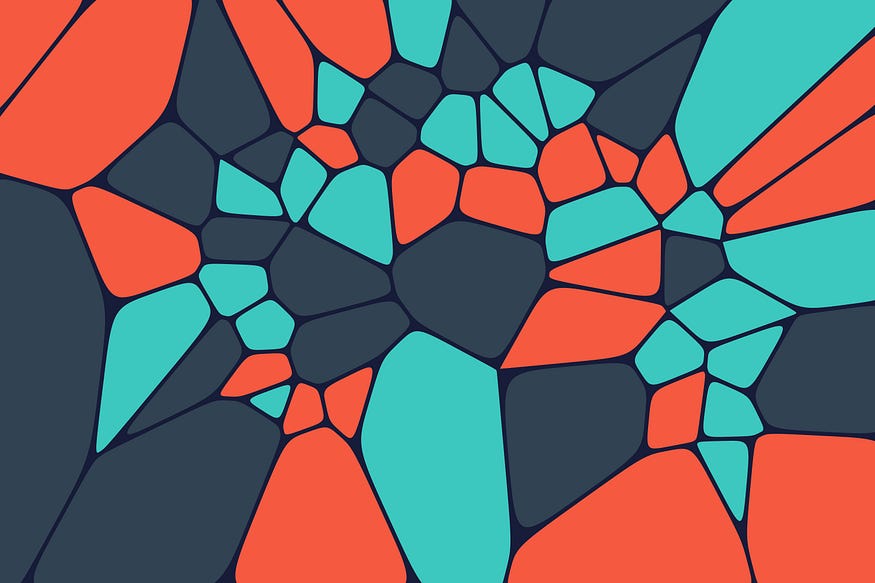
一、说明
SVM(支持向量机)简单而优雅用于分类和回归的监督机器学习方法。该算法试图找到一个超平面,将数据分为不同的类,并具有尽可能最大的边距。本篇我们将介绍如果最大边距不存在的时候,如何创造最大边距。
二、让我们逐步了解 SVM
假设我们有一维湿度数据,红点代表不下雨的日子,蓝点代表下雨的日子。

根据我们拥有的一维观测数据,我们可以确定阈值。该阈值将充当分类器。由于我们的数据是一维的,分类器将有一个阈值。如果我们的数据是二维的,我们会使用一条线。
观察到的数据(最近的数据点)与分类器阈值之间的最短距离称为边距。能够提供最大margin的阈值称为Maximal Margin Classifier (Hyperplane)。在我们的例子中,它将位于双方最接近数据的中点。
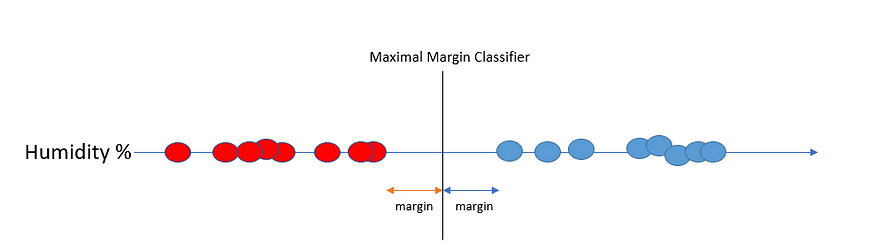
最大保证金在实践中不太适用。因为它对异常值没有抵抗力。想象一下,我们有一个具有蓝色值的离群红点。在这种情况下,分类器将非常接近蓝点,远离红点。
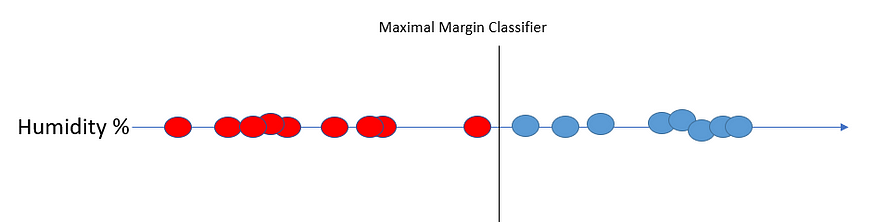
为了改善这一点,我们应该允许异常值和错误分类。我们在系统中引入偏差(并减少方差)。现在,边距称为软边距。使用软间隔的分类器称为支持向量分类器或软间隔分类器。边缘上和软边缘内的数据点称为支持向量。
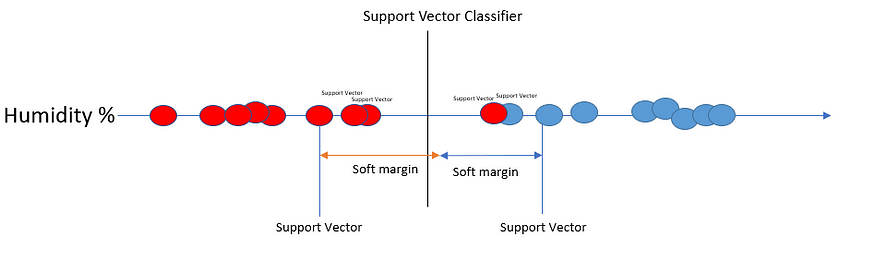
我们使用交叉验证来确定软边距应该在哪里。
在 2D 数据中,支持向量分类器是一条线。在 3D 中,它是一个平面。在 4 个或更多维度中,支持向量分类器是一个超平面。从技术上讲,所有 SVC 都是超平面,但在 2D 情况下更容易将它们称为平面。
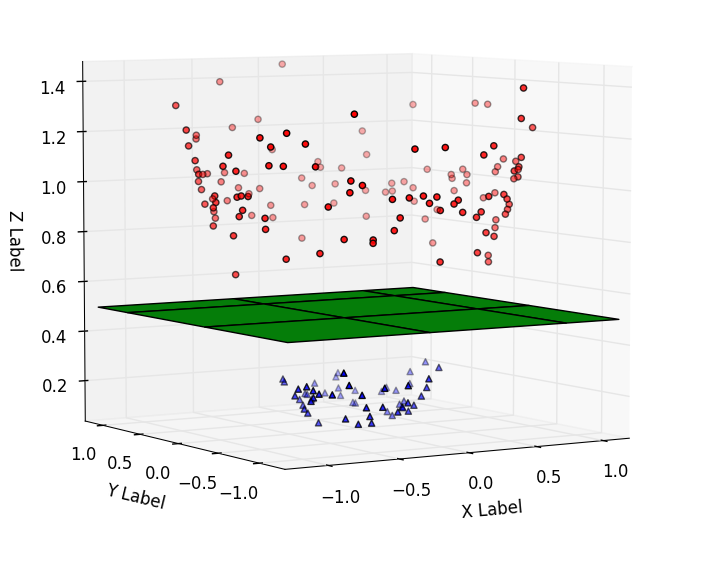
//www.analyticsvidhya.com/blog/2021/05/support-vector-machines/和https://www.sciencedirect.com/topics/computer-science/support-vector-machine
正如我们在上面看到的,支持向量分类器可以处理异常值并允许错误分类。但是,我们如何处理如下所示的重叠数据呢?

这就是支持向量机发挥作用的地方。让我们为问题添加另一个维度。我们有特征 X,作为新的维度,我们取 X 的平方并将其绘制在 y 轴上。
由于现在的数据是二维的,我们可以画一条支持向量分类器线。
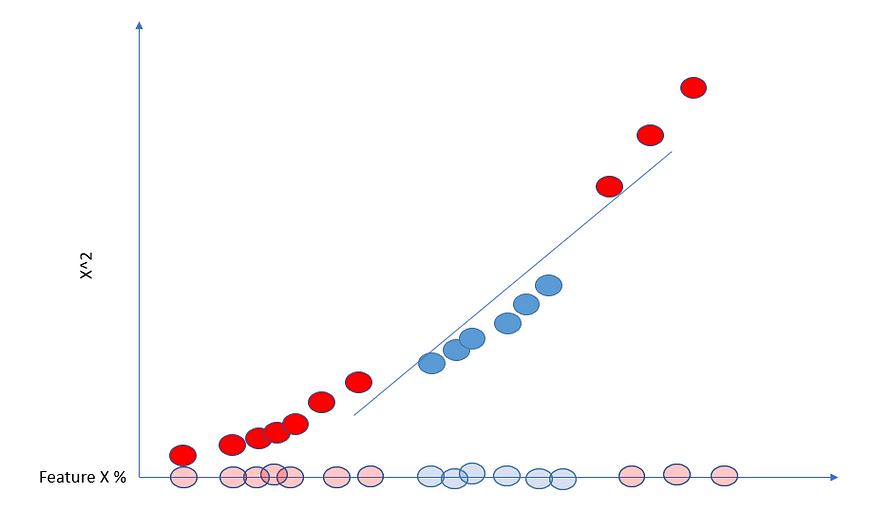
支持向量机获取低维数据,将其移至更高维度,并找到支持向量分类器。
与我们上面所做的类似,支持向量机使用核函数来查找更高维度的支持向量分类器。核函数是一种函数,它采用原始输入空间中的两个输入数据点,并计算变换后(高维)特征空间中它们对应的特征向量的内积。
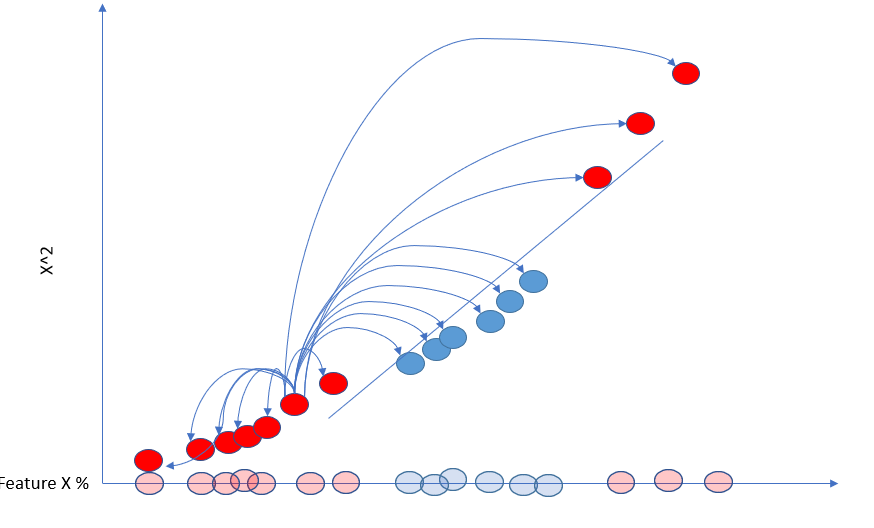
核函数允许 SVM 在变换后的特征空间中运行,而无需显式计算变换后的特征向量,这对于大型数据集或复杂的变换来说计算成本可能很高。相反,核函数直接在原始输入空间中计算特征向量之间的内积。这称为内核技巧。
三、多项式核
多项式核用于将输入数据从低维空间变换到高维空间,在高维空间中使用线性决策边界更容易分离类。
多项式核。
a和b是两个不同的观测值,r是多项式系数,d是多项式的次数。假设d为 2,r为 1/2。
数学。
我们最终得到一个点积。第一项(a和b)是 x 轴,第二项(a²和b²)是 y 轴。因此,我们需要做的就是计算每对点之间的点积。例如更高维度中两点之间的关系;a = 9,b = 14 => (9 x 114 + 1/2)² = 16000,25。
四、径向内核 (RBF)
径向核在无限维度中查找支持向量分类器。
它为距离测试点较近的点分配较高的权重,为较远的点(如最近的邻居)分配较低的权重。较远的观察对数据点的分类影响相对较小。
内核函数。
它计算两个数据之间的平方距离。Gamma 由交叉验证确定,它会缩放平方距离,这意味着它会缩放两个点彼此之间的影响。在此公式中,随着两点之间的距离增加,该值将接近于零。
