在前面的Arrow系列文章中,我们介绍了Arrow的基础数据类型[1]以及高级数据类型[2],这让我们具备了在内存中建立起一个immutable数据集的能力。但这并非我们的目标,我们最终是要对建立起来的数据集进行查询和分析等操作(manipulation)的。
在这一篇文章中,我们就来看看如何基于Go arrow的实现对内存中的Arrow数据集进行操作。
注:由于Arrow官方文档尚没有Go语言的cookbook,这里的一些例子参考了其他语言的Cookbook,比如Python[3]。
1. 从CSV文件中读取数据
在操作数据之前,我们首先需要准备数据,并将数据读取到内存中以Arrow的列式存储形式组织起来。Arrow的Go实现支持从多种文件格式中将数据读取出来并在内存中构建出Arrow列式数据集:
从图中我们看到:Arrow Go支持读取的文件格式包括CSV、JSON和Parquet[4]等。CSV、JSON都是日常最常用的文件格式,那么Parquet是什么呢?这是一种面向列的文件存储格式,支持高效的数据存储和数据获取能力。influxdb iox存储引擎采用的就是Apache Arrow + Parquet的经典组合。我们在本系列的后续文章中会单独说一下Arrow + Parquet,在本文中Parquet不是重点。
注:Parquet的读音是:['pɑːkeɪ] 。
在这篇文章中,我们以从CSV文件中读取数据为例。我们的CSV文件来自于Kaggle平台上的开放数据集[5],这是一份记录着Delhi这个地方(应该是印度城市德里)1996年到2017年小时级的天气数据的CSV文件[6]:testset.csv。该文件带有列头,有20列,10w多行记录。
我们先来小试牛刀,即取该csv文件前10几行,存成名为testset.tiny.csv的文件。我们编写一段Go程序来读取CSV中的数据并在内存中建立一个Arrow Record Batch!大家还记得Arrow Record Batch是什么结构了么?我们回顾一下“高级数据结构”[7]中的那张图你就记起来了:
接下来我们就使用Arrow Go实现提供的csv包读取testset.tiny.csv文件并输出经由读出的数据建构的Record Batch:
// read_tiny_csv_multi_trunks.go
package main
import (
"fmt"
"io"
"os"
"github.com/apache/arrow/go/v13/arrow/csv"
)
func read(data io.ReadCloser) error {
// read 5 lines at a time to create record batches
rdr := csv.NewInferringReader(data, csv.WithChunk(5),
// strings can be null, and these are the values
// to consider as null
csv.WithNullReader(true, "", "null", "[]"),
// assume the first line is a header line which names the columns
csv.WithHeader(true))
for rdr.Next() {
rec := rdr.Record()
fmt.Println(rec)
}
return nil
}
func main() {
data, err := os.Open("./testset.tiny.csv")
if err != nil {
panic(err)
}
read(data)
}
这里的csv包可不是标准库中的那个包,而是Arrow Go实现中专门用于将csv文件数据读取并转换为Arrow内存对象的包。csv包提供了两个创建csv.Reader实例的函数,这里使用的是NewInferringReader(即带列类型推导的Reader)。该函数可以自动读取位于第一行的csv文件的header,获取列字段的名称与个数,形成Record的schema,并在读取下一条记录时尝试推导(infer)这一列的类型(data type)。
这里在调用NewInferringReader时还传入了一个功能选项开关WithChunk(5),即一次读取5条记录来构建一个新的Record Batch。
我们运行一下上面的代码:
$go run read_tiny_csv_multi_trunks.go
record:
schema:
fields: 20
- datetime_utc: type=utf8, nullable
- _conds: type=utf8, nullable
- _dewptm: type=int64, nullable
- _fog: type=int64, nullable
- _hail: type=int64, nullable
- _heatindexm: type=utf8, nullable
- _hum: type=int64, nullable
- _precipm: type=utf8, nullable
- _pressurem: type=int64, nullable
- _rain: type=int64, nullable
- _snow: type=int64, nullable
- _tempm: type=int64, nullable
- _thunder: type=int64, nullable
- _tornado: type=int64, nullable
- _vism: type=int64, nullable
- _wdird: type=int64, nullable
- _wdire: type=utf8, nullable
- _wgustm: type=utf8, nullable
- _windchillm: type=utf8, nullable
- _wspdm: type=float64, nullable
rows: 5
col[0][datetime_utc]: ["19961101-11:00" "19961101-12:00" "19961101-13:00" "19961101-14:00" "19961101-16:00"]
col[1][ _conds]: ["Smoke" "Smoke" "Smoke" "Smoke" "Smoke"]
