processScan函数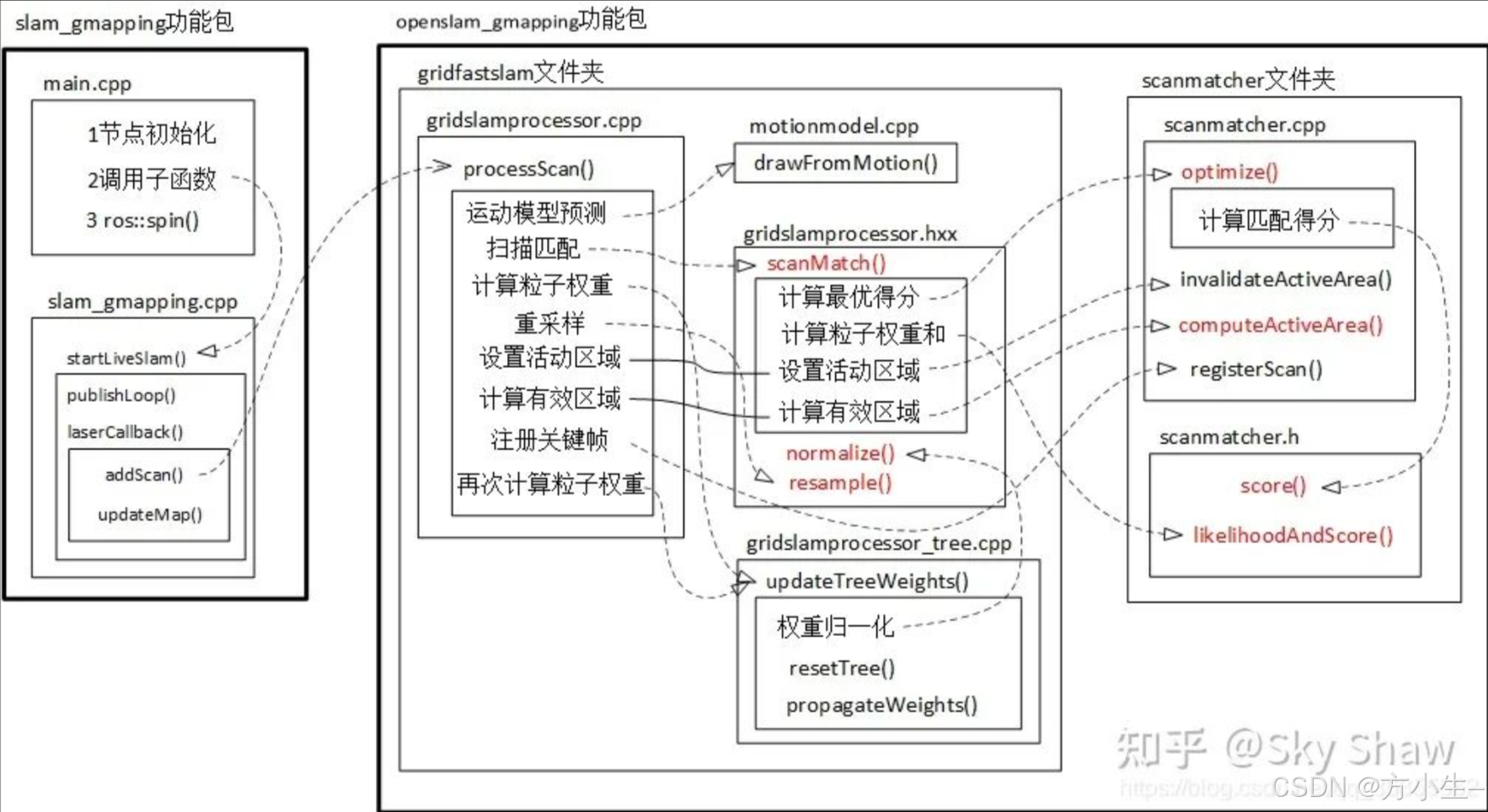
参考:https://blog.csdn.net/CV_Autobot/article/details/131058981
drawFromMotion
:根据运动模型更新粒子位姿
scanMatch
:进行扫描匹配
resample
:重采样
逐步分解并详细解释代码
1. 获取当前扫描的相对位姿
OrientedPoint relPose = reading.getPose(); // 获取当前扫描的相对位姿
if (!m_count){ // 如果是第一次处理扫描
m_lastPartPose = m_odoPose = relPose; // 初始化上一次粒子位姿和里程计位姿
}
- 从
reading
中获取当前扫描的相对位姿relPose
。 - 如果是第一次处理扫描(
m_count
为0),则初始化上一次粒子位姿m_lastPartPose
和里程计位姿m_odoPose
为relPose
。
2. 使用运动模型更新所有粒子
for (ParticleVector::iterator it = m_particles.begin(); it != m_particles.end(); it++){
OrientedPoint& pose(it->pose);
pose = m_motionModel.drawFromMotion(it->pose, relPose, m_odoPose); // 根据运动模型更新粒子位姿
}
- 遍历所有粒子,使用运动模型
m_motionModel
更新每个粒子的位姿。
3. 更新输出文件
if (m_outputStream.is_open()){
m_outputStream << setiosflags(ios::fixed) << setprecision(6);
m_outputStream << "ODOM ";
m_outputStream << setiosflags(ios::fixed) << setprecision(3) << m_odoPose.x << " " << m_odoPose.y << " ";
m_outputStream << setiosflags(ios::fixed) << setprecision(6) << m_odoPose.theta << " ";
m_outputStream << reading.getTime();
m_outputStream << endl;
}
if (m_outputStream.is_open()){
m_outputStream << setiosflags(ios::fixed) << setprecision(6);
m_outputStream << "ODO_UPDATE " << m_particles.size() << " ";
for (ParticleVector::iterator it = m_particles.begin(); it != m_particles.end(); it++){
OrientedPoint& pose(it->pose);
m_outputStream << setiosflags(ios::fixed) << setprecision(3) << pose.x << " " << pose.y << " ";
m_outputStream << setiosflags(ios::fixed) << setprecision(6) << pose.theta << " " << it-> weight << " ";
}
m_outputStream << reading.getTime();
m_outputStream << endl;
}
- 如果输出流
m_outputStream
是打开的,则将里程计信息和粒子更新信息写入输出文件。
4. 调用回调函数
onOdometryUpdate();
- 调用里程计更新回调函数
onOdometryUpdate()
。
5. 累积机器人的平移和旋转
OrientedPoint move = relPose - m_odoPose;
move.theta = atan2(sin(move.theta), cos(move.theta));
m_linearDistance += sqrt(move * move);
m_angularDistance += fabs(move.theta);
- 计算机器人从上一次里程计位姿到当前位姿的平移和旋转。
- 累积线性距离
m_linearDistance
和角度距离m_angularDistance
。
6. 检查机器人是否跳跃
if (m_linearDistance > m_distanceThresholdCheck){
cerr << "***********************************************************************" << endl;
cerr << "********** Error: m_distanceThresholdCheck overridden!!!! *************" << endl;
cerr << "m_distanceThresholdCheck=" << m_distanceThresholdCheck << endl;
cerr << "Old Odometry Pose= " << m_odoPose.x << " " << m_odoPose.y
<< " " << m_odoPose.theta << endl;
cerr << "New Odometry Pose (reported from observation)= " << relPose.x << " " << relPose.y
<< " " << relPose.theta << endl;
cerr << "***********************************************************************" << endl;
