一、前言
众所周知,深度学习是一个"黑盒"系统。它通过“end-to-end”的方式来工作,输入数据例如RGB图像,输出目标例如类别标签、回归值等,中间过程不可得知。如何才能打开“黑盒”,一探究竟,让“黑盒”变成“灰盒”,甚至“白盒”?因此就有了“深度学习可解释性“这一领域,而特征可视化技术就是其中之一,其利用可视化的特征来探究深度卷积神经网络的工作机制和判断依据。本文从以下三方面来论述当前常用的特征可视化技术,并附带代码解析(pytorch)。
(1)特征图可视化
特征图可视化有两类方法,一类是直接将某一层的feature map映射到0-255的范围,变成图像。另一类是使用一个预训练的反卷积网络(反卷积、反池化)将feature map变成图像,从而达到可视化feature map的目的。
(2)卷积核可视化
我们知道,卷积的过程就是特征提取的过程,每一个卷积核代表着一种特征。如果图像中某块区域与某个卷积核的结果越大,那么该区域就越“像”该卷积核。基于以上的推论,如果我们找到一张图像,能够使得这张图像对某个卷积核的输出最大,那么我们就说找到了该卷积核最感兴趣的图像。
(3)类别激活可视化(Class Activation Mapping,CAM)
CAM(Class Activation Mapping,类别激活映射图),亦称为类别热力图或显著性图。它的大小与原图一致,像素值表示原始图片的对应区域对预测输出的影响程度,值越大贡献越大。目前常用的CAM系列包括:CAM、Grad-CAM、Grad-CAM++。
(4)注意力特征可视化
与CAM类似,只不过每个特征图所占权重来自于注意力,而不是最后层的全连接,基于注意力的特征可视化方法近年有比较多的研究,我们将在下一篇统一总结。
(5)一些技术工具
tensorflow框架提供了模型和特征可视化的工具tensorboard,可使用pytorch框架直接引入。
from torch.utils.tensorboard import SummaryWriter
更多使用细节参考https://zhuanlan.zhihu.com/p/60753993
二、特征图可视化
1. 最通用直接的特征可视化方法
思想很简单,想要哪一层的特征图直接提取那一层的输出即可,并将那一层的特征图可视化即可。注意输出的是灰度图像,所以针对多通道情况下直接可视化所有通道的特征图,而不进行额外处理。
直接上代码:
importtorchfromtorchvisionimportmodels,transformsfromPILimportImageimportmatplotlib.pyplotaspltimportnumpyasnpimportscipy.misc# 导入数据defget_image_info(image_dir):# 以RGB格式打开图像# Pytorch DataLoader就是使用PIL所读取的图像格式# 建议就用这种方法读取图像,当读入灰度图像时convert('')image_info=Image.open(image_dir).convert('RGB')# 数据预处理方法image_transform=transforms.Compose([transforms.Resize(256),transforms.CenterCrop(224),transforms.ToTensor(),transforms.Normalize([0.485,0.456,0.406],[0.229,0.224,0.225])])image_info=image_transform(image_info)image_info=image_info.unsqueeze(0)returnimage_info# 获取第k层的特征图defget_k_layer_feature_map(feature_extractor,k,x):withtorch.no_grad():forindex,layerinenumerate(feature_extractor):x=layer(x)ifk==index:returnx# 可视化特征图defshow_feature_map(feature_map):feature_map=feature_map.squeeze(0)feature_map=feature_map.cpu().numpy()feature_map_num=feature_map.shape[0]row_num=np.ceil(np.sqrt(feature_map_num))plt.figure()forindexinrange(1,feature_map_num+1):plt.subplot(row_num,row_num,index)plt.imshow(feature_map[index-1],cmap='gray')plt.axis('off')scipy.misc.imsave(str(index)+".png",feature_map[index-1])plt.show()if__name__=='__main__':# 初始化图像的路径image_dir=r"husky.png"# 定义提取第几层的feature mapk=1# 导入Pytorch封装的AlexNet网络模型model=models.alexnet(pretrained=True)# 是否使用gpu运算use_gpu=torch.cuda.is_available()use_gpu=False# 读取图像信息image_info=get_image_info(image_dir)# 判断是否使用gpuifuse_gpu:model=model.cuda()image_info=image_info.cuda()# alexnet只有features部分有特征图# classifier部分的feature map是向量feature_extractor=model.featuresfeature_map=get_k_layer_feature_map(feature_extractor,k,image_info)show_feature_map(feature_map)