col[2][ _dewptm]: [9 10 11 10 11]
col[3][ _fog]: [0 0 0 0 0]
col[4][ _hail]: [0 0 0 0 0]
col[5][ _heatindexm]: [(null) (null) (null) (null) (null)]
col[6][ _hum]: [27 32 44 41 47]
col[7][ _precipm]: [(null) (null) (null) (null) (null)]
col[8][ _pressurem]: [1010 -9999 -9999 1010 1011]
col[9][ _rain]: [0 0 0 0 0]
col[10][ _snow]: [0 0 0 0 0]
col[11][ _tempm]: [30 28 24 24 23]
col[12][ _thunder]: [0 0 0 0 0]
col[13][ _tornado]: [0 0 0 0 0]
col[14][ _vism]: [5 (null) (null) 2 (null)]
col[15][ _wdird]: [280 0 0 0 0]
col[16][ _wdire]: ["West" "North" "North" "North" "North"]
col[17][ _wgustm]: [(null) (null) (null) (null) (null)]
col[18][ _windchillm]: [(null) (null) (null) (null) (null)]
col[19][ _wspdm]: [7.4 (null) (null) (null) 0]
我们看到结果输出了将csv文件中数据读取并转换后的Record Batch的信息!
不过这个结果有一个问题,那就是我们的testset.tiny.csv有12行数据,上述结果为什么仅读出了5行呢?利用go.work[8]引用本地下载的arrow代码做一下print调试后发现这样的一个错误:
strconv.ParseInt: parsing "1.2": invalid syntax
翻看一下testset.tiny.csv文件,在第五行发现了包含1.2的这条数据:
19961101-16:00,Smoke,11,0,0,,47,,1011,0,0,23,0,0,1.2,0,North,,,0
1.2这数据对应的是" _vism"这一列,我们看一下上面这一列的schema信息:
- _vism: type=int64, nullable
我们看到NewInferringReader将这一列识别成int64类型了!NewInferringReader是根据第一行数据中来做类型推导的,而vism这一列的第一条数据恰为5,将其推导为int64也就不足为奇了。那么如何修正上述问题呢?NewInferringReader提供了一个WithColumnTypes的功能选项,通过它我们可以指定vism列的Arrow DataType:
rdr := csv.NewInferringReader(data, csv.WithChunk(5),
// strings can be null, and these are the values
// to consider as null
csv.WithNullReader(true, "", "null", "[]"),
// assume the first line is a header line which names the columns
csv.WithHeader(true),
csv.WithColumnTypes(map[string]arrow.DataType{
" _vism": arrow.PrimitiveTypes.Float64,
}),
)
修改后,我们再来运行一下read_tiny_csv_multi_trunks.go这个文件的代码:
$go run read_tiny_csv_multi_trunks.go
record:
schema:
fields: 20
- datetime_utc: type=utf8, nullable
- _conds: type=utf8, nullable
- _dewptm: type=int64, nullable
- _fog: type=int64, nullable
- _hail: type=int64, nullable
- _heatindexm: type=utf8, nullable
- _hum: type=int64, nullable
- _precipm: type=utf8, nullable
- _pressurem: type=int64, nullable
- _rain: type=int64, nullable
- _snow: type=int64, nullable
- _tempm: type=int64, nullable
- _thunder: type=int64, nullable
- _tornado: type=int64, nullable
- _vism: type=float64, nullable
- _wdird: type=int64, nullable
- _wdire: type=utf8, nullable
- _wgustm: type=utf8, nullable
- _windchillm: type=utf8, nullable
- _wspdm: type=float64, nullable
rows: 5
col[0][datetime_utc]: ["19961101-11:00" "19961101-12:00" "19961101-13:00" "19961101-14:00" "19961101-16:00"]
col[1][ _conds]: ["Smoke" "Smoke" "Smoke" "Smoke" "Smoke"]
col[2][ _dewptm]: [9 10 11 10 11]
col[3][ _fog]: [0 0 0 0 0]
col[4][ _hail]: [0 0 0 0 0]
col[5][ _heatindexm]: [(null) (null) (null) (null) (null)]
col[6][ _hum]: [27 32 44 41 47]
