'
when time2<=18 and time2>13 then 'afternoon'
when time2<=22 and time2>18 then 'evening'
else 'night' end time3
from t2
)select store_id,time3,count(1) sp from t3 group by time3,store_id order by sp desc;
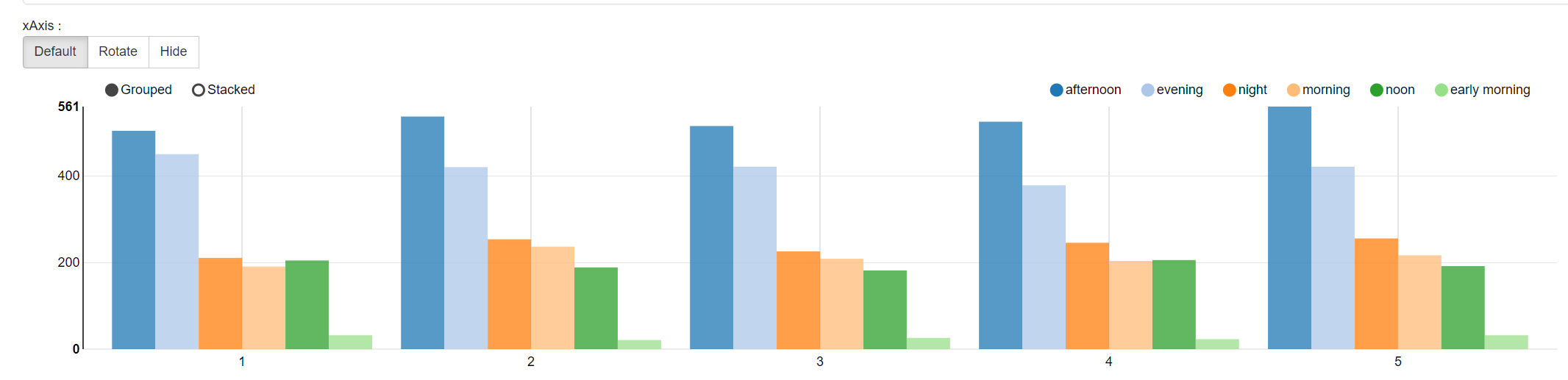
3.9 找出每家店的忠实顾客
with t1 as ( select
row_number() over (partition by customer_id,store_id) ro,*
from transaction_details )
select customer_id,store_id,count(1) c from t1
group by customer_id,store_id having c>=8
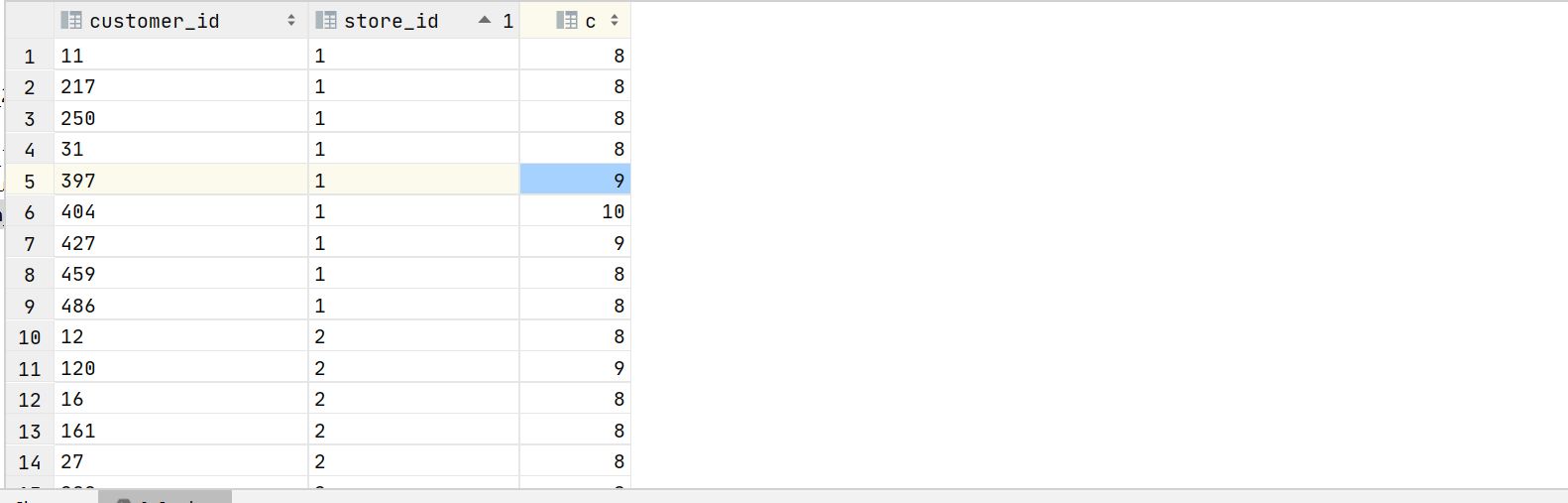
3.10 根据每位员工的最高收入找出明星商店
select distinct td.store_id,esd.store_name,esd.employee_number,t1.sp/esd.employee_number rk from transaction_details td
join ext_store_details esd on td.store_id = esd.store_id
join (select store_id,sum(price) sp from transaction_details group by store_id) t1 on td.store_id=t1.store_id
order by rk desc

Review分析
4.1 在ext_store_review中找出存在冲突的交易映射关系
with basetb as(
select row_number() over (partition by transaction_id) as row_number1,* from ext_review_details
)