2. 反卷积可视化特征
对于高维特征图,特征图数量(通道数)远大于3,因此很难一灰度图或者RGB图像的形式可视,与1中直接单独显示每个通道的特征图不同,反卷积可以直接将高维特征图转换为低维图像,并且还原图像尺寸。
如下图所示,反卷积网络的用途是对一个训练好的神经网络中任意一层feature map经过反卷积网络后重构出像素空间,主要操作是反池化unpooling、修正rectify、滤波filter,换句话说就是反池化,反激活,反卷积。

由于不可能获取标签数据,因此反卷积网络是一个无监督的,不具备学习能力的,就像一个训练好的网络的检测器,或者说是一个复杂的映射函数。
更多实现细节可参考文章《Visualizing and Understanding Convolutional Networks》
还有改进版的导向反向传播《Striving for Simplicity:The All Convolutional Net》
上述两种方法对特征图可视化有个明显的不足,即无法可视化图像中哪些区域对识别具体某个类别的作用,这个主要是使用CAM系列的方法,会在第四章中介绍。下面将介绍可视化卷积核的方法。
三、卷积核可视化
卷积核到底是如何识别物体的呢?想要解决这个问题,有一个方法就是去了解卷积核最感兴趣的图像是怎样的。我们知道,卷积的过程就是特征提取的过程,每一个卷积核代表着一种特征。如果图像中某块区域与某个卷积核的结果越大,那么该区域就越“像”该卷积核。基于以上的推论,如果我们找到一张图像,能够使得这张图像对某个卷积核的输出最大,那么我们就说找到了该卷积核最感兴趣的图像。
具体思路:从一张带有随机噪声的图像开始,每个像素值随机选取一种颜色。接下来,我们使用这张噪声图作为CNN网络的输入向前传播,然后取得其在网络中第 i 层 j 个卷积核的激活 a_ij(x),然后做一个反向传播计算的梯度 G=∂F/∂I,目标是希望通过改变每个像素的颜色值以增加对该卷积核的激活,用梯度上升的方法迭代更新图像 I=I+η∗G,η是类似于学习率的东西。
#使用keras可视化卷积核代码
import numpy as np
import tensorflow as tf
from tensorflow import keras
# The dimensions of our input image
img_width = 180
img_height = 180
# Our target layer: we will visualize the filters from this layer.
# See `model.summary()` for list of layer names, if you want to change this.
layer_name = "conv3_block4_out"
# Build a ResNet50V2 model loaded with pre-trained ImageNet weights
model = keras.applications.ResNet50V2(weights="imagenet", include_top=False)
# Set up a model that returns the activation values for our target layerlayer = model.get_layer(name=layer_name)
feature_extractor = keras.Model(inputs=model.inputs, outputs=layer.output)
# loss函数取最大化指定卷积核的响应值的平均值
def compute_loss(input_image, filter_index):
activation = feature_extractor(input_image)
# We avoid border artifacts by only involving non-border pixels in the loss.
filter_activation = activation[:, 2:-2, 2:-2, filter_index]
return tf.reduce_mean(filter_activation)
@tf.function
def gradient_ascent_step(img, filter_index, learning_rate):
with tf.GradientTape() as tape:
tape.watch(img)
loss = compute_loss(img, filter_index)
# Compute gradients.
grads = tape.gradient(loss, img)
# Normalize gradients.
grads = tf.math.l2_normalize(grads)
img += learning_rate * grads
return loss, img
def initialize_image():
# We start from a gray image with some random noise
img = tf.random.uniform((1, img_width, img_height, 3))
# ResNet50V2 expects inputs in the range [-1, +1].
# Here we scale our random inputs to [-0.125, +0.125]
return (img - 0.5) * 0.25
def visualize_filter(filter_index):
# We run gradient ascent for 20 steps
iterations = 30
learning_rate = 10.0
img = initialize_image()
for iteration in range(iterations):
loss, img = gradient_ascent_step(img, filter_index, learning_rate)
# Decode the resulting input image
img = deprocess_image(img[0].numpy())
return loss, img
def deprocess_image(img):
# Normalize array: center on 0., ensure variance is 0.15
img -= img.mean()
img /= img.std() + 1e-5
img *= 0.15
# Center crop
img = img[25:-25, 25:-25, :]
# Clip to [0, 1]
img += 0.5
img = np.clip(img, 0, 1)
# Convert to RGB array
img *= 255
img = np.clip(img, 0, 255).astype("uint8")
return img
代码中,使用训练好的VGG16模型,可视化该模型的卷积核。结果如下

代码与可视化图的参考链接
How convolutional neural networks see the world
http://www.51zixue.net/deeplearning/746.html
四、类别激活可视化CAM
CAM全称Class Activation Mapping,既类别激活映射图,也被称为类别热力图、显著性图等。是一张和原始图片等同大小图,该图片上每个位置的像素取值范围从0到1,一般用0到255的灰度图表示。可以理解为对预测输出的贡献分布,分数越高的地方表示原始图片对应区域对网络的响应越高、贡献越大。常用的CAM方法有:

1. CAM
一个深层的卷积神经网络而言,通过多次卷积和池化以后,它的最后一层卷积层包含了最丰富的空间和语义信息,再往下就是全连接层和softmax层了,其中所包含的信息都是人类难以理解的,很难以可视化的方式展示出来。所以说,要让卷积神经网络的对其分类结果给出一个合理解释,必须要充分利用好最后一个卷积层。

如上图所示,CAM的结构由CNN特征提取网络,全局平均池化GAP,全连接层和Softmax组成。一张图片在经过CNN特征提取网络后得到feature maps, 再对每一个feature map进行全局平均池化,变成一维向量,再经过全连接层与softmax得到类的概率。假定在GAP前是n个通道,则经过GAP后得到的是一个长度为1x n的向量,假定类别数为m,则全连接层的权值为一个n x m的张量。(注:这里先忽视batch-size)。对于某一个类别C, 现在想要可视化这个模型对于识别类别C,原图像的哪些区域起主要作用,换句话说模型是根据哪些信息得到该图像就是类别C。
做法是取出全连接层中得到类别C的概率的那一维权值,用W表示,即上图的下半部分。然后对GAP前的feature map进行加权求和,由于此时feature map不是原图像大小,在加权求和后还需要进行上采样,即可得到Class Activation Map。
用公式表示如下:(k表示通道,c表示类别,fk(x,y)表示feature map)
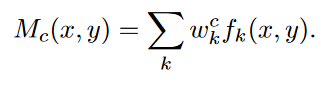
参考核心代码:
class CAM(_CAM):
"""Implements a class activation map extractor as described in `"Learning Deep Features for Discriminative
Localization" <https://arxiv.org/pdf/1512.04150.pdf>`_.
The Class Activation Map (CAM) is defined for image classification models that have global pooling at the end
of the visual feature extraction block. The localization map is computed as follows:
.. math::
L^{(c)}_{CAM}(x, y) = ReLU\\Big(\\sum\\limits_k w_k^{(c)} A_k(x, y)\\Big)
where :math:`A_k(x, y)` is the activation of node :math:`k` in the target layer of the model at
position :math:`(x, y)`,
and :math:`w_k^{(c)}` is the weight corresponding to class :math:`c` for unit :math:`k` in the fully
connected layer..
Example::
>>> from torchvision.models import resnet18
>>> from torchcam.cams import CAM
>>> model = resnet18(pretrained=True).eval()
>>> cam = CAM(model, 'layer4', 'fc')
>>> with torch.no_grad(): out = model(input_tensor)
>>> cam(class_idx=100)
Args:
model: input model
target_layer: name of the target layer
fc_layer: name of the fully convolutional layer
input_shape: shape of the expected input tensor excluding the batch dimension
"""
def __init__(
self,
model: nn.Module,
target_layer: Optional[str] = None,
fc_layer: Optional[str] = None,
input_shape: Tuple[int, ...] = (3, 224, 224),
**kwargs: Any,
) -> None:
super().__init__(model, target_layer, input_shape, **kwargs)
# If the layer is not specified, try automatic resolution
if fc_layer is None:
fc_layer = locate_linear_layer(model)
# Warn the user of the choice
if isinstance(fc_layer, str):
logging.warning(f"no value was provided for `fc_layer`, thus set to '{fc_layer}'.")
else:
raise ValueError("unable to resolve `fc_layer` automatically, please specify its value.")
# Softmax weight
self._fc_weights = self.submodule_dict[fc_layer].weight.data
# squeeze to accomodate replacement by Conv1x1
if self._fc_weights.ndim > 2:
self._fc_weights = self._fc_weights.view(*self._fc_weights.shape[:2])
def _get_weights(self, class_idx: int, scores: Optional[Tensor] = None) -> Tensor:
"""Computes the weight coefficients of the hooked activation maps"""
# Take the FC weights of the target class
return self._fc_weights[class_idx, :]
2. Grad-CAM
利用 GAP 获取 CAM 的方式有它的局限性:
1)要求模型必须有 GAP 层;
2)只能提取最后一层特征图的热力图。
Grad-CAM 是为了克服上面的缺陷而提出的,Grad-CAM的最大特点就是不再需要修改现有的模型结构了,也不需要重新训练了,直接在原模型上即可可视化,可提取任意层的热力图。
原理:Grad-CAM根据输出向量,进行backward,求取特征图的梯度,得到每个特征图上每个像素点对应的梯度,也就是特征图对应的梯度图,然后再对每个梯度图求平均,这个平均值就对应于每个特征图的权重,然后再将权重与特征图进行加权求和,最后经过relu激活函数就可以得到最终的类激活图。
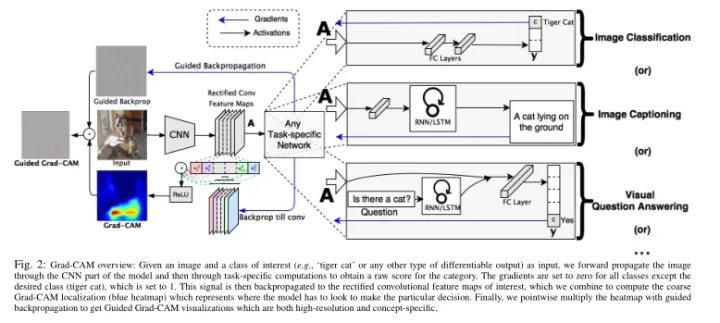
参考核心代码:
class _GradCAM(_CAM):
"""Implements a gradient-based class activation map extractor
Args:
model: input model
target_layer: name of the target layer
input_shape: shape of the expected input tensor excluding the batch dimension
"""
def __init__(
self,
model: torch.nn.Module,
target_layer: Optional[str] = None,
input_shape: Tuple[int, ...] = (3, 224, 224),
**kwargs: Any,
) -> None:
super().__init__(model, target_layer, input_shape, **kwargs)
# Init hook
self.hook_g: Optional[Tensor] = None
# Ensure ReLU is applied before normalization
self._relu = True
# Model output is used by the extractor
self._score_used = True
# Trick to avoid issues with inplace operations cf. https://github.com/pytorch/pytorch/issues/61519
self.hook_handles.append(self.submodule_dict[self.target_layer].register_forward_hook(self._hook_g))
def _store_grad(self, grad: Tensor) -> None:
if self._hooks_enabled:
self.hook_g = grad.data
def _hook_g(self, module: torch.nn.Module, input: Tensor, output: Tensor) -> None:
"""Gradient hook"""
if self._hooks_enabled:
self.hook_handles.append(output.register_hook(self._store_grad))
def _backprop(self, scores: Tensor, class_idx: int) -> None:
"""Backpropagate the loss for a specific output class"""
if self.hook_a is None:
raise TypeError("Inputs need to be forwarded in the model for the conv features to be hooked")
# Backpropagate to get the gradients on the hooked layer
loss = scores[:, class_idx].sum()
self.model.zero_grad()
loss.backward(retain_graph=True)
def _get_weights(self, class_idx, scores):
raise NotImplementedError
class GradCAM(_GradCAM):
"""Implements a class activation map extractor as described in `"Grad-CAM: Visual Explanations from Deep Networks
via Gradient-based Localization" <https://arxiv.org/pdf/1610.02391.pdf>`_.
The localization map is computed as follows:
.. math::
L^{(c)}_{Grad-CAM}(x, y) = ReLU\\Big(\\sum\\limits_k w_k^{(c)} A_k(x, y)\\Big)
with the coefficient :math:`w_k^{(c)}` being defined as:
.. math::
w_k^{(c)} = \\frac{1}{H \\cdot W} \\sum\\limits_{i=1}^H \\sum\\limits_{j=1}^W
\\frac{\\partial Y^{(c)}}{\\partial A_k(i, j)}
where :math:`A_k(x, y)` is the activation of node :math:`k` in the target layer of the model at
position :math:`(x, y)`,
and :math:`Y^{(c)}` is the model output score for class :math:`c` before softmax.
Example::
>>> from torchvision.models import resnet18
>>> from torchcam.cams import GradCAM
>>> model = resnet18(pretrained=True).eval()
>>> cam = GradCAM(model, 'layer4')
>>> scores = model(input_tensor)
>>> cam(class_idx=100, scores=scores)
Args:
model: input model
target_layer: name of the target layer
input_shape: shape of the expected input tensor excluding the batch dimension
"""
def _get_weights(self, class_idx: int, scores: Tensor) -> Tensor: # type: ignore[override]
"""Computes the weight coefficients of the hooked activation maps"""
self.hook_g: Tensor
# Backpropagate
self._backprop(scores, class_idx)
# Global average pool the gradients over spatial dimensions
return self.hook_g.squeeze(0).flatten(1).mean(-1)
3. Grad-CAM++
Grad-CAM是利用目标特征图的梯度求平均(GAP)获取特征图权重,可以看做梯度map上每一个元素的贡献是一样。为了得到更好的效果(特别是在某一分类的物体在图像中不止一个的情况下),Chattopadhyay等认为梯度map上的每一个元素的贡献不同,又进一步提出了Grad-CAM++,主要的变动是在对应于某个分类的特征映射的权重表示中加入了ReLU和权重梯度 :
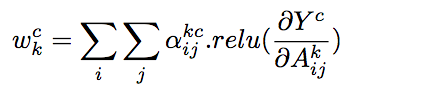
只需一次反向传播即可计算梯度:
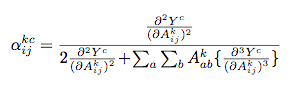
下图是CAM、Grad-CAM、Grad-CAM++架构对比:

核心代码展示:
class GradCAMpp(_GradCAM):
"""Implements a class activation map extractor as described in `"Grad-CAM++: Improved Visual Explanations for
Deep Convolutional Networks" <https://arxiv.org/pdf/1710.11063.pdf>`_.
The localization map is computed as follows:
.. math::
L^{(c)}_{Grad-CAM++}(x, y) = \\sum\\limits_k w_k^{(c)} A_k(x, y)
with the coefficient :math:`w_k^{(c)}` being defined as:
.. math::
w_k^{(c)} = \\sum\\limits_{i=1}^H \\sum\\limits_{j=1}^W \\alpha_k^{(c)}(i, j) \\cdot
ReLU\\Big(\\frac{\\partial Y^{(c)}}{\\partial A_k(i, j)}\\Big)
where :math:`A_k(x, y)` is the activation of node :math:`k` in the target layer of the model at
position :math:`(x, y)`,
:math:`Y^{(c)}` is the model output score for class :math:`c` before softmax,
and :math:`\\alpha_k^{(c)}(i, j)` being defined as:
.. math::
\\alpha_k^{(c)}(i, j) = \\frac{1}{\\sum\\limits_{i, j} \\frac{\\partial Y^{(c)}}{\\partial A_k(i, j)}}
= \\frac{\\frac{\\partial^2 Y^{(c)}}{(\\partial A_k(i,j))^2}}{2 \\cdot
\\frac{\\partial^2 Y^{(c)}}{(\\partial A_k(i,j))^2} + \\sum\\limits_{a,b} A_k (a,b) \\cdot
\\frac{\\partial^3 Y^{(c)}}{(\\partial A_k(i,j))^3}}
if :math:`\\frac{\\partial Y^{(c)}}{\\partial A_k(i, j)} = 1` else :math:`0`.
Example::
>>> from torchvision.models import resnet18
>>> from torchcam.cams import GradCAMpp
>>> model = resnet18(pretrained=True).eval()
>>> cam = GradCAMpp(model, 'layer4')
>>> scores = model(input_tensor)
>>> cam(class_idx=100, scores=scores)
Args:
model: input model
target_layer: name of the target layer
input_shape: shape of the expected input tensor excluding the batch dimension
"""
def _get_weights(self, class_idx: int, scores: Tensor) -> Tensor: # type: ignore[override]
"""Computes the weight coefficients of the hooked activation maps"""
self.hook_g: Tensor
# Backpropagate
self._backprop(scores, class_idx)
# Alpha coefficient for each pixel
grad_2 = self.hook_g.pow(2)
grad_3 = grad_2 * self.hook_g
# Watch out for NaNs produced by underflow
spatial_dims = self.hook_a.ndim - 2 # type: ignore[union-attr]
denom = 2 * grad_2 + (grad_3 * self.hook_a).flatten(2).sum(-1)[(...,) + (None,) * spatial_dims]
nan_mask = grad_2 > 0
alpha = grad_2
alpha[nan_mask].div_(denom[nan_mask])
# Apply pixel coefficient in each weight
return alpha.squeeze_(0).mul_(torch.relu(self.hook_g.squeeze(0))).flatten(1).sum(-1)
本节参考论文:
Deconvolution Visualizing and Understanding Convolutional Networks
Guided-backpropagation Striving for Simplicity: The All Convolutional Net
CAM Learning Deep Features for Discriminative Localization
Grad-CAM: Visual Explanations from Deep Networks via Gradient-based Localization
Yes, Deep Networks are great, but are they Trustworthy?
五、其他资料
pytorch 完整实现'CAM', 'ScoreCAM', 'SSCAM', 'ISCAM' 'GradCAM', 'GradCAMpp', 'SmoothGradCAMpp', 'XGradCAM', 'LayerCAM' :
https://github.com/ZhugeKongan/TorchCAMgithub.com/ZhugeKongan/TorchCAM