col[7][ _precipm]: [(null) (null) (null) (null) (null)]
col[8][ _pressurem]: [1010 -9999 -9999 1010 1011]
col[9][ _rain]: [0 0 0 0 0]
col[10][ _snow]: [0 0 0 0 0]
col[11][ _tempm]: [30 28 24 24 23]
col[12][ _thunder]: [0 0 0 0 0]
col[13][ _tornado]: [0 0 0 0 0]
col[14][ _vism]: [5 (null) (null) 2 1.2]
col[15][ _wdird]: [280 0 0 0 0]
col[16][ _wdire]: ["West" "North" "North" "North" "North"]
col[17][ _wgustm]: [(null) (null) (null) (null) (null)]
col[18][ _windchillm]: [(null) (null) (null) (null) (null)]
col[19][ _wspdm]: [7.4 (null) (null) (null) 0]
record:
schema:
fields: 20
- datetime_utc: type=utf8, nullable
- _conds: type=utf8, nullable
- _dewptm: type=int64, nullable
- _fog: type=int64, nullable
- _hail: type=int64, nullable
- _heatindexm: type=utf8, nullable
- _hum: type=int64, nullable
- _precipm: type=utf8, nullable
- _pressurem: type=int64, nullable
- _rain: type=int64, nullable
- _snow: type=int64, nullable
- _tempm: type=int64, nullable
- _thunder: type=int64, nullable
- _tornado: type=int64, nullable
- _vism: type=float64, nullable
- _wdird: type=int64, nullable
- _wdire: type=utf8, nullable
- _wgustm: type=utf8, nullable
- _windchillm: type=utf8, nullable
- _wspdm: type=float64, nullable
rows: 5
col[0][datetime_utc]: ["19961101-17:00" "19961101-18:00" "19961101-19:00" "19961101-20:00" "19961101-21:00"]
col[1][ _conds]: ["Smoke" "Smoke" "Smoke" "Smoke" "Smoke"]
col[2][ _dewptm]: [12 13 13 13 13]
col[3][ _fog]: [0 0 0 0 0]
col[4][ _hail]: [0 0 0 0 0]
col[5][ _heatindexm]: [(null) (null) (null) (null) (null)]
col[6][ _hum]: [56 60 60 68 68]
col[7][ _precipm]: [(null) (null) (null) (null) (null)]
col[8][ _pressurem]: [1011 1010 -9999 -9999 1010]
col[9][ _rain]: [0 0 0 0 0]
col[10][ _snow]: [0 0 0 0 0]
col[11][ _tempm]: [21 21 21 19 19]
col[12][ _thunder]: [0 0 0 0 0]
col[13][ _tornado]: [0 0 0 0 0]
col[14][ _vism]: [(null) 0.8 (null) (null) (null)]
col[15][ _wdird]: [0 0 0 0 0]
col[16][ _wdire]: ["North" "North" "North" "North" "North"]
col[17][ _wgustm]: [(null) (null) (null) (null) (null)]
col[18][ _windchillm]: [(null) (null) (null) (null) (null)]
col[19][ _wspdm]: [(null) 0 (null) (null) (null)]
record:
schema:
fields: 20
- datetime_utc: type=utf8, nullable
- _conds: type=utf8, nullable
- _dewptm: type=int64, nullable
- _fog: type=int64, nullable
- _hail: type=int64, nullable
- _heatindexm: type=utf8, nullable
- _hum: type=int64, nullable
- _precipm: type=utf8, nullable
- _pressurem: type=int64, nullable
- _rain: type=int64, nullable
- _snow: type=int64, nullable
- _tempm: type=int64, nullable
- _thunder: type=int64, nullable
- _tornado: type=int64, nullable
- _vism: type=float64, nullable
- _wdird: type=int64, nullable
- _wdire: type=utf8, nullable
- _wgustm: type=utf8, nullable