当类之间的决策边界是非线性且复杂的时,径向核特别有用,因为它可以捕获输入特征之间的复杂关系。
五、Python实现
我们可以使用支持向量机sklearn.
from sklearn.svm import SVC
具有不同内核的 SVC。来源
SVC
接受一些参数:
C
是正则化参数。较大的值会使模型在训练数据上犯更多错误(错误分类)。因此,它的目的是有一个更好的概括。默认值为 1。kernel
设置核函数。默认为rbf。其他选择是:Linear、poly、sigmoid和precompulated。此外,您还可以传递自己的内核函数。degree
指定多项式核的次数。仅当内核是多项式时它才可用。默认值为 3。gamma
控制核函数的形状。它可用于rbf、poly和sigmoid内核,较小的 gamma 值使决策边界更平滑,较大的值使决策边界更复杂。默认值是比例,等于 1 / (n_features x X.var())。auto是 1 / n_features。或者您可以传递一个浮点值。coef0
仅用于 poly 和 sigmoid 内核。它控制多项式核函数中高阶项的影响。默认值为 0。shrinking
控制是否使用收缩启发式。这是一个加速启发式过程。tol
是停止标准的容差。当目标函数的变化小于tol时,优化过程将停止。class_weight
平衡分类问题中类别的权重。可以将其设置为平衡,以根据课程频率自动调整权重。默认值为“无”。max_iter
是迭代极限。-1 表示无限制(默认)。probability
指定是否启用概率估计。当它设置为 True 时,估计器将估计类概率,而不仅仅是返回预测的类标签。当probability
设置为 True 时,可以使用predict_proba
该类的方法来获取新数据点的类标签的估计概率。SVC
cache_size
用于设置SVM算法使用的内核缓存的大小。当训练样本数量非常大或者内核计算成本很高时,内核缓存会很有用。通过将核评估存储在缓存中,SVM 算法可以在计算正则化参数 C 的不同值的决策函数时重用结果。
SVC 使用具有不同参数的 RBF 内核。来源
一个简单的实现:
from sklearn.datasets import load_breast_cancer
from sklearn.model_selection import train_test_split
from sklearn.svm import SVC
# cancer data
cancer = load_breast_cancer()
X_train, X_test, y_train, y_test = train_test_split(cancer.data, cancer.target, random_state=42)
# parameters
params = {'C': 1.0, 'kernel': 'rbf', 'gamma': 'scale',
'probability': False, 'cache_size': 200}
# training
svc = SVC(**params)
svc.fit(X_train, y_train)
# we can use svc's own score function
score = svc.score(X_test, y_test)
print("Accuracy on test set: {:.2f}".format(score))
#Accuracy on test set: 0.95
六、回归
我们也可以在回归问题中使用支持向量机。
from sklearn.svm import SVR
epsilon
是指定回归线周围容差大小的参数。回归线由 SVR 算法确定,使其在一定的误差范围内拟合训练数据,该误差范围由参数定义epsilon
。
from sklearn.datasets import fetch_california_housing
from sklearn.model_selection import train_test_split
from sklearn.svm import SVR
from sklearn.metrics import mean_squared_error
