模型训练过程中常需边训练边做validation或在训练完的模型需要做测试,通常的做法当然是先创建model实例然后掉用load_state_dict()装载训练出来的权重到model里再调用model.eval()把模型转为测试模式,这样写对于训练完专门做测试时当然是比较合适的,但是对于边训练边做validation使用这种方式就需要写一堆代码,如果能使用copy.deepcopy()直接深度拷贝训练中的model用来做validation显然是比较简洁的写法,但是由于copy.deepcopy()的限制,写model里代码时如果没注意,调用copy.deepcopy(model)时可能就会遇到这个错误:Only Tensors created explicitly by the user (graph leaves) support the deepcopy protocol at the moment,详细错误信息如下:
File "/usr/local/lib/python3.6/site-packages/prc/framework/model/validation.py", line 147, in init_val_model
val_model = copy.deepcopy(model)
File "/usr/lib64/python3.6/copy.py", line 180, in deepcopy
y = _reconstruct(x, memo, *rv)
File "/usr/lib64/python3.6/copy.py", line 280, in _reconstruct
state = deepcopy(state, memo)
File "/usr/lib64/python3.6/copy.py", line 150, in deepcopy
y = copier(x, memo)
File "/usr/lib64/python3.6/copy.py", line 240, in _deepcopy_dict
y[deepcopy(key, memo)] = deepcopy(value, memo)
File "/usr/lib64/python3.6/copy.py", line 180, in deepcopy
y = _reconstruct(x, memo, *rv)
File "/usr/lib64/python3.6/copy.py", line 306, in _reconstruct
value = deepcopy(value, memo)
File "/usr/lib64/python3.6/copy.py", line 180, in deepcopy
y = _reconstruct(x, memo, *rv)
File "/usr/lib64/python3.6/copy.py", line 280, in _reconstruct
state = deepcopy(state, memo)
File "/usr/lib64/python3.6/copy.py", line 150, in deepcopy
y = copier(x, memo)
File "/usr/lib64/python3.6/copy.py", line 240, in _deepcopy_dict
y[deepcopy(key, memo)] = deepcopy(value, memo)
File "/usr/lib64/python3.6/copy.py", line 180, in deepcopy
y = _reconstruct(x, memo, *rv)
File "/usr/lib64/python3.6/copy.py", line 306, in _reconstruct
value = deepcopy(value, memo)
File "/usr/lib64/python3.6/copy.py", line 180, in deepcopy
y = _reconstruct(x, memo, *rv)
File "/usr/lib64/python3.6/copy.py", line 280, in _reconstruct
state = deepcopy(state, memo)
File "/usr/lib64/python3.6/copy.py", line 150, in deepcopy
y = copier(x, memo)
File "/usr/lib64/python3.6/copy.py", line 240, in _deepcopy_dict
y[deepcopy(key, memo)] = deepcopy(value, memo)
File "/usr/lib64/python3.6/copy.py", line 161, in deepcopy
y = copier(memo)
File "/root/.local/lib/python3.6/site-packages/torch/_tensor.py", line 55, in __deepcopy__
raise RuntimeError("Only Tensors created explicitly by the user "
RuntimeError: Only Tensors created explicitly by the user (graph leaves) support the deepcopy protocol at the moment
这个错误简单地说就是copy.deepcopy()不支持拷贝requires_grad=True的Tensor(在网络中一般是非叶子结点Tensor, grad_fn不为None),开始以为真的哪个地方Tensor的requires_grad没有按要求设置,熬了几个夜去检查调试网络代码没发现什么线索很郁闷,后来想既然是copy.deepcopy()里报错的,源码也有那就去它里面debug看是拷贝网络的那部分时抛出的Exception吧,折腾了一阵发现里面这个地方加breakpoint比较合适:
if dictiter is not None:
if deep:
for key, value in dictiter:
key = deepcopy(key, memo)
value = deepcopy(value, memo)
y[key] = value
else:
for key, value in dictiter:
y[key] = value
我这个网络的结构是使用的python dict方式定义的,运行时使用注册机制动态创建出来的,既然是dict,这里的key和value就是对应配置文件里的定义网络每层结构的dict的key和value,在这里加bp可以比较清楚地跟踪看到是在哪个地方导致的抛出Exception,结果发现原因是因为有个实现分割功能的head类的内部有个成员变量保存了这层的输出结果Tensor用于后面计算loss,模型每层的输出数据Tensor自然是requires_grad=True,把这个成员变量去掉,改成forward()输出结果,然后在网络的主类里接收它并传入计算Loss的函数,然后deepcopy(model)就不报上面的错了!