- _windchillm: type=utf8, nullable
- _wspdm: type=float64, nullable
rows: 2
col[0][datetime_utc]: ["19961101-22:00" "19961101-23:00"]
col[1][ _conds]: ["Smoke" "Smoke"]
col[2][ _dewptm]: [13 12]
col[3][ _fog]: [0 0]
col[4][ _hail]: [0 0]
col[5][ _heatindexm]: [(null) (null)]
col[6][ _hum]: [68 64]
col[7][ _precipm]: [(null) (null)]
col[8][ _pressurem]: [1009 1009]
col[9][ _rain]: [0 0]
col[10][ _snow]: [0 0]
col[11][ _tempm]: [19 19]
col[12][ _thunder]: [0 0]
col[13][ _tornado]: [0 0]
col[14][ _vism]: [(null) (null)]
col[15][ _wdird]: [0 0]
col[16][ _wdire]: ["North" "North"]
col[17][ _wgustm]: [(null) (null)]
col[18][ _windchillm]: [(null) (null)]
col[19][ _wspdm]: [(null) (null)]
这次12行数据都被成功读取出来了!
接下来,我们再来读取一下完整数据集testset.csv,我们通过输出读取的数据集行数来判断一下读取是否完全成功:
// read_csv_rows_count.go
func read(data io.ReadCloser) error {
var total int64
// read 10000 lines at a time to create record batches
rdr := csv.NewInferringReader(data, csv.WithChunk(10000),
// strings can be null, and these are the values
// to consider as null
csv.WithNullReader(true, "", "null", "[]"),
// assume the first line is a header line which names the columns
csv.WithHeader(true),
csv.WithColumnTypes(map[string]arrow.DataType{
" _vism": arrow.PrimitiveTypes.Float64,
}),
)
for rdr.Next() {
rec := rdr.Record()
total += rec.NumRows()
}
fmt.Println("total columns =", total)
return nil
}
我们开着错误输出的调试语句,看看上面的代码的输出结果:
======nextn: strconv.ParseInt: parsing "N/A": invalid syntax
total columns = 10000
我们看到上述程序仅读取了1w条记录,并输出了一个错误信息:CSV文件中包含“N/A”字样的数据,导致CSV Reader读取失败。经过数据对比核查,发现hum的数据存在大量“N/A”,另外pressurem的类型也有问题。那么如何解决这个问题呢?NewInferringReader提供了WithIncludeColumns功能选项可以供我们提供我们想要的列,这样我们可以给出一个列白名单,将hum列排除在外。修改后的read代码如下:
// read_csv_rows_count_with_col_filter.go
func read(data io.ReadCloser) error {
var total int64
// read 10000 lines at a time to create record batches
rdr := csv.NewInferringReader(data, csv.WithChunk(10000),
// strings can be null, and these are the values
// to consider as null
csv.WithNullReader(true, "", "null", "[]"),
// assume the first line is a header line which names the columns
csv.WithHeader(true),
csv.WithColumnTypes(map[string]arrow.DataType{
" _pressurem": arrow.PrimitiveTypes.Float64,
}),
csv.WithIncludeColumns([]string{
"datetime_utc", // 19961101-11:00
" _conds", // Smoke、Haze
" _fog", // 0
" _heatindexm",
" _pressurem", //
" _rain", //
" _snow", //
" _tempm", //
" _thunder", //
" _tornado", //
}),
)
for rdr.Next() {
rec := rdr.Record()
total += rec.NumRows()
}
fmt.Println("total columns =", total)
return nil
}
运行修改后的代码:
$go run read_csv_rows_count_with_col_filter.go
total columns = 100990
我们顺利将CSV中的数据读到了内存中,并组织成了多个Record Batch。
2. Arrow compute API介绍
一旦内存中有了Arrow格式的数据后,我们就可以基于这份数据进行数据操作了,比如过滤、查询、计算、转换等等。那么是否需要开发人员自己根据对Arrow type的结构的理解来实现针对这些数据操作的算法呢?不用的!
