文章目录
- Introduction
- Data and Sample
- Download Data
- Clean Data
- Extract Estimation Unit and Set Global Variables
- Implement Brogaard Decomposition
- Estimate VAR Coefficients, Matrix B B B, ϵ t \epsilon_t ϵt, Σ e \Sigma_e Σe, and Σ ϵ \Sigma_\epsilon Σϵ
- Estimate 15-step cumulative structural IRFs θ r m \theta_{rm} θrm, θ x \theta_x θx, and θ r \theta_r θr
- Procedures for estimating θ \theta θ
- Obtain coefficient matrix A j ( j = 1 , 2 , . . . , 5 ) A_j(j=1,2,...,5) Aj(j=1,2,...,5)
- Calculate IRFs and cumulative un-orthogonalized Structural IRFs
- Extract θ \theta θ
- Calculate noise term
- Calculate the variance from each component
- Calculate variance contribution
- Pack codes
- Loop over sample
- Conclusion
Introduction
Having recognized the potential of the stock volatility decomposition method introduced by Brogaard et al. (2022, RFS) in my previous blog, I will show how to implement this method to empower your own research in this blog.
For readers with time constraints, the codes for implementing this variance decomposition method can be approched via this link.
As replicating Brogaard et al. (2022, RFS) requires some manipulations on the VAR estimation outputs, I took some time to figure out the theory and estimation of the reduced-form VAR coefficients, Impulse response functions (IRFs), structural IRFS, orthogonalized IRFs, and variance decomposition and summarized what I’ve got in a three-blog series about VAR.
In the first blog, I show the basic logics of VAR model with the simplest 2-variable, 1-lag VAR model. In the second blog, I show how to use var
and svar
commands to conveniently estimate the VAR model in Stata. In the third blog, I dig deeper, show the theoretical definitions and calculation formula of major outputs in VAR model, and manually calculate them in Stata to thoroughly uncover the black box of the VAR estimation.
For this blog, I will only focus on the paper-specific ideas. Readers who need more background information about VAR estimation can find clues in my three-blog series about VAR.
Data and Sample
The sample used by Brogaard et al. (2022, RFS) consists of all common stocks listed on the NYSE, AMEX, and NASDAQ spanning from 1960 to 2015. Estimation of the VAR model requires daily data on stock returns, market returns, and dollar-signed stock trading volumes.
The reduced-form VAR model below is estimated in stock-year level.
r
m
,
t
=
a
0
∗
+
∑
l
=
1
5
a
1
,
l
∗
r
m
,
t
−
l
+
∑
l
=
1
5
a
2
,
l
∗
x
t
−
l
+
∑
l
=
1
5
a
3
,
l
∗
r
t
−
l
+
e
r
m
,
t
x
t
=
b
0
∗
+
∑
l
=
1
5
b
1
,
l
∗
r
m
,
t
−
l
+
∑
l
=
1
5
b
2
,
l
∗
x
t
−
l
+
∑
l
=
1
5
b
3
,
l
∗
r
t
−
l
+
e
x
,
t
r
t
=
c
0
∗
+
∑
l
=
1
5
c
1
,
l
∗
r
m
,
t
−
l
+
∑
l
=
1
5
c
2
,
l
∗
x
t
−
l
+
∑
l
=
1
5
c
3
,
l
∗
r
t
−
l
+
e
r
,
t
(1)
\begin{aligned} &r_{m, t}=a_0^*+\sum_{l=1}^5 a_{1, l}^* r_{m, t-l}+\sum_{l=1}^5 a_{2, l}^* x_{t-l}+\sum_{l=1}^5 a_{3, l}^* r_{t-l}+e_{r_m, t} \\ &x_t=b_0^*+\sum_{l=1}^5 b_{1, l}^* r_{m, t-l}+\sum_{l=1}^5 b_{2, l}^* x_{t-l}+\sum_{l=1}^5 b_{3, l}^* r_{t-l}+e_{x, t} \\ &r_t=c_0^*+\sum_{l=1}^5 c_{1, l}^* r_{m, t-l}+\sum_{l=1}^5 c_{2, l}^* x_{t-l}+\sum_{l=1}^5 c_{3, l}^* r_{t-l}+e_{r, t} \end{aligned} \tag{1}
rm,t=a0∗+l=1∑5a1,l∗rm,t−l+l=1∑5a2,l∗xt−l+l=1∑5a3,l∗rt−l+erm,txt=b0∗+l=1∑5b1,l∗rm,t−l+l=1∑5b2,l∗xt−l+l=1∑5b3,l∗rt−l+ex,trt=c0∗+l=1∑5c1,l∗rm,t−l+l=1∑5c2,l∗xt−l+l=1∑5c3,l∗rt−l+er,t(1)
where
- r m , t r_{m,t} rm,t is the market return, the corresponding innovation ε r m , t \varepsilon_{r_{m,t}} εrm,t represents innovations in market-wide information
- x t x_t xt is the signed dollar volume of trading in the given stock, the corresponding innovation ε x , t \varepsilon_{x,t} εx,t represents innovations in firm-specific private information
- r t r_t rt is the stock return, the corresponding innovation ε r , t \varepsilon_{r,t} εr,t represents innovations in firm-specific public information
- the authors assume that ε r m , t , ε x , t , ε r , t \\{\varepsilon_{r_m, t}, \varepsilon_{x, t}, \varepsilon_{r, t}\\} εrm,t,εx,t,εr,t are contemporaneously uncorrelated
Download Data
To better serve my research purpose, in this blog I will implement the stock-year level variance decomposition for all common stocks listed on the NYSE, AMEX, and NASDAQ spanning from 2005 to 2021.
