功能
把depth维的数据移到space上,与spacetodepth刚好是相反的操作,depth对应channel,space对应height和width,而该操作是把depth上的数据分给height和width上,
所以对应有一个参数block_size,要求原tensor的depth是block_size的block_size^2倍
这样,新的tensor
dst_w = src_w * block_size
dst_h = src_h * block_size
dst_depth = src_depth / (block_size * block_size)
#tensorflow原型如下
tf.nn.depth_to_space(
input, block_size, data_format='NHWC', name=None
)
因为这里严格区分了C与H/W各维度,如果数据格式不是nhwc,需要指定data_format
官方示例
[1,1,1,12] -> [1,2,2,1]
t = tf.range(12)
t = tf.reshape(t,[1,1,1,12])
print(t)
############
### tf.Tensor([[[[ 0 1 2 3 4 5 6 7 8 9 10 11]]]], shape=(1, 1, 1, 12), dtype=int32)
############
t = tf.nn.depth_to_space(t, 2)
print(t)
############
tf.Tensor(
[[[[ 0 1 2]
[ 3 4 5]]
[[ 6 7 8]
[ 9 10 11]]]], shape=(1, 2, 2, 3), dtype=int32)
###########
[1,2,2,4] -> [1,4,4,1]
t = tf.range(16)
t = tf.reshape(t,[1,2,2,4])
print(t)
########################
tf.Tensor(
[[[[ 0 1 2 3]
[ 4 5 6 7]]
[[ 8 9 10 11]
[12 13 14 15]]]], shape=(1, 2, 2, 4), dtype=int32)
########################
t = tf.nn.depth_to_space(t, 2)
print(t)
########################
tf.Tensor(
[[[[ 0]
[ 1]
[ 4]
[ 5]]
[[ 2]
[ 3]
[ 6]
[ 7]]
[[ 8]
[ 9]
[12]
[13]]
[[10]
[11]
[14]
[15]]]], shape=(1, 4, 4, 1), dtype=int32)
#######################
3、更复杂点的
t = tf.range(72)
t = tf.reshape(t,[1,2,3,12])
print(t)
####################################
tf.Tensor(
[[[[ 0 1 2 3 4 5 6 7 8 9 10 11]
[12 13 14 15 16 17 18 19 20 21 22 23]
[24 25 26 27 28 29 30 31 32 33 34 35]]
[[36 37 38 39 40 41 42 43 44 45 46 47]
[48 49 50 51 52 53 54 55 56 57 58 59]
[60 61 62 63 64 65 66 67 68 69 70 71]]]], shape=(1, 2, 3, 12), dtype=int32)
####################################
该tensor表示如下:
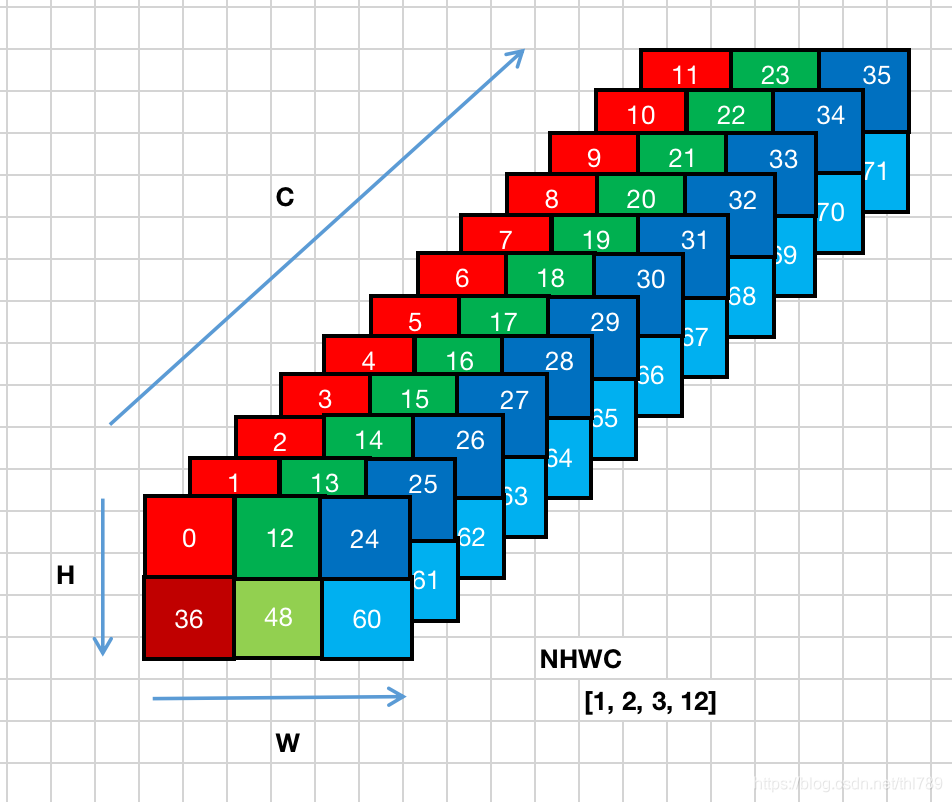
处理过程就是下面这样
1、按照width->height方向选择channel
2、对一个channel里的数据,用reshape操作改为 [in_batch, block_size, block_size, in_channel / (block_size*block_size)] (原来应该是[in_batch,1,1,in_channel])
3、对reshape后的数据,按照width->height的顺序再拼起来
比如上面的例3第一个channel应该是例1中 0~11
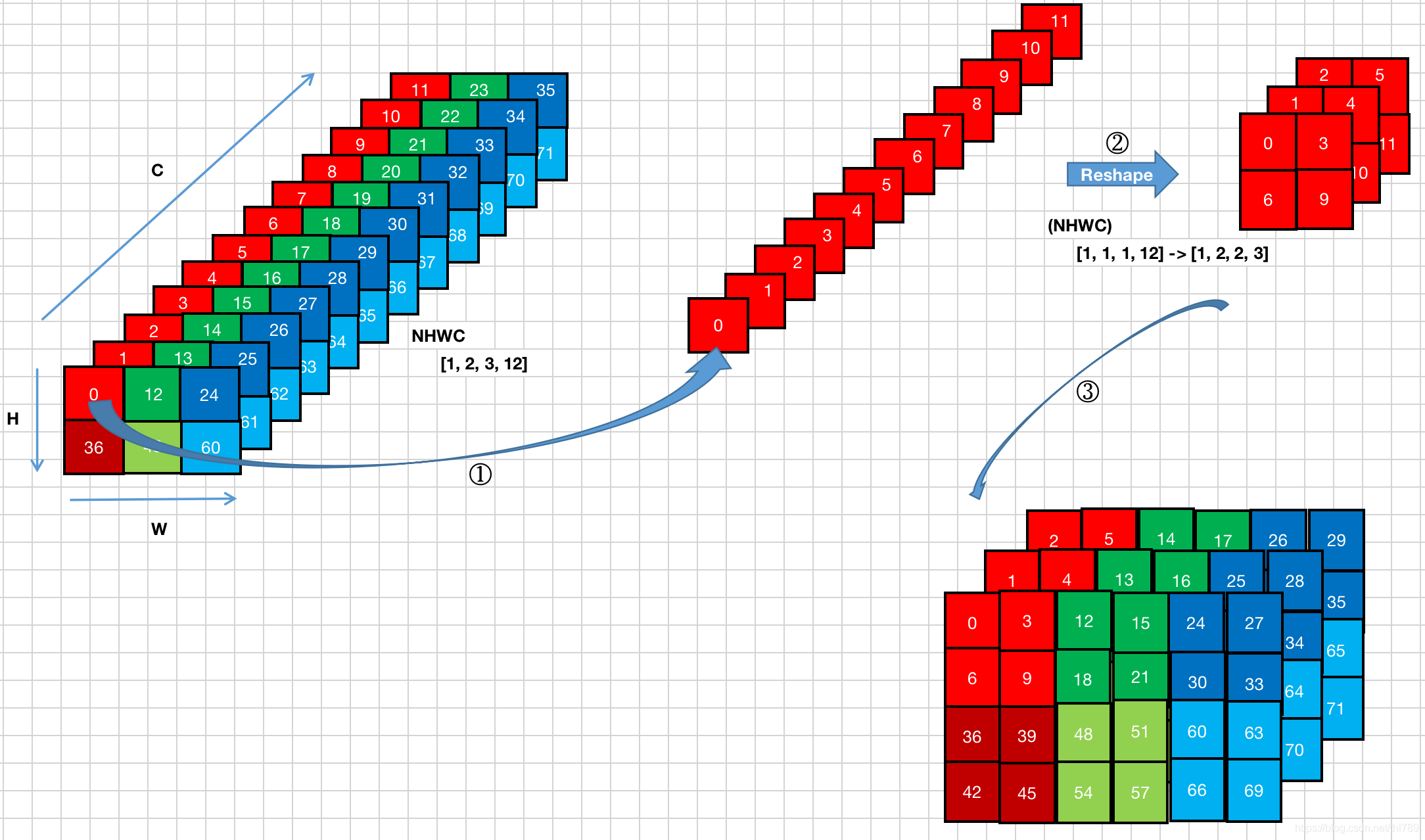
其他channel依葫芦画瓢最后连接起来就行