Arrow社区提供了compute API[9]以及各种语言的高性能实现以供基于Arrow格式进行数据操作的开发人员直接复用。
Go Arrow实现也提供了compute包用于操作内存中的Arrow object。不过根据compute包的注释来看,目前Go compute包还属于实验性质,并非stable的API,将来可能有变:
// The overwhemling majority of things in this package require go1.18 as
// it utilizes generics. The files in this package and its sub-packages
// are all excluded from being built by go versions lower than 1.18 so
// that the larger Arrow module itself is still compatible with go1.17.
//
// Everything in this package should be considered Experimental for now.
package compute
另外我们从上面注释也可以看到,compute包用到了泛型,因此需要Go 1.18及以后版本才能使用。
为了更好地理解compute API,我们需要知道一些有关compute的概念,首先了解一下Datum。
2.1 Datum
compute API中的函数需要支持多种类型数据作为输入,可以是arrow的array type,也可以是一个标量值(scalar),为了统一输出表示,compute API建立了一个名为Datum的抽象。Datum可以理解为一个compute API函数可以接受的各种arrow类型的union类型,union中既可以是一个scalar(标量值),也可以是Array、Chunked Array,甚至是一整个Record Batch或一个Arrow Table。
不出预料,Go中采用接口来建立Datum这个抽象:
// Datum is a variant interface for wrapping the various Arrow data structures
// for now the various Datum types just hold a Value which is the type they
// are wrapping, but it might make sense in the future for those types
// to actually be aliases or embed their types instead. Not sure yet.
type Datum interface {
fmt.Stringer
Kind() DatumKind
Len() int64
Equals(Datum) bool
Release()
data() any
}
Datum支持的类型通过DatumKind的常量枚举值可以看出:
// DatumKind is an enum used for denoting which kind of type a datum is encapsulating
type DatumKind int
const (
KindNone DatumKind = iota // none
KindScalar // scalar
KindArray // array
KindChunked // chunked_array
KindRecord // record_batch
KindTable // table
)
2.2 Function Type
compute包提供的是协助数据操作和分析的函数,这些函数可以被分为几类,我们由简单到复制的顺序逐一看一下:
2.2.1 标量(scalar)函数或逐元素(element-wise)函数
这类函数接受一个scalar参数或一个array类型的datum参数,函数会对输入参数中的逐个元素进行操作,比如求反、求绝对值等。如果传入的是scalar,则返回scalar,如果传入的是array类型,则返回array类型。传入和返回的array长度应相同。
下图(来自《In-Memory Analytics with Apache Arrow》[10]一书)直观地解释了这类函数的操作特性:
我们用go代码实现一下上图中的两个示例,先来看unary element-wise操作的例子:
// unary_elementwise_function.go
func main() {
data := []int32{5, 10, 0, 25, 2}