# the California Housing dataset
california = fetch_california_housing()
X_train, X_test, y_train, y_test = train_test_split(california.data, california.target, random_state=42)
# training
svr = SVR(kernel='rbf', C=1.0, epsilon=0.1)
svr.fit(X_train, y_train)
# Evaluate the model on the testing data
y_pred = svr.predict(X_test)
mse = mean_squared_error(y_test, y_pred)
print("MSE on test set: {:.2f}".format(mse))
#MSE on test set: 1.35
我们还可以使用 来绘制边界matplotlib
。
import numpy as np
import matplotlib.pyplot as plt
from sklearn.datasets import load_iris
from sklearn.svm import SVC
# Load the Iris dataset
iris = load_iris()
# Extract the first two features (sepal length and sepal width)
X = iris.data[:, :2]
y = iris.target
# Create an SVM classifier
svm = SVC(kernel='linear', C=1.0)
svm.fit(X, y)
# Create a mesh of points to plot in
x_min, x_max = X[:, 0].min() - 1, X[:, 0].max() + 1
y_min, y_max = X[:, 1].min() - 1, X[:, 1].max() + 1
xx, yy = np.meshgrid(np.arange(x_min, x_max, 0.02),
np.arange(y_min, y_max, 0.02))
# Plot the decision boundary
Z = svm.predict(np.c_[xx.ravel(), yy.ravel()])
Z = Z.reshape(xx.shape)
plt.contourf(xx, yy, Z, cmap=plt.cm.Paired, alpha=0.8)
# Plot the training points
plt.scatter(X[:, 0], X[:, 1], c=y, cmap=plt.cm.Paired)
plt.xlabel('Sepal length')
plt.ylabel('Sepal width')
plt.xlim(xx.min(), xx.max())
plt.ylim(yy.min(), yy.max())
plt.xticks(())
plt.yticks(())
plt.title('SVM decision boundary for Iris dataset')
plt.show()
边界。图片由作者提供。
SVM 是一种相对较慢的方法。
import time
from sklearn.datasets import load_breast_cancer
from sklearn.linear_model import LogisticRegression
from sklearn.svm import SVC
from sklearn.model_selection import train_test_split
# Load the breast cancer dataset
data = load_breast_cancer()
X, y = data.data, data.target
# Split the data into training and testing sets
X_train, X_test, y_train, y_test = train_test_split(X, y, random_state=42)