cerr << "** The Odometry has a big jump here. This is probably a bug in the **" << endl;
cerr << "** odometry/laser input. We continue now, but the result is probably **" << endl;
cerr << "** crap or can lead to a core dump since the map doesn't fit.... C&G **" << endl;
cerr << "***********************************************************************" << endl;
}
- 如果线性距离超过阈值
m_distanceThresholdCheck
,则输出警告信息。
7. 更新里程计位姿
m_odoPose = relPose; // 更新里程计位姿
- 更新里程计位姿
m_odoPose
为当前相对位姿relPose
。
8. 处理扫描
bool processed = false;
if (!m_count
|| m_linearDistance > m_linearThresholdDistance
|| m_angularDistance > m_angularThresholdDistance){
if (m_outputStream.is_open()){
m_outputStream << setiosflags(ios::fixed) << setprecision(6);
m_outputStream << "FRAME " << m_readingCount;
m_outputStream << " " << m_linearDistance;
m_outputStream << " " << m_angularDistance << endl;
}
if (m_infoStream)
m_infoStream << "update frame " << m_readingCount << endl
<< "update ld=" << m_linearDistance << " ad=" << m_angularDistance << endl;
cerr << "Laser Pose= " << reading.getPose().x << " " << reading.getPose().y
<< " " << reading.getPose().theta << endl;
assert(reading.size() == m_beams);
double * plainReading = new double[m_beams];
for(unsigned int i = 0; i < m_beams; i++){
plainReading[i] = reading[i];
}
m_infoStream << "m_count " << m_count << endl;
if (m_count > 0){
scanMatch(plainReading); // 进行扫描匹配
if (m_outputStream.is_open()){
m_outputStream << "LASER_READING " << reading.size() << " ";
m_outputStream << setiosflags(ios::fixed) << setprecision(2);
for (RangeReading::const_iterator b = reading.begin(); b != reading.end(); b++){
m_outputStream << *b << " ";
}
OrientedPoint p = reading.getPose();
m_outputStream << setiosflags(ios::fixed) << setprecision(6);
m_outputStream << p.x << " " << p.y << " " << p.theta << " " << reading.getTime() << endl;
m_outputStream << "SM_UPDATE " << m_particles.size() << " ";
for (ParticleVector::const_iterator it = m_particles.begin(); it != m_particles.end(); it++){
const OrientedPoint& pose = it->pose;
m_outputStream << setiosflags(ios::fixed) << setprecision(3) << pose.x << " " << pose.y << " ";
m_outputStream << setiosflags(ios::fixed) << setprecision(6) << pose.theta << " " << it-> weight << " ";
}
m_outputStream << endl;
}
onScanmatchUpdate(); // 调用扫描匹配更新回调
updateTreeWeights(false); // 更新树权重
if (m_infoStream){
m_infoStream << "neff= " << m_neff << endl;
}
if (m_outputStream.is_open()){
m_outputStream << setiosflags(ios::fixed) << setprecision(6);
m_outputStream << "NEFF " << m_neff << endl;
}
resample(plainReading, adaptParticles); // 重采样
} else {