bldr := array.NewInt32Builder(memory.DefaultAllocator)
defer bldr.Release()
bldr.AppendValues(data, nil)
arr := bldr.NewArray()
defer arr.Release()
dat, err := compute.Negate(context.Background(), compute.ArithmeticOptions{}, compute.NewDatum(arr))
if err != nil {
fmt.Println(err)
return
}
arr1, ok := dat.(*compute.ArrayDatum)
if !ok {
fmt.Println("type assert fail")
return
}
fmt.Println(arr1.MakeArray()) // [-5 -10 0 -25 -2]
}
compute包实现了常见的一元和二元arithmetic函数:
下面是二元Add操作的示例:
// binary_elementwise_function.go
func main() {
data1 := []int32{5, 10, 0, 25, 2}
data2 := []int32{1, 5, 2, 10, 5}
scalarData1 := int32(6)
bldr := array.NewInt32Builder(memory.DefaultAllocator)
defer bldr.Release()
bldr.AppendValues(data1, nil)
arr1 := bldr.NewArray()
defer arr1.Release()
bldr.AppendValues(data2, nil)
arr2 := bldr.NewArray()
defer arr2.Release()
result1, err := compute.Add(context.Background(), compute.ArithmeticOptions{},
compute.NewDatum(arr1),
compute.NewDatum(arr2))
if err != nil {
fmt.Println(err)
return
}
result2, err := compute.Add(context.Background(), compute.ArithmeticOptions{},
compute.NewDatum(arr1),
compute.NewDatum(scalarData1))
if err != nil {
fmt.Println(err)
return
}
resultArr1, ok := result1.(*compute.ArrayDatum)
if !ok {
fmt.Println("type assert fail")
return
}
fmt.Println(resultArr1.MakeArray()) // [6 15 2 35 7]
resultArr2, ok := result2.(*compute.ArrayDatum)
if !ok {
fmt.Println("type assert fail")
return
}
fmt.Println(resultArr2.MakeArray()) // [11 16 6 31 8]
}
在这个示例里,我们实现了array + array和array + scalar两个操作,两个加法操作的结果都是一个新array。
接下来我们来看
2.2.2 array-wise(逐array)函数
这一类的函数使用整个array进行操作,经常进行转换或输出与输入array不同长度的结果。下图(来自《In-Memory Analytics with Apache Arrow》[11]一书)直观地解释了这类函数的操作特性:
Go compute包没有提供sort_unique函数,这里用Unique模拟一个unary array-wise操作,代码如下:
// unary_arraywise_function.go
func main() {
data := []int32{5, 10, 0, 25, 2, 10, 2, 25}
bldr := array.NewInt32Builder(memory.DefaultAllocator)
defer bldr.Release()
bldr.AppendValues(data, nil)
arr := bldr.NewArray()
defer arr.Release()
dat, err := compute.Unique(context.Background(), compute.NewDatum(arr))