# Fit a logistic regression model and time it
start_time = time.time()
lr = LogisticRegression(max_iter=1000)
lr.fit(X_train, y_train)
end_time = time.time()
lr_runtime = end_time - start_time
# Fit an SVM model and time it
start_time = time.time()
svm = SVC(kernel='linear', C=1.0)
svm.fit(X_train, y_train)
end_time = time.time()
svm_runtime = end_time - start_time
# Print the runtimes
print("Logistic regression runtime: {:.3f} seconds".format(lr_runtime))
print("SVM runtime: {:.3f} seconds".format(svm_runtime))
"""
Logistic regression runtime: 0.112 seconds
SVM runtime: 0.547 seconds
"""
支持向量机 (SVM) 可能会很慢,原因如下:
- SVM 是计算密集型的:SVM 涉及解决凸优化问题,对于具有许多特征的大型数据集来说,计算成本可能很高。SVM 的时间复杂度通常至少为 O(n²),其中 n 是数据点的数量,对于非线性内核来说,时间复杂度可能要高得多。
- 用于调整超参数的交叉验证:SVM需要调整超参数,例如正则化参数
C
和核超参数,这涉及使用交叉验证来评估不同的超参数设置。这可能非常耗时,尤其是对于大型数据集或复杂模型。 - 大量支持向量:对于非线性SVM,支持向量的数量会随着数据集的大小或模型的复杂性而快速增加。这可能会减慢预测时间,尤其是在模型需要频繁重新训练的情况下。
我们可以通过尝试以下一些方法来加速 SVM:
- 使用线性核:线性 SVM 的训练速度比非线性 SVM 更快,因为优化问题更简单。如果您的数据是线性可分的或者不需要高度复杂的模型,请考虑使用线性核。
from sklearn.datasets import fetch_openml
from sklearn.model_selection import train_test_split
from sklearn.svm import SVC, LinearSVC
import time
# Load MNIST digits dataset
mnist = fetch_openml('mnist_784', version=1)
data, target = mnist['data'], mnist['target']
X_train, X_test, y_train, y_test = train_test_split(data, target, test_size=0.2, random_state=42)
# Train linear SVM
start_time = time.time()
linear_svc = LinearSVC()
linear_svc.fit(X_train, y_train)
linear_train_time = time.time() - start_time
# Train non-linear SVM with RBF kernel
start_time = time.time()
rbf_svc = SVC(kernel='rbf')
rbf_svc.fit(X_train, y_train)
rbf_train_time = time.time() - start_time
print('Linear SVM training time:', linear_train_time)
print('Non-linear SVM training time:', rbf_train_time)
"""
Linear SVM training time: 109.03955698013306