另外,显式创建一个Tensor时指定requires_grad=True(默认是False)并不会导致copy.deepcopy()报错,不管这个Tensor是在cpu上还是gpu上,关键是用户自己创建的Tensor是叶子结点Tensor,它的grad_fn是None,在这个Tensor上做切片或者加载到gpu上等操作得到的新的Tensor就不是叶子结点了,pytorch认为requires_grad=Trued的Tensor经过运算得到新的Tensor是需要求导的会自动加上grad_fn而不管这个Tensor是不是网络的一部分,这时再使用copy.deepcopy()深度拷贝新的Tensor时会抛出上面的错误,看完下面的示例就知道了:
>>> t = torch.tensor([1,2,3.5],dtype=torch.float32, requires_grad=True, device='cuda:0')
>>> t
tensor([1.0000, 2.0000, 3.5000], device='cuda:0', requires_grad=True)
>>> x = copy.deepcopy(t)
>>> x
tensor([1.0000, 2.0000, 3.5000], device='cuda:0', requires_grad=True)
>>> t1 = t[:2]
>>> t1
tensor([1., 2.], device='cuda:0', grad_fn=<SliceBackward0>)
>>> x = copy.deepcopy(t1)
Traceback (most recent call last):
File "<stdin>", line 1, in <module>
File "/usr/local/python3.8/lib/python3.8/copy.py", line 153, in deepcopy
y = copier(memo)
File "/root/.local/lib/python3.8/site-packages/torch/_tensor.py", line 85, in __deepcopy__
raise RuntimeError("Only Tensors created explicitly by the user "
RuntimeError: Only Tensors created explicitly by the user (graph leaves) support the deepcopy protocol at the moment
>>> t = torch.tensor([1,2,3.5],dtype=torch.float32, requires_grad=True)
>>> t1 = t.cuda()
>>> t1
tensor([1.0000, 2.0000, 3.5000], device='cuda:0', grad_fn=<ToCopyBackward0>)
>>> x = copy.deepcopy(t1)
Traceback (most recent call last):
File "<stdin>", line 1, in <module>
File "/usr/local/python3.8/lib/python3.8/copy.py", line 153, in deepcopy
y = copier(memo)
File "/root/.local/lib/python3.8/site-packages/torch/_tensor.py", line 85, in __deepcopy__
raise RuntimeError("Only Tensors created explicitly by the user "
RuntimeError: Only Tensors created explicitly by the user (graph leaves) support the deepcopy protocol at the moment
>>> t = torch.tensor([1,2,3.5],dtype=torch.float32, requires_grad=False)
>>> t
tensor([1.0000, 2.0000, 3.5000])
>>> x = copy.deepcopy(t)
>>> x
tensor([1.0000, 2.0000, 3.5000])
>>> t1 = t[:2]
>>> t1
tensor([1., 2.])
>>> x = copy.deepcopy(t1)
为何deepcopy()不直接支持有梯度的Tensor,按理要支持复制一个当时的瞬间值应该也没问题,看到https://discuss.pytorch.org/t/copy-deepcopy-vs-clone/55022/10这里这个经常回答问题的胡子哥给了个猜测:
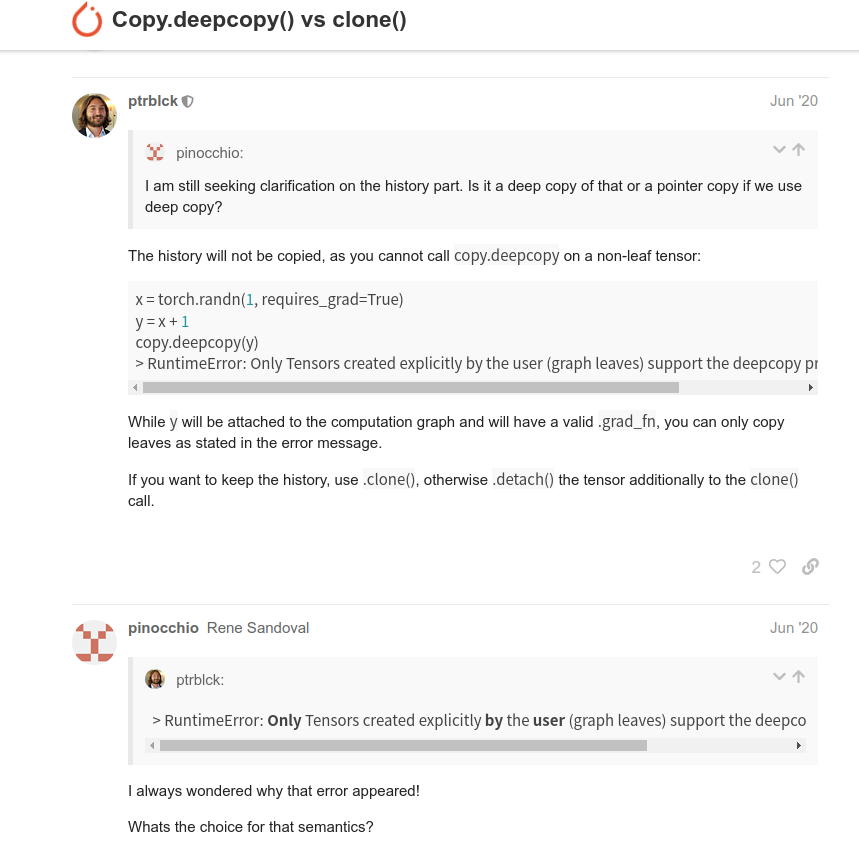
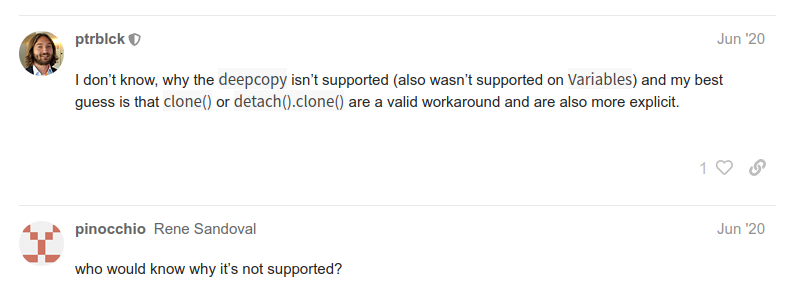