if err != nil {
fmt.Println(err)
return
}
arr1, ok := dat.(*compute.ArrayDatum)
if !ok {
fmt.Println("type assert fail")
return
}
fmt.Println(arr1.MakeArray()) // [5 10 0 25 2]
}
而上图中的二元array-wise Filter操作可以由下面代码实现:
// binary_arraywise_function.go
func main() {
data := []int32{5, 10, 0, 25, 2}
filterMask := []bool{true, false, true, false, true}
bldr := array.NewInt32Builder(memory.DefaultAllocator)
defer bldr.Release()
bldr.AppendValues(data, nil)
arr := bldr.NewArray()
defer arr.Release()
bldr1 := array.NewBooleanBuilder(memory.DefaultAllocator)
defer bldr1.Release()
bldr1.AppendValues(filterMask, nil)
filterArr := bldr1.NewArray()
defer filterArr.Release()
dat, err := compute.Filter(context.Background(), compute.NewDatum(arr),
compute.NewDatum(filterArr),
compute.FilterOptions{})
if err != nil {
fmt.Println(err)
return
}
arr1, ok := dat.(*compute.ArrayDatum)
if !ok {
fmt.Println("type assert fail")
return
}
fmt.Println(arr1.MakeArray()) // [5 0 2]
}
注意:compute.Filter函数要求传入的value datum和filter datum的底层array长度要相同。
2.2.3 聚合(Aggregation)函数
Arrow compute支持两类聚合函数,一类是标量聚合(scalar aggregation),它的操作对象为一个array或一个标量,计算后输出一个标量值,常见的例子包括:count、min、max、mean、avg、sum等聚合计算;另外一类则是分组聚合(grouped aggregation),即先按某些“key”列进行分组后,再分别聚合,有些类似SQL中的group by操作。下图(来自《In-Memory Analytics with Apache Arrow》[12]一书)直观地解释了这两类函数的操作特性:
不过遗憾的是Go尚未提供对这类聚合函数的直接支持[13]。
要想实现上述十分有用的聚合数据操作,在官方尚未提供支持之前,我们可以考虑自行扩展compute包。
注:相对完整的标量聚合和分组聚合的函数列表,可以参考C++版本的API ref[14]。
3. 小结
鉴于本篇篇幅以及Go对聚合函数的尚未支持,计划中对Delhi CSV文件的聚合分析只能留到后面系列文章了。
简单回顾一下本文内容。我们介绍了Go Arrow实现从CSV文件读取数据的方法以及一些技巧,然后我们介绍了Arrow除了其format之外的一个重点内容:compute API,这为基于arrow的array数据进行数据操作提供了开箱即用和高性能的API,大家要理解其中Datum的抽象概念,以及各类Function的操作对象和返回结果类型。
注:本文涉及的源代码在这里[15]可以下载。
4. 参考资料
Make Data Files Easier to Work With Using Golang and Apache Arrow[16] - https://voltrondata.com/resources/make-data-files-easier-to-work-with-golang-arrow
《In-Memory Analytics with Apache Arrow》- https://book.douban.com/subject/35954154/
C++ compute API - https://arrow.apache.org/docs/cpp/compute.html
C++ Acero高级API - https://arrow.apache.org/docs/cpp/streaming_execution.html