The SAS code is as follows. I first log in the WRDS server in SAS. Then I download the daily stock price, trading volume, return, and market return for all common stocks listed on the NYSE, AMEX, and NASDAQ - that’s exactly what the CRSP got. For ease of importing into Stata, I transfer all the downloaded sas
dataset into csv
format. As the daily CRSP data is too huge, I implement all the above procedures year by year.
libname home "C:\Users\xu-m\Documents\testVAR\rawdata2005-2021sas";
/* log in WRDS server */
%let wrds = wrds.wharton.upenn.edu 4016;
options comamid=TCP remote=WRDS;
signon username=xxx pwd=xxx;
run;
%signoff;
/* download daily stock price, trading volume, return, and market return from CRSP*/
rsubmit;
%macro downyear;
%do year = 2005 %to 2020;
%let firstdate = %sysfunc(mdy(1,1,&year));
%let lastdate = %sysfunc(mdy(12,31,&year));
%put &year;
proc sql;
create table sampleforvar&year as select distinct
cusip, date, ret, prc, vol, numtrd, shrout, hsiccd
from crsp.dsf
where date ge &firstdate and date le &lastdate;
quit;
proc sql;
create table sampleforvar&year as select a.*, b.*
from sampleforvar&year a, crsp.dsi b
where a.date=b.date;
quit;
proc download data=sampleforvar&year out=home.sampleforvar&year; run;
%end;
%mend;
%downyear;
endrsubmit;
/* transfer the downloaded sas dataset into csv format */
%macro expyear;
%do year = 2005 %to 2021;
%let outfile = %sysfunc(cat(C:\Users\xu-m\Documents\testVAR\rawdata2005-2021sas\sampleforvar, &year, .csv));
%let outfile = "%sysfunc(tranwrd(&outfile,%str( ),%str(%" %")))";
proc export data=home.sampleforvar&year outfile=&outfile dbms=csv replace; run;
%end;
%mend;
%expyear;
The output of this step is as follows. The raw data for each year is stored in csv
file named as sampleforvar
+ year
.
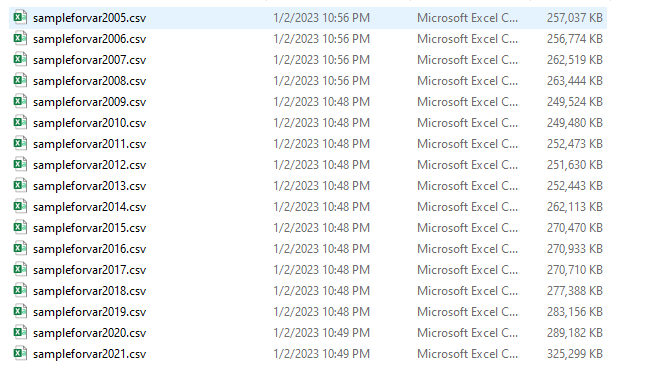
Clean Data
We have two tasks in this step.
- generate the 3 variables
rm
,x
, andr
for VAR estimation - set time series, which is the prerequisite for using the
svar
command
Following Brogaard et al. (2022, RFS), the 3 variables for VAR estimation is constructed as follows.
- I use Equal-Weighted Return (
ewretd
in CRSP) in basis points as market returnrm
- I use the daily Holding Period Return (
ret
in CRSP) in basis points as stock returnr
- I use the daily signed dollar volume in $ thousands as stock order flow
x
- The daily signed dollar volume is defined as the product of daily stock price (
prc
in CRSP), trading volume (vol
in CRSP), and the sign of the stocks’ daily return
- The daily signed dollar volume is defined as the product of daily stock price (
To mitigate the impacts of outliers, I winsorized all the above variables at the 5% and 95% levels.
I set the index of trading days within a given stock-year to tag the time series.
The Stata codes are as follows. Note that I use the cusip
and year
as identifiers. For the convenience of looping over stocks, I generate a unique number cusipcode
for each stock.
The output in this step is the yearly dataset named as sampledata
+year
that is ready for the implementation of the VAR estimation in stock-year level.