Non-linear SVM training time: 165.98812198638916
"""
- 使用较小的数据集:如果您的数据集非常大,请考虑使用较小的数据子集进行训练。您可以使用随机抽样或分层抽样等技术来确保子集代表完整数据集。
- 使用特征选择:如果您的数据集具有许多特征,请考虑使用特征选择技术来减少特征数量。这可以降低问题的维度并加快训练速度。
- 使用较小的值
C
:正则化参数C
控制最大化边际和最小化分类误差之间的权衡。较小的值C
可以产生具有较少支持向量的更简单的模型,这可以加速训练和预测。
import time
from sklearn.datasets import load_breast_cancer
from sklearn.svm import SVC
from sklearn.model_selection import train_test_split
# Load the breast cancer dataset
data = load_breast_cancer()
X, y = data.data, data.target
# Split the data into training and testing sets
X_train, X_test, y_train, y_test = train_test_split(X, y, random_state=42)
for C in [0.1, 1, 10]:
start_time = time.time()
svm = SVC(kernel='linear', C=C, random_state=42)
svm.fit(X_train, y_train)
train_time = time.time() - start_time
print('Training time with C={}: {:.2f}s'.format(C, train_time))
"""
Training time with C=0.1: 0.08s
Training time with C=1: 0.55s
Training time with C=10: 0.90s
"""
- 使用缓存:SVM 涉及计算数据点对之间的内积,这可能会导致计算成本高昂。Scikit-learn 的 SVM 实现包括一个缓存,用于存储常用数据点的内积值,这可以加快训练和预测速度。您可以使用参数调整缓存的大小
cache_size
。
from sklearn.datasets import load_breast_cancer
from sklearn.svm import SVC
import time
# Load the dataset
X, y = load_breast_cancer(return_X_y=True)
# Train the model without a cache
start_time = time.time()
clf = SVC(kernel='linear', cache_size=1).fit(X, y)
end_time = time.time()
print(f"Training time without cache: {end_time - start_time:.3f} seconds")
# Train the model with a cache of 200 MB
start_time = time.time()
clf_cache = SVC(kernel='linear', cache_size=200, max_iter=10000).fit(X, y)
end_time = time.time()
print(f"Training time with cache: {end_time - start_time:.3f} seconds")
"""
Training time without cache: 0.535 seconds
Training time with cache: 0.014 seconds
"""
七、结论
一般来说,SVM 适用于特征数量与样本数量相比相对较少且不同类之间有明显分离余量的分类任务。SVM 还可以处理高维数据以及特征和目标变量之间的非线性关系。然而,SVM 可能不适合非常大的数据集,因为它们可能是计算密集型的并且需要大量内存。
参考文章: