1.SGD(Stochastic Gradient Descent)
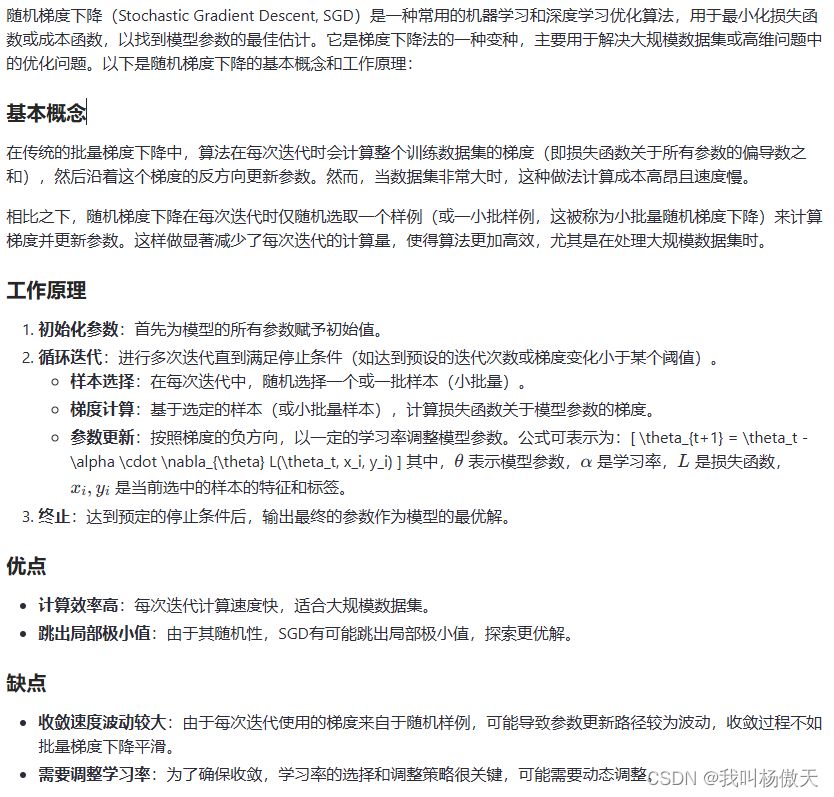

2.RMSprop(Root Mean Square Propagation)
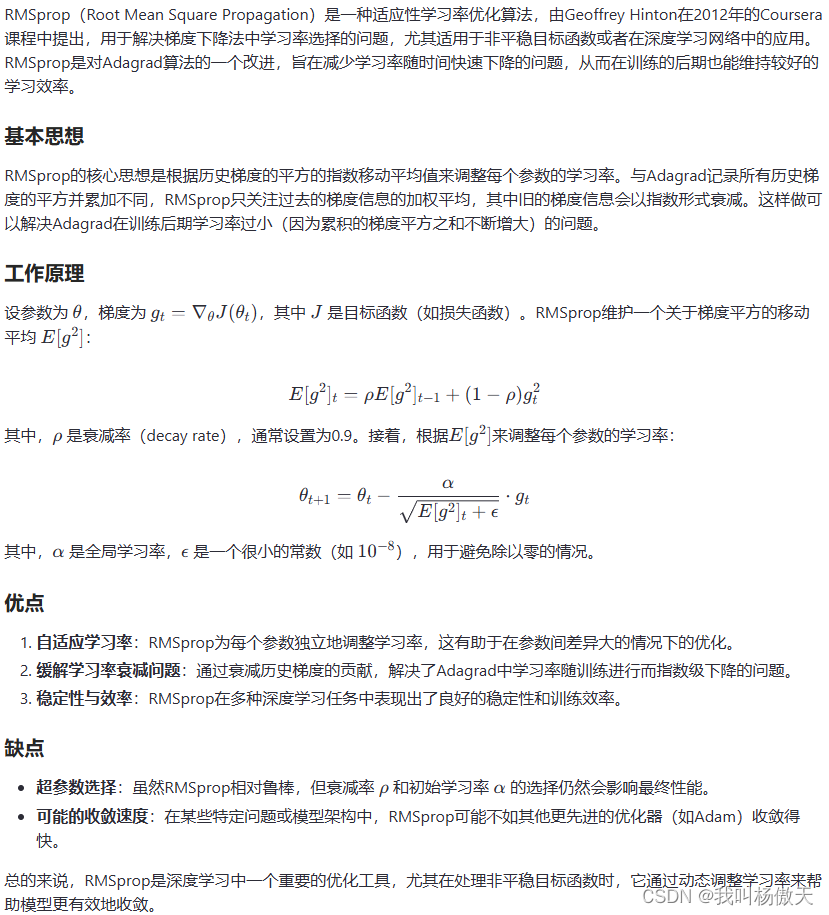
3.Adadelta
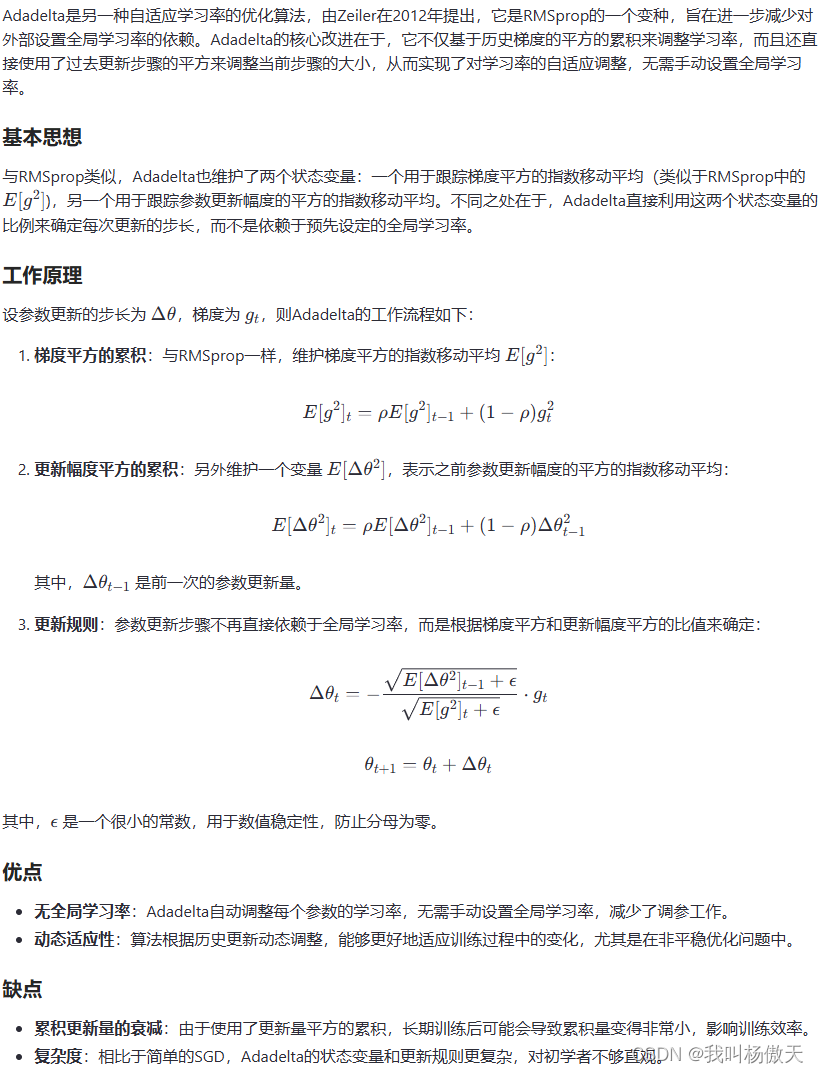
4.Adam(Adaptive Moment Estimation)
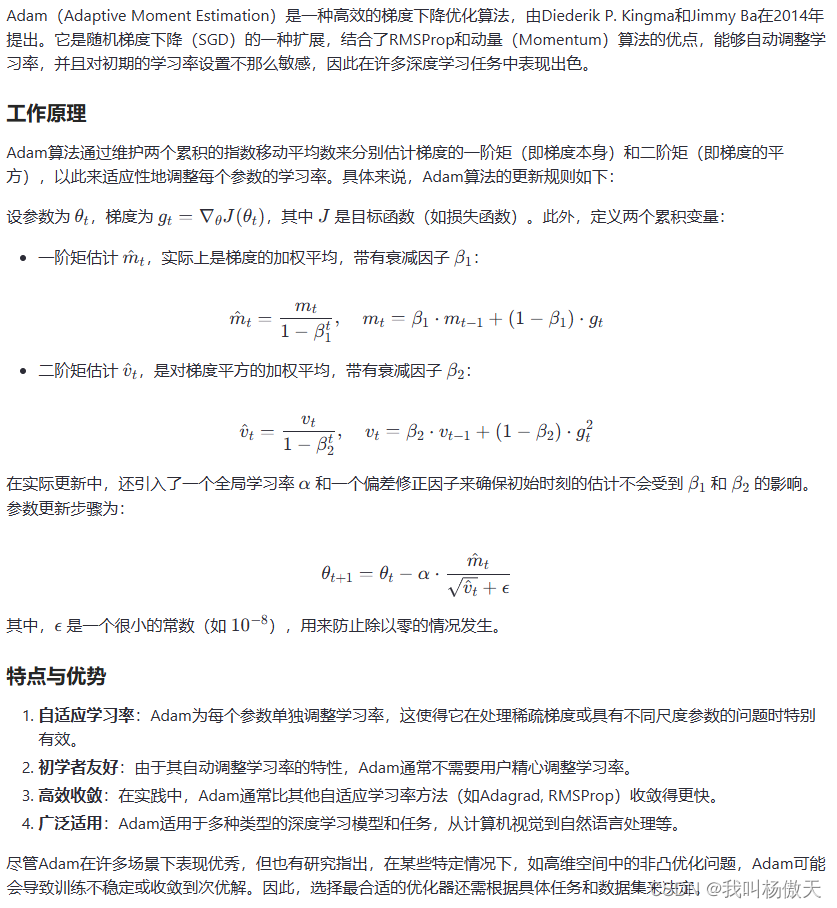
5.Nadam
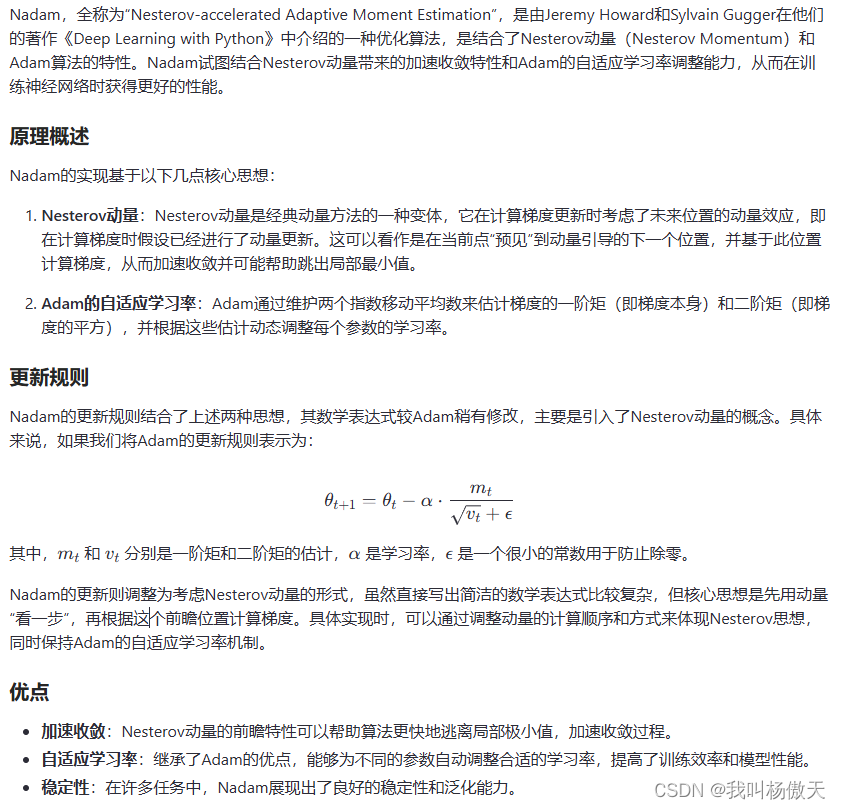
6.代码实现
from sklearn.compose import make_column_transformer
from sklearn.model_selection import GroupShuffleSplit, train_test_split
# !pip install --upgrade tensorflow
import tensorflow as tf
from tensorflow.keras import layers, callbacks
from tensorflow.keras.optimizers import SGD, Adam, Nadam,RMSprop, Adagrad, Adadelta
from tensorflow.keras.models import Model
from tensorflow.keras import backend as K
# 构建LSTM模型
model = tf.keras.Sequential()
model.add(layers.LSTM(units=64, input_shape=(timesteps, features_per_timestamp)))
model.add(layers.Dropout(0.5))
model.add(layers.Dense(32, activation='relu'))
model.add(layers.Dropout(0.5))
model.add(layers.Dense(forecast_horizon, activation=None)) # 回归任务通常不使用激活函数
#优化器
# 使用Adam优化器并设置学习率为0.001
optimizer = tf.keras.optimizers.Adam(learning_rate=0.001)
# 或者使用SGD优化器
# optimizer = SGD(learning_rate=0.01, momentum=0.9)
# 使用RMSprop优化器并设置学习率为0.001,rho值为0.9(衰减率)
# optimizer = RMSprop(learning_rate=0.001, rho=0.9)
# 使用Adadelta优化器,默认rho值为0.95(对应RMSprop中的rho),默认epsilon为1e-6(数值稳定参数)
# optimizer = Adadelta()
# 使用Nadam优化器,并设置学习率为0.002,beta_1和beta_2分别为默认的0.9和0.999
# optimizer = Nadam(learning_rate=0.002)
model.compile(
loss=loss,
sample_weight_mode = 'temporal',
metrics=[RMSE, MSE, MAE, R2, MAPE],
optimizer=optimizer
)