* import data
cd C:\Users\xu-m\Documents\testVAR\rawdata2005-2021sas
cap program drop cleanforbrogaard
cap program define cleanforbrogaard
import delimited "sampleforvar`1'.csv",clear
destring ret, force replace
drop if ret==.|prc<0|vol<0
g r = ret*10000
g prcsign = cond(ret>0,1,-1)
g x=vol*prc*prcsign/1000
g rm = ewretd*10000
winsor2 rm x r, cuts(5 95) replace
encode cusip, g(cusipcode)
sort cusipcode date
by cusipcode: g index=_n
xtset cusipcode index
g year = floor(date[1]/10000)
keep cusip cusipcode year index rm x r
save sampledata`1',replace
end
forvalues j = 2005/2021{
cleanforbrogaard `j'
}
Extract Estimation Unit and Set Global Variables
As the VAR estimation is implemented in stock-year level, we need firstly extract the sample for each stock and year with the identifiers cusip
and year
. All the subsequent manipulations are functioning in the single stock-year dataset as follows.
+----------------------------------------------------------------------+
| cusip r x rm cusipc~e index year |
|----------------------------------------------------------------------|
1. | 00032Q10 266.24 637.6158 54.7 1 1 2020 |
2. | 00032Q10 -1.56 -121.6986 -30.57 1 2 2020 |
3. | 00032Q10 123.44 190.1839 45.48 1 3 2020 |
4. | 00032Q10 233.06 243.7433 -.41 1 4 2020 |
5. | 00032Q10 -599.08 -1396.12 9.139999 1 5 2020 |
|----------------------------------------------------------------------|
6. | 00032Q10 251.22 234.7973 29.41 1 6 2020 |
7. | 00032Q10 -245.06 -288.1359 -12.47 1 7 2020 |
8. | 00032Q10 -178.28 -149.2384 46.14 1 8 2020 |
9. | 00032Q10 -16.5 -76.8955 29.2 1 9 2020 |
10. | 00032Q10 201.65 135.6328 31.06 1 10 2020 |
|----------------------------------------------------------------------|
...
|----------------------------------------------------------------------|
246. | 00032Q10 400 4548.106 -18.55 1 246 2020 |
247. | 00032Q10 -96.15 -1940.581 38.66 1 247 2020 |
248. | 00032Q10 291.26 4240.543 108.42 1 248 2020 |
249. | 00032Q10 -94.34 -937.4673 -2.77 1 249 2020 |
250. | 00032Q10 -285.71 -2000.298 13.5 1 250 2020 |
|----------------------------------------------------------------------|
251. | 00032Q10 -588.24 -2000.485 -84.83 1 251 2020 |
252. | 00032Q10 312.5 1369.296 101.55 1 252 2020 |
253. | 00032Q10 -101.01 -862.252 -10.58 1 253 2020 |
+----------------------------------------------------------------------+
In this step, I also set two global variables that will be repeatedly used in the subsequent procedures.
- the variable names in the VAR system
names
- the number of observations in the dataset unit
rownum
The codes for this step are as follows.
* load dataset unit
use sampledata2020,replace
qui keep if cusipcode == 1
list, nolabel
* set global variables
global names "rm x r"
global rownum = _N
Implement Brogaard Decomposition
For now, we’ve collected all the necessary variables, and get the data ready for Brogaard decomposition. Before we actually start to estimate, I would like to provide a big picture for implementing the Brogaard decomposition in a single stock-year dataset.
The tasks we’re going to resolve are as follows.
-
Estimate the reduced-form VAR in Equation (1), saving the residuals e e e and variance/covariance matrix of residuals Σ e \Sigma_e Σe
-
Estimate matrix B B B, which specifies the contemporaneous effects among variables in the VAR system
-
Estimate the structural shocks ϵ t \epsilon_t ϵt and their variance-covariance matrix Σ ϵ \Sigma_\epsilon Σϵ
-
Estimate the 15-step cumulative structural IRFs θ r m \theta_{rm} θrm, θ x \theta_x θx, θ r \theta_r θr, which represent the (permanent) cumulative return responses to unit shocks of the corresponding structural-model innovations
-
Combine the estimated variances of the structural innovations from step 3 with the long-run responses from step 4 to get the variance components and variance shares of each information source using the following formula.
MktInfo = θ r m 2 σ ε r m 2 PrivateInfo = θ x 2 σ ε x 2 PublicInfo = θ r 2 σ ε r 2 . \begin{aligned} \text { MktInfo } &=\theta_{r_m}^2 \sigma_{\varepsilon_{r_m}}^2 \\ \text { PrivateInfo } &=\theta_x^2 \sigma_{\varepsilon_x}^2 \\ \text { PublicInfo } &=\theta_r^2 \sigma_{\varepsilon_r}^2. \end{aligned} MktInfo PrivateInfo PublicInfo =θrm2σεrm2=θx2σεx2=θr2σεr2. -
Estimate the contemporaneous noise term with the following Equation
Δ s = r t − a 0 − θ r m ϵ r m , t − θ x ϵ x , t − θ r ϵ r , t \Delta_s = r_t-a_0-\theta_{rm}\epsilon_{rm,t}-\theta_x\epsilon_{x,t}-\theta_r\epsilon_{r,t} Δs=rt−a0−θrmϵrm,t−θxϵx,t−θrϵr,t
As we’re only interested in the variance of Δ s \Delta_s Δs, which is by construct the variance from noise, we can ignore the constant term a 0 a_0 a0 and use the variance of KaTeX parse error: Undefined control sequence: \* at position 10: \Delta_s^\̲*̲ to represent the noise variance instead, where
KaTeX parse error: Undefined control sequence: \* at position 48: …)=Var(\Delta_s^\̲*̲)\\ \Delta_s^\*… -
Get variance shares by normalizing these variance components
MktInfoShare = θ r m 2 σ ε r m 2 / ( σ w 2 + σ r 2 ) PrivateInfoShare = θ x 2 σ ε x 2 / ( σ w 2 + σ r 2 ) PublicInfoShare = θ r 2 σ ε r 2 / ( σ w 2 + σ r 2 ) NoiseShare = σ s 2 / ( σ w 2 + σ r 2 ) . \begin{aligned} \text { MktInfoShare } &=\theta_{r_m}^2 \sigma_{\varepsilon_{r_m}}^2 /(\sigma_w^2+\sigma_r^2 )\\ \text { PrivateInfoShare } &=\theta_{x}^2 \sigma_{\varepsilon_x}^2 /(\sigma_w^2+\sigma_r^2 ) \\ \text { PublicInfoShare } &=\theta_r^2 \sigma_{\varepsilon_r}^2 /(\sigma_w^2+\sigma_r^2 ) \\ \text { NoiseShare } &=\sigma_s^2 /(\sigma_w^2+\sigma_r^2 ) . \end{aligned} \notag MktInfoShare PrivateInfoShare PublicInfoShare NoiseShare =θrm2σεrm2/(σw2+σr2)=θx2σεx2/(σw2+σr2)=θr2σεr2/(σw2+σr2)=σs2/(σw2+σr2).
Estimate VAR Coefficients, Matrix B B B, ϵ t \epsilon_t ϵt, Σ e \Sigma_e Σe, and Σ ϵ \Sigma_\epsilon Σϵ
I set the lag order as 5 to keep consistent with Broggard et al. (2022, RFS) and then I use svar
model to estimate the VAR model, with imposing a Cholesky type restriction to contemporaneous matrix
B
B
B as mentioned in the paper.
The readers can see details about the matrix
B
B
B in Dig into Estimation of VAR Coefficients, IRFs, and Variance Decomposition in Stata and see why svar
command can directly estimate it in Estimations of VAR, IRFs, and Variance Decomposition in Stata.
The codes for estimating the reduced-form model are as follows.
* estimate B and coefficients of VAR
matrix A1 = (1,0,0 \ .,1,0 \ .,.,1)
matrix B1 = (.,0,0 \ 0,.,0 \ 0,0,.)
qui svar rm x r, lags(1/5) aeq(A1) beq(B1)
The svar
command stores the matrix
B
B
B as e(A)
, the coefficient matrix as e(b_var)
and the variance/covariance matrix of residuals
Σ
e
\Sigma_e
Σe as e(Sigma)
. So I didn’t estimate them once more but just take them directly from the results of svar
estimation.
* store parameter matrix
mat B = e(A)
mat coef = e(b_var)
mat sigma_hat = e(Sigma)
I adjust the freedom of variance/covariance matrix of residuals generated from svar
command sigma_hat
from
n
n
n to
n
−
1
n-1
n−1 and name the adjusted variance/covariance matrix as sigma_e
. It shouldn’t make much difference if the readers ignore this step.
By definition,
ϵ
t
=
B
e
t
\epsilon_t=Be_t
ϵt=Bet
That also implies
Σ
ϵ
=
B
Σ
e
B
\Sigma_\epsilon=B\Sigma_eB
Σϵ=BΣeB
With above formulas, we can calculate the structural shocks
ϵ
t
\epsilon_t
ϵt and their variance/covariance matrix
Σ
ϵ
\Sigma_\epsilon
Σϵ as follows. I stored the structural shocks in a matrix named epsilons
and the variance/covariance matrix of structural shocks in a matrix named sigma_epsilon
.
** get residuals e_t
foreach var of varlist rm x r{
qui cap predict e_`var', resi equation(`var')
}
** get epsilons
mkmat e_rm e_x e_r, matrix(resi)
mat epsilons = (B*resi')'
* get variance-covariance matrix of residuals and epsilons
mat sigma_e = sigma_hat*_N/(_N-1)
mat sigma_epsilon = B*sigma_e*B'
Estimate 15-step cumulative structural IRFs θ r m \theta_{rm} θrm, θ x \theta_x θx, and θ r \theta_r θr
While the svar
command can easily produce the results for IRFs, Orthogonalized IRFs, and Orthogonalized Structural IRFs automatically, what we need are the un-orthogonalized Structural IRFs.