select row_number1,a.transaction_id,a.store_id,b.store_id,a.review_score,b.review_score from basetb a
join ext_review_details b on a.transaction_id=b.transaction_id
where row_number1 >1
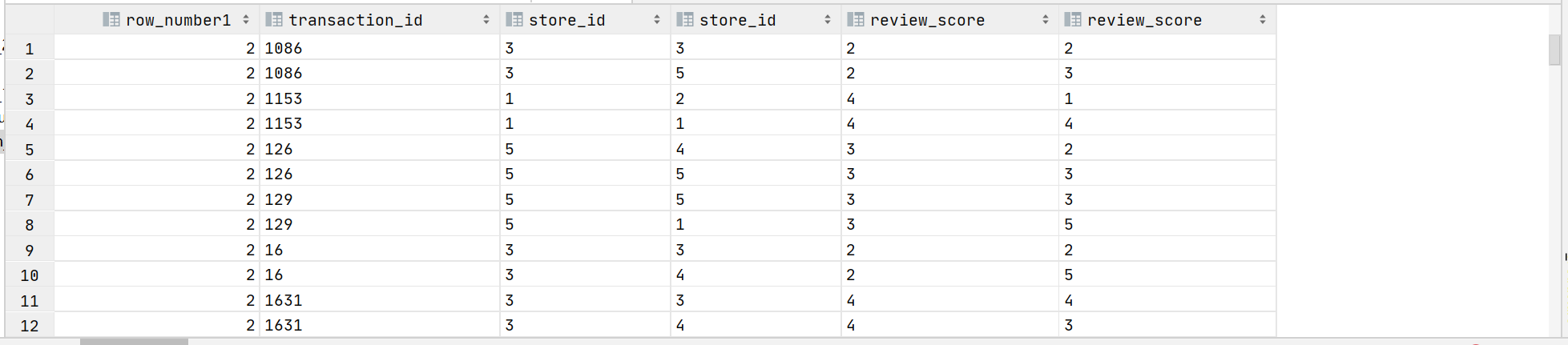
4.2 了解客户评价的覆盖率
with t1 as (
select count(1) c1 from ext_review_details where review_score <> ''
),
t2 as (
select count(1) c2 from ext_review_details where review_score = ''
)
select concat(cast((c1-c2)/c1*100 as decimal(4,2)),'%') from t1 join t2
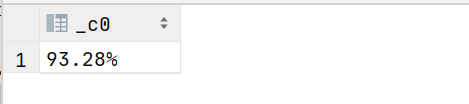
4.3 根据评分了解客户的分布情况
select c.country,r.review_score,count(price) from ext_review_details r
join transaction_details t on r.transaction_id=t.transaction_id
join ext_customer_details c on t.customer_id = c.customer_id
group by review_score,c.country;
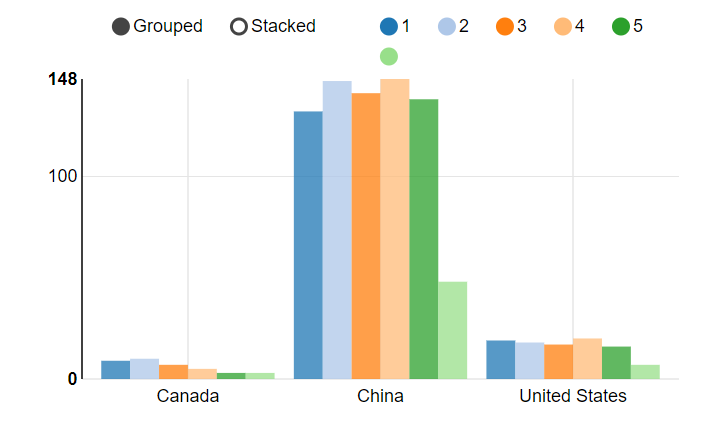
4.4 根据交易了解客户的分布情况
select
country,sum(price),count(price)
from transaction_details td
join ext_customer_details cd on td.customer_id = cd.customer_id
group by cd.country

4.5 客户给出的最佳评价是否总是同一家门店