“Gopher部落”知识星球[17]旨在打造一个精品Go学习和进阶社群!高品质首发Go技术文章,“三天”首发阅读权,每年两期Go语言发展现状分析,每天提前1小时阅读到新鲜的Gopher日报,网课、技术专栏、图书内容前瞻,六小时内必答保证等满足你关于Go语言生态的所有需求!2023年,Gopher部落将进一步聚焦于如何编写雅、地道、可读、可测试的Go代码,关注代码质量并深入理解Go核心技术,并继续加强与星友的互动。欢迎大家加入!
著名云主机服务厂商DigitalOcean发布最新的主机计划,入门级Droplet配置升级为:1 core CPU、1G内存、25G高速SSD,价格5$/月。有使用DigitalOcean需求的朋友,可以打开这个链接地址[18]:https://m.do.co/c/bff6eed92687 开启你的DO主机之路。
Gopher Daily(Gopher每日新闻)归档仓库 - https://github.com/bigwhite/gopherdaily
我的联系方式:
微博(暂不可用):https://weibo.com/bigwhite20xx
微博2:https://weibo.com/u/6484441286
博客:tonybai.com
github: https://github.com/bigwhite
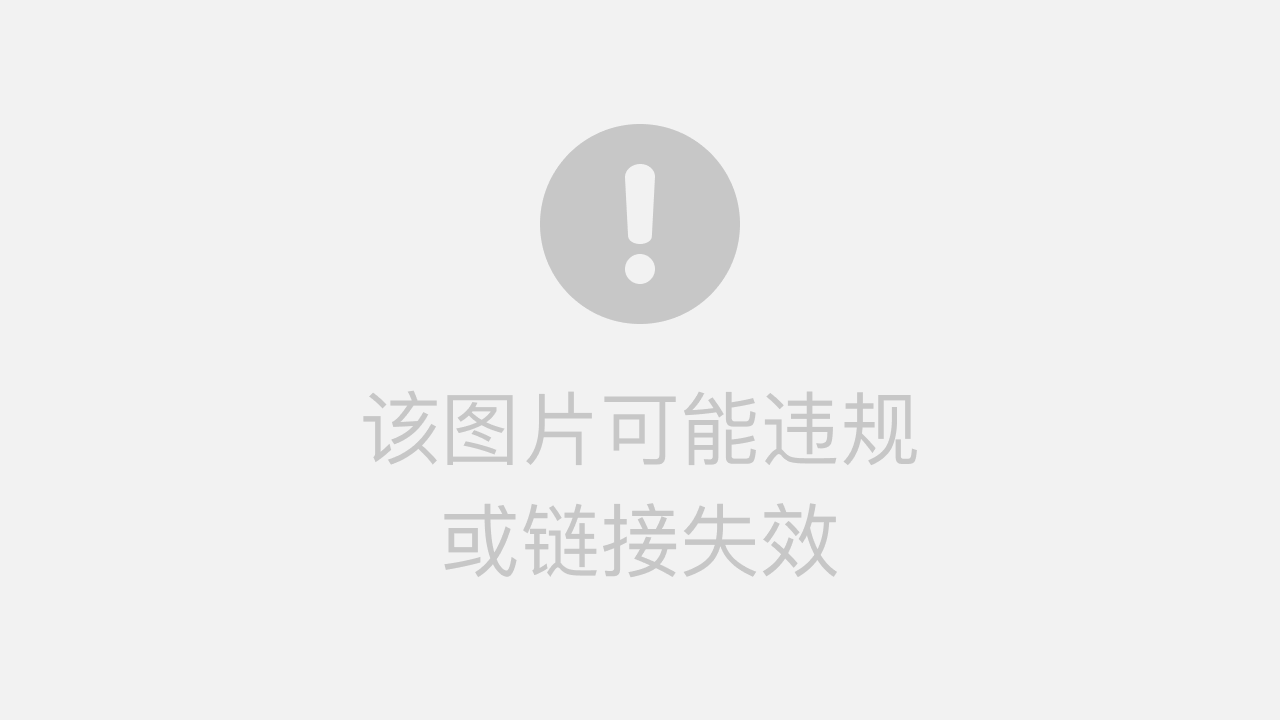
商务合作方式:撰稿、出书、培训、在线课程、合伙创业、咨询、广告合作。
参考资料
[1]
Arrow的基础数据类型: https://tonybai.com/2023/06/25/a-guide-of-using-apache-arrow-for-gopher-part1
[2]高级数据类型: https://tonybai.com/2023/07/08/a-guide-of-using-apache-arrow-for-gopher-part3
[3]Python: https://arrow.apache.org/cookbook/py
[4]Parquet: https://parquet.apache.org/
[5]Kaggle平台上的开放数据集: https://www.kaggle.com/datasets
[6]CSV文件: https://www.kaggle.com/datasets/mahirkukreja/delhi-weather-data
[7]“高级数据结构”: https://tonybai.com/2023/07/08/a-guide-of-using-apache-arrow-for-gopher-part3
[8]go.work: https://tonybai.com/2021/11/12/go-workspace-mode-in-go-1-18
[9]compute API: https://arrow.apache.org/docs/cpp/api/compute.html
[10]《In-Memory Analytics with Apache Arrow》: https://book.douban.com/subject/35954154/
[11]《In-Memory Analytics with Apache Arrow》: https://book.douban.com/subject/35954154/
[12]《In-Memory Analytics with Apache Arrow》: https://book.douban.com/subject/35954154/
[13]Go尚未提供对这类聚合函数的直接支持: https://github.com/apache/arrow/issues/32545
[14]参考C++版本的API ref: https://arrow.apache.org/docs/cpp/compute.html#aggregations
[15]这里: https://github.com/bigwhite/experiments/blob/master/arrow/manipulation
[16]Make Data Files Easier to Work With Using Golang and Apache Arrow: https://voltrondata.com/resources/make-data-files-easier-to-work-with-golang-arrow
[17]“Gopher部落”知识星球: https://wx.zsxq.com/dweb2/index/group/51284458844544
[18]链接地址: https://m.do.co/c/bff6eed92687