Procedures for estimating θ \theta θ
These un-orthogonalized Structural IRFs can be quickly and conveniently calculated via the following procedures (please see more details in Dig into Estimation of VAR Coefficients, IRFs, and Variance Decomposition in Stata).
- calculate the IRFs Φ i ( i = 1 , 2 , 3... , 15 ) \Phi_i(i=1,2,3...,15) Φi(i=1,2,3...,15) following the following formula, where k k k is the number of variables in the VAR system. A j A_j Aj is the j j j-lag coefficient matrix for the reduced-form VAR
Φ 0 = I k Φ i = Σ j = 1 i Φ i − j A j (2) \Phi_0 = I_k\\ \Phi_i = \Sigma_{j=1}^{i}\Phi_{i-j}A_j \tag{2} Φ0=IkΦi=Σj=1iΦi−jAj(2)
-
post-multiply IRF Φ i \Phi_i Φi with B − 1 B^{-1} B−1 to get (un-orthogonalized) structural IRFs Λ i \Lambda_i Λi for each forward-looking step i = 1 , 2 , 3 , . . , 15 i=1,2,3,..,15 i=1,2,3,..,15
Λ i = Φ i B − 1 (3) \Lambda_i =\Phi_iB^{-1} \tag{3} Λi=ΦiB−1(3) -
Sum all the 15-step (un-orthogonalized) structural IRFs Λ i \Lambda_i Λi to obtain the cumulative structural IRFs.
-
As we are only interested in the 15-step cumulative structural IRFs functioning on the stock returns, which are specified in the third equation in the VAR system, the θ r m \theta_{rm} θrm, θ x \theta_x θx, and θ r \theta_r θr lie on the third row of the 15-step cumulative structural IRF matrix.
Obtain coefficient matrix A j ( j = 1 , 2 , . . . , 5 ) A_j(j=1,2,...,5) Aj(j=1,2,...,5)
To implement the above procedures, the first thing we need to get are the reduced-form coefficient matrix
A
j
(
j
=
1
,
2
,
.
.
.
,
5
)
A_j(j=1,2,...,5)
Aj(j=1,2,...,5). As we have obtained all the reduce-form coefficients with svar
command and stored them in a matrix coef
in the last step, we don’t have to compute them again but just need to reshape the matrix coef
into the shape we need.
The coefficient matrix we currently have coef
is a
1
×
48
1\times 48
1×48 matrix. I first reshape it to a
3
×
16
3\times 16
3×16 matrix named newcoef
, where each row contains the coefficients for one equation in the VAR system. Within each row, the coefficients are ordered with fixed rules: the coefficients for the first variable rm
with 1 to 5 lags, the coefficients for the second variable x
with 1 to 5 lags, the coefficients for the third variable x
with 1 to 5 lags, and the constant for the corresponding equation. That implies, the coefficients for the same lag can always be found every 5 columns.
With the above observations, I generated matrix A 1 A_1 A1 to A 5 A_5 A5 with the following codes.
* reshape coeficient matrix
cap mat drop newcoef
forvalues i = 1/3{
mat temp= coef[1..1, 1+16*(`i'-1)..16*`i']
mat newcoef = nullmat(newcoef) \ temp
}
* generate a1 to a5
forvalues i = 1/5{
mat A`i' = (newcoef[1..3,`i'], newcoef[1..3,`i'+5], newcoef[1..3,`i'+10])
mat rownames A`i' = $names
mat colnames A`i' = $names
}
I list the 3-lag coefficient matrix A 3 A_3 A3 as an example to show the desired format of coefficient matrix A 1 A_1 A1 to A 5 A_5 A5. For the i j ij ij-th element of the matrix A j A_j Aj, it represents the impact of one-unit reduced-form shock e j t e_{jt} ejt on the Equation with variable i i i as dependent variable.
. mat list A3
A3[3,3]
inflation unrate ffr
inflation -.06574644 .00181085 -.00500138
unrate 1.4581185 .04263687 -1.835178
ffr -.01217184 -.00032878 -.06017122
Calculate IRFs and cumulative un-orthogonalized Structural IRFs
To this stage, we’ve made it clear about the formulas of calculating the IRFs and un-orthogonalized Structural IRFs (please see Equation (2) and (3)) and obtained all the necessary ingredients (coefficient matrix A i A_i Ai and matrix B B B) for the calculations.
The codes for calculating IRFs and cumulative un-orthogonalized Structural IRFs are as follows. I summed up all the un-orthogonalized Structural IRF matrix step by step to get the 15-step cumulative un-orthogonalized Structural IRFs and name it as csirf
.
* calculate IRFs and cumulative un-orthogonalized Structural IRFs
mat irf0 = I(3)
mat sirf0 = irf0*inv(B)
mat csirf = sirf0
forvalues i=1/15{
mat irf`i' = J(3,3,0)
forvalues j = 1/5{
if `i' >= `j'{
local temp = `i'-`j'
mat temp2 = irf`temp'*A`j'
mat irf`i' = irf`i'+ temp2
}
}
mat sirf`i' = irf`i'*inv(B)
mat csirf = csirf + sirf`i'
}
mat rownames csirf = $names
Extract θ \theta θ
The 15-step cumulative un-orthogonalized Structural IRF matrix csirf
is as follows. The
i
j
ij
ij-th element of this matrix represents the permanent (cumulative) impact of one-unit structural shock
ϵ
j
,
t
\epsilon_{j,t}
ϵj,t on the
i
i
i-th Equation in the VAR system.
. mat list csirf
csirf[3,3]
rm x r
rm 1.0590505 .00356754 .00036924
x 11.662946 .84352094 -1.0125034
r .94265444 .01400394 .76133195
By definition, the elements in the 3rd row of the matrix csirf
are
θ
r
m
\theta_{rm}
θrm,
θ
x
\theta_x
θx, and
θ
r
\theta_r
θr respectively.
Thus, we can extract thetas from the matrix csirf
and save the thetas into a new matrix named theta
.
* extract thetas
mat theta = csirf[3..3, 1..3]
Calculate noise term
As we’ve discussed in the road map, the noise variance is given by the following formula.
KaTeX parse error: Undefined control sequence: \* at position 48: …)=Var(\Delta_s^\̲*̲)\\ \Delta_s^\*…
Intuitively, we need to first calculate
Δ
s
∗
\Delta_s^*
Δs∗ by substracting the combinations of structural shocks
ϵ
t
\epsilon_t
ϵt and the permanent impact of structural shocks on stock returns
θ
\theta
θ from the contemporaneous stock return
r
t
r_t
rt.
As we’ve saved the structural shocks
ϵ
t
\epsilon_t
ϵt in a matrix named epsilons
and the permanent impact of structural shocks on stock returns
θ
\theta
θ in a matrix named theta
, the contemporaneous noise term
Δ
s
∗
\Delta_s^*
Δs∗ can be calculated with the following codes, where I save the noise term into a matrix named delta_s
.
To more conveniently produce the variance of the noise term, I saved the noise term matrix delta_s
into a new column named delta_s
in the dataset.
* calculate noise
mkmat r, matrix(r)
mat delta_s = r - (theta*epsilons')'
mat colnames delta_s = "delta_s"
svmat delta_s, names(col)
Calculate the variance from each component
Till now, we’ve collected all the ingredients needed to compute the variance contribution of all the four components defined by the Brogaard et al. (2022, RFS).
Firstly, we calculate the variance contribution from three-types of information with the following formula.
MktInfo
=
θ
r
m
2
σ
ε
r
m
2
PrivateInfo
=
θ
x
2
σ
ε
x
2
PublicInfo
=
θ
r
2
σ
ε
r
2
.
\begin{aligned} \text { MktInfo } &=\theta_{r_m}^2 \sigma_{\varepsilon_{r_m}}^2 \\ \text { PrivateInfo } &=\theta_x^2 \sigma_{\varepsilon_x}^2 \\ \text { PublicInfo } &=\theta_r^2 \sigma_{\varepsilon_r}^2. \end{aligned}
MktInfo PrivateInfo PublicInfo =θrm2σεrm2=θx2σεx2=θr2σεr2.
As we’ve saved the thetas in a matrix theta
and the variance/covariance matrix of structural shocks
ϵ
t
\epsilon_t
ϵt into a matrix sigma_epsilon
, we can calculate the information-based variances as follows.
** calculate information part variance
mat var_epsilon = vecdiag(sigma_epsilon)
mat brogaard = J(1,3,0)
forvalues i = 1/3{
mat brogaard[1,`i']=theta[1, `i']^2*var_epsilon[1, `i']
}
mat brogaard = (brogaard\theta\var_epsilon)
mat rownames brogaard = varpart theta var_epsilon
mat colnames brogaard = $names
Note that I put all the variance components along with the related parameters
θ
\theta
θ and
σ
ϵ
\sigma_\epsilon
σϵ into a new matrix named brogaard
. This matrix looks like as follows.
. mat list brogaard
brogaard[3,3]
rm x r
varpart 17851.6 7821.7685 56649.107
theta .94265444 .01400394 .76133195
var_epsilon 20089.638 39884558 97733.84
Secondly, I calculate the variance contribution from noise, which is proxied by the variance of
Δ
s
∗
\Delta_s^*
Δs∗ we’ve calculated above. Of course, I add the noise variance into the result matrix brogaard
.
** calculate noise part variance
mat brogaard = (brogaard, J(3,1,0))'
qui sum delta_s
mat brogaard[4,1] = r(sd)^2
mat rownames brogaard = $names "s"
After this step, we’ve figured out the variance contribution from each component defined by the Brogaard paper and saved them into the result matrix brogaard
.
The final result matrix brogaard
is as follows.
. mat list brogaard
brogaard[4,3]
varpart theta var_epsilon
rm 17851.6 .94265444 20089.638
x 7821.7685 .01400394 39884558
r 56649.107 .76133195 97733.84
s 20275.294 0 0
Calculate variance contribution
To more conveniently calculate the variance contribution, I saved the result matrix brogaard
into the dataset. I follow the following formula to calculate the variance contribution of each component and save the percentages into a new variable named varpct
.
MktInfoShare
=
θ
r
m
2
σ
ε
r
m
2
/
(
σ
w
2
+
σ
r
2
)
PrivateInfoShare
=
θ
x
2
σ
ε
x
2
/
(
σ
w
2
+
σ
r
2
)
PublicInfoShare
=
θ
r
2
σ
ε
r
2
/
(
σ
w
2
+
σ
r
2
)
NoiseShare
=
σ
s
2
/
(
σ
w
2
+
σ
r
2
)
.
\begin{aligned} \text { MktInfoShare } &=\theta_{r_m}^2 \sigma_{\varepsilon_{r_m}}^2 /(\sigma_w^2+\sigma_r^2 )\\ \text { PrivateInfoShare } &=\theta_{x}^2 \sigma_{\varepsilon_x}^2 /(\sigma_w^2+\sigma_r^2 ) \\ \text { PublicInfoShare } &=\theta_r^2 \sigma_{\varepsilon_r}^2 /(\sigma_w^2+\sigma_r^2 ) \\ \text { NoiseShare } &=\sigma_s^2 /(\sigma_w^2+\sigma_r^2 ) . \end{aligned} \notag
MktInfoShare PrivateInfoShare PublicInfoShare NoiseShare =θrm2σεrm2/(σw2+σr2)=θx2σεx2/(σw2+σr2)=θr2σεr2/(σw2+σr2)=σs2/(σw2+σr2).
keep cusip year
qui keep in 1/4
qui svmat brogaard, names(col)
qui g rownames = ""
local index = 1
foreach name in $names "s"{
qui replace rownames = "`name'" if _n == `index'
local index = `index' + 1
}
egen fullvar = sum(varpart)
qui g varpct = varpart/fullvar*100
Pack codes
Remember the Broggard decomposition is implemented in stock-year level. That means we need to loop over the above codes over the daily observations of each stock in each year. That requires an efficient packing of the codes.
There are two issues worth noted in the packing procedures.
- I require there are at least 50 observations for the estimation of the VAR model
- otherwise, the VAR estimation doesn’t converge or lacks vaidility with too few freedoms
- I require the estimation of VAR model converges
- otherwise, it’s not possible to get converged residuals, which are the prerequisite for the subsequent calculations
cap program drop loopb
cap program define loopb
use sampledata`2',replace
qui keep if cusipcode == `1'
if _N >= 50{
* set global variables
global names "rm x r"
global rownum = _N
* estimate B and coefficients of VAR
matrix A1 = (1,0,0 \ .,1,0 \ .,.,1)
matrix B1 = (.,0,0 \ 0,.,0 \ 0,0,.)
qui svar rm x r, lags(1/5) aeq(A1) beq(B1)
mat B = e(A)
mat coef = e(b_var)
mat sigma_hat = e(Sigma)
* get coefficient matrix of var a1-a5
* reshape coeficient matrix
cap mat drop newcoef
forvalues i = 1/3{
mat temp= coef[1..1, 1+16*(`i'-1)..16*`i']
mat newcoef = nullmat(newcoef) \ temp
}
* generate a1 to a5
forvalues i = 1/5{
mat A`i' = (newcoef[1..3,`i'], newcoef[1..3,`i'+5], newcoef[1..3,`i'+10])
mat rownames A`i' = $names
mat colnames A`i' = $names
}
* get 15-step coefficients of VMA (irf), structual irf (sirf), and cumulative sirf
mat irf0 = I(3)
mat sirf0 = irf0*inv(B)
mat csirf = sirf0
forvalues i=1/15{
mat irf`i' = J(3,3,0)
forvalues j = 1/5{
if `i' >= `j'{
local temp = `i'-`j'
mat temp2 = irf`temp'*A`j'
mat irf`i' = irf`i'+ temp2
}
}
mat sirf`i' = irf`i'*inv(B)
mat csirf = csirf + sirf`i'
}
* extract thetas
mat theta = csirf[3..3, 1..3]
* get reisduals, epsilons, and their variance-covariance matrix
** get residuals
foreach var of varlist rm x r{
qui cap predict e_`var', resi equation(`var')
}
capture confirm variable e_rm
if (_rc == 0){
** get epsilons
mkmat e_rm e_x e_r, matrix(resi)
mat epsilons = (B*resi')'
** get variance-covariance matrix of residuals and epsilons
mat sigma_e = sigma_hat*_N/(_N-1)
mat sigma_epsilon = B*sigma_e*B'
* calculate noise
mkmat r, matrix(r)
mat delta_s = r - (theta*epsilons')'
mat colnames delta_s = "delta_s"
svmat delta_s, names(col)
* calculate variance decomposition of each part
** calculate information part variance
mat var_epsilon = vecdiag(sigma_epsilon)
mat brogaard = J(1,3,0)
forvalues i = 1/3{
mat brogaard[1,`i']=theta[1, `i']^2*var_epsilon[1, `i']
}
mat brogaard = (brogaard\theta\var_epsilon)
mat rownames brogaard = varpart theta var_epsilon
** calculate noise part variance
mat brogaard = (brogaard, J(3,1,0))'
qui sum delta_s
mat brogaard[4,1] = r(sd)^2
mat rownames brogaard = $names "s"
*mat list brogaard
** save the variance decomposition results
keep cusip year
qui keep in 1/4
qui svmat brogaard, names(col)
qui g rownames = ""
local index = 1
foreach name in $names "s"{
qui replace rownames = "`name'" if _n == `index'
local index = `index' + 1
}
egen fullvar = sum(varpart)
qui g varpct = varpart/fullvar*100
* save final results
local savename = "C:\Users\xu-m\Documents\testVAR\resvardecompose\brogaard_'$cusip'_$year.dta"
qui save "`savename'", replace
}
}
end
Loop over sample
I run the packed code over stocks in each year and collected all the results for different years together. Then I reshaped the dataset into panel data. The codes are as follows.
cd C:\Users\xu-m\Documents\testVAR\resvardecompose
* collect results for each year
cap program drop collectbroggardbyyear
cap program define collectbroggardbyyear
clear
set obs 0
save brogaard`1', replace emptyok
local ff : dir . files "*_`1'.dta"
local yearnum : word count "`ff'"
local index = 1
foreach f of local ff {
append using "`f'"
di "`index' of `yearnum' in year `1'"
local index = `index' + 1
}
save brogaard`1', replace
end
* collect all years
clear
set obs 0
save ../brogaard, replace emptyok
forvalues year = 2005/2021{
collectbroggardbyyear `year'
use ../brogaard, replace
append using brogaard`year'
save ../brogaard, replace
}
* reshape into panel data
cd ../
use brogaard, replace
rename fullvar_r sdr
replace rownames = "_"+rownames
reshape wide varpart theta var_epsilon fullvar varpct sdr, i(cusip year) j(rownames) string
rename varpct_rm mktinfo
rename varpct_x privteinfo
rename varpct_r publicinfo
rename varpct_s noise
keep cusip year *info noise
save panelbrogaard, replace
The final outcome is as follows.
. list in 1/20
+-------------------------------------------------------------+
| cusip year public~o mktinfo noise privte~o |
|-------------------------------------------------------------|
1. | 00030710 2014 .4612017 17.60379 58.85497 23.08004 |
2. | 00030710 2015 43.65164 10.60914 12.57214 33.16709 |
3. | 00030710 2016 41.93059 13.32436 6.182855 38.5622 |
4. | 00030710 2017 54.59381 1.191425 14.96389 29.25087 |
5. | 00030710 2018 43.838 25.09339 8.907529 22.16108 |
|-------------------------------------------------------------|
6. | 00030710 2019 30.02275 25.98764 12.31215 31.67746 |
7. | 00032Q10 2018 18.90079 23.99627 36.99344 20.10949 |
8. | 00032Q10 2019 59.49644 5.478648 11.91035 23.11457 |
9. | 00032Q10 2020 55.21476 17.3996 19.76192 7.623722 |
10. | 00032Q10 2021 51.75403 25.38084 12.44535 10.41978 |
|-------------------------------------------------------------|
11. | 00036020 2005 40.70956 7.924543 26.06547 25.30043 |
12. | 00036020 2006 38.53111 21.68062 6.496971 33.29131 |
13. | 00036020 2007 32.78075 4.495751 27.55989 35.1636 |
14. | 00036020 2008 21.36486 47.52046 16.44107 14.6736 |
15. | 00036020 2009 34.37528 31.25706 17.96114 16.40653 |
|-------------------------------------------------------------|
16. | 00036020 2010 39.55574 34.02036 8.709065 17.71484 |
17. | 00036020 2011 21.50586 17.19429 46.27998 15.01987 |
18. | 00036020 2012 52.9045 11.12552 11.89774 24.07223 |
19. | 00036020 2013 12.02968 14.62235 16.48395 56.86403 |
20. | 00036020 2014 31.94392 42.19098 8.426245 17.43885 |
+-------------------------------------------------------------
Conclusion
In this blog, I replicated the stock volatility decomposition method introduced by Brogaard et al. (2022, RFS). Given the potential of this information-based decomposition method as I’ve discussed in Theory for the information-based decomposition of stock price, I hope this blog can help the readers make use of this method to empower their own research.