m_infoStream << "Registering First Scan" << endl;
for (ParticleVector::iterator it = m_particles.begin(); it != m_particles.end(); it++){
m_matcher.invalidateActiveArea();
m_matcher.computeActiveArea(it->map, it->pose, plainReading);
m_matcher.registerScan(it->map, it->pose, plainReading);
TNode* node = new TNode(it->pose, 0., it->node, 0);
node->reading = 0;
it->node = node;
}
}
updateTreeWeights(false); // 更新树权重
delete [] plainReading; // 释放内存
m_lastPartPose = m_odoPose; // 更新上一次粒子位姿
m_linearDistance = 0; // 重置线性距离
m_angularDistance = 0; // 重置角度距离
m_count++; // 增加计数
processed = true; // 设置处理标志
for (ParticleVector::iterator it = m_particles.begin(); it != m_particles.end(); it++){
it->previousPose = it->pose;
}
}
if (m_outputStream.is_open())
m_outputStream << flush;
m_readingCount++; // 增加读取计数
return processed; // 返回处理标志
- 只有在机器人行驶了一定距离后才处理扫描。
- 将读取转换为扫描匹配器可用的形式。
- 如果是第一次处理扫描,则注册第一帧扫描。
- 进行扫描匹配,更新树权重,重采样。
- 更新上一次粒子位姿,重置线性距离和角度距离,增加计数。
- 返回处理标志。
总结代码
主要功能
- 初始化位姿:如果是第一次处理扫描,初始化上一次粒子位姿和里程计位姿。
- 更新粒子位姿:使用运动模型更新所有粒子的位姿。
- 输出信息:将里程计信息和粒子更新信息写入输出文件。
- 累积距离:累积机器人的平移和旋转距离。
- 检查跳跃:如果机器人跳跃则发出警告。
- 处理扫描:只有在机器人行驶了一定距离后才处理扫描,进行扫描匹配、更新树权重、重采样等操作。
- 返回处理标志:返回是否处理了当前扫描的标志。
该代码的主要功能是处理激光扫描数据,更新粒子滤波器中的粒子位姿,并根据机器人的运动情况进行扫描匹配和重采样,以实现SLAM(同时定位与地图构建)。
bool GridSlamProcessor::processScan(const RangeReading & reading, int adaptParticles){
/** 从读取中检索位置,并计算里程计 */
OrientedPoint relPose = reading.getPose(); // 获取当前扫描的相对位姿
if (!m_count){ // 如果是第一次处理扫描
m_lastPartPose = m_odoPose = relPose; // 初始化上一次粒子位姿和里程计位姿
}
// 将读取的状态写入并使用运动模型更新所有粒子
for (ParticleVector::iterator it = m_particles.begin(); it != m_particles.end(); it++){
OrientedPoint& pose(it->pose);
// it->pose:当前粒子位姿
// relPose:当前扫描的相对位姿
// m_odoPose:上一次粒子位姿
pose = m_motionModel.drawFromMotion(it->pose, relPose, m_odoPose); // 根据运动模型更新粒子位姿
}
// 更新输出文件
if (m_outputStream.is_open()){
m_outputStream << setiosflags(ios::fixed) << setprecision(6);
m_outputStream << "ODOM ";
m_outputStream << setiosflags(ios::fixed) << setprecision(3) << m_odoPose.x << " " << m_odoPose.y << " ";
m_outputStream << setiosflags(ios::fixed) << setprecision(6) << m_odoPose.theta << " ";
m_outputStream << reading.getTime();
m_outputStream << endl;
}
if (m_outputStream.is_open()){
m_outputStream << setiosflags(ios::fixed) << setprecision(6);
m_outputStream << "ODO_UPDATE " << m_particles.size() << " ";
for (ParticleVector::iterator it = m_particles.begin(); it != m_particles.end(); it++){
OrientedPoint& pose(it->pose);
m_outputStream << setiosflags(ios::fixed) << setprecision(3) << pose.x << " " << pose.y << " ";
m_outputStream << setiosflags(ios::fixed) << setprecision(6) << pose.theta << " " << it-> weight << " ";
}
m_outputStream << reading.getTime();
m_outputStream << endl;
}
// 调用回调函数
onOdometryUpdate();
// 累积机器人的平移和旋转
OrientedPoint move = relPose - m_odoPose;
move.theta = atan2(sin(move.theta), cos(move.theta));
m_linearDistance += sqrt(move * move);
m_angularDistance += fabs(move.theta);
// 如果机器人跳跃则发出警告
if (m_linearDistance > m_distanceThresholdCheck){
cerr << "***********************************************************************" << endl;
cerr << "********** Error: m_distanceThresholdCheck overridden!!!! *************" << endl;
cerr << "m_distanceThresholdCheck=" << m_distanceThresholdCheck << endl;
cerr << "Old Odometry Pose= " << m_odoPose.x << " " << m_odoPose.y
<< " " << m_odoPose.theta << endl;
cerr << "New Odometry Pose (reported from observation)= " << relPose.x << " " << relPose.y
<< " " << relPose.theta << endl;
cerr << "***********************************************************************" << endl;
cerr << "** The Odometry has a big jump here. This is probably a bug in the **" << endl;
cerr << "** odometry/laser input. We continue now, but the result is probably **" << endl;
cerr << "** crap or can lead to a core dump since the map doesn't fit.... C&G **" << endl;
cerr << "***********************************************************************" << endl;
}
m_odoPose = relPose; // 更新里程计位姿
bool processed = false;
// 只有在机器人行驶了一定距离后才处理扫描 更新距离和角度
if (!m_count
|| m_linearDistance > m_linearThresholdDistance
|| m_angularDistance > m_angularThresholdDistance){
if (m_outputStream.is_open()){
m_outputStream << setiosflags(ios::fixed) << setprecision(6);
m_outputStream << "FRAME " << m_readingCount;
m_outputStream << " " << m_linearDistance;
m_outputStream << " " << m_angularDistance << endl;
}
if (m_infoStream)
m_infoStream << "update frame " << m_readingCount << endl
<< "update ld=" << m_linearDistance << " ad=" << m_angularDistance << endl;
cerr << "Laser Pose= " << reading.getPose().x << " " << reading.getPose().y
<< " " << reading.getPose().theta << endl;
// 将读取转换为扫描匹配器可用的形式
assert(reading.size() == m_beams);
double * plainReading = new double[m_beams];
for(unsigned int i = 0; i < m_beams; i++){
plainReading[i] = reading[i];
}
m_infoStream << "m_count " << m_count << endl;
if (m_count > 0){
scanMatch(plainReading); // 进行扫描匹配
if (m_outputStream.is_open()){
m_outputStream << "LASER_READING " << reading.size() << " ";
m_outputStream << setiosflags(ios::fixed) << setprecision(2);
for (RangeReading::const_iterator b = reading.begin(); b != reading.end(); b++){
m_outputStream << *b << " ";
}
OrientedPoint p = reading.getPose();
m_outputStream << setiosflags(ios::fixed) << setprecision(6);
m_outputStream << p.x << " " << p.y << " " << p.theta << " " << reading.getTime() << endl;
m_outputStream << "SM_UPDATE " << m_particles.size() << " ";
for (ParticleVector::const_iterator it = m_particles.begin(); it != m_particles.end(); it++){
const OrientedPoint& pose = it->pose;
m_outputStream << setiosflags(ios::fixed) << setprecision(3) << pose.x << " " << pose.y << " ";
m_outputStream << setiosflags(ios::fixed) << setprecision(6) << pose.theta << " " << it-> weight << " ";
}
m_outputStream << endl;
}
onScanmatchUpdate(); // 调用扫描匹配更新回调
updateTreeWeights(false); // 更新树权重
if (m_infoStream){
m_infoStream << "neff= " << m_neff << endl;
}
if (m_outputStream.is_open()){
m_outputStream << setiosflags(ios::fixed) << setprecision(6);
m_outputStream << "NEFF " << m_neff << endl;
}
resample(plainReading, adaptParticles); // 重采样
} else {
m_infoStream << "Registering First Scan" << endl;
for (ParticleVector::iterator it = m_particles.begin(); it != m_particles.end(); it++){
m_matcher.invalidateActiveArea();
m_matcher.computeActiveArea(it->map, it->pose, plainReading);
m_matcher.registerScan(it->map, it->pose, plainReading);
// cyr: 不再需要,粒子一开始就指向根节点!
TNode* node = new TNode(it->pose, 0., it->node, 0);
node->reading = 0;
it->node = node;
}
}
// 更新树权重
updateTreeWeights(false);
delete [] plainReading; // 释放内存
m_lastPartPose = m_odoPose; // 更新上一次粒子位姿
m_linearDistance = 0; // 重置线性距离
m_angularDistance = 0; // 重置角度距离
m_count++; // 增加计数
processed = true; // 设置处理标志
// 为下一次迭代准备
for (ParticleVector::iterator it = m_particles.begin(); it != m_particles.end(); it++){
it->previousPose = it->pose;
}
}
if (m_outputStream.is_open())
m_outputStream << flush;
m_readingCount++; // 增加读取计数
return processed; // 返回处理标志
}
drawFromMotion函数
-
计算位移变化:
OrientedPoint delta = absoluteDifference(pnew, pold);
假设
pnew
和pold
分别为:pnew = (x_new, y_new, theta_new) pold = (x_old, y_old, theta_old)
那么
delta
计算如下:delta.x = x_new - x_old delta.y = y_new - y_old delta.theta = theta_new - theta_old
-
添加高斯噪声:
-
x坐标:
noisypoint.x += sampleGaussian(srr * fabs(delta.x) + str * fabs(delta.theta) + sxy * fabs(delta.y));
假设
srr
,str
,sxy
分别为sigma_rr
,sigma_tr
,sigma_xy
,那么噪声计算如下:noise_x = sampleGaussian(sigma_rr * |delta.x| + sigma_tr * |delta.theta| + sigma_xy * |delta.y|) noisypoint.x = delta.x + noise_x
-
y坐标:
noisypoint.y += sampleGaussian(srr * fabs(delta.y) + str * fabs(delta.theta) + sxy * fabs(delta.x));
噪声计算如下:
noise_y = sampleGaussian(sigma_rr * |delta.y| + sigma_tr * |delta.theta| + sigma_xy * |delta.x|) noisypoint.y = delta.y + noise_y
-
角度:
noisypoint.theta += sampleGaussian(stt * fabs(delta.theta) + srt * sqrt(delta.x * delta.x + delta.y * delta.y));
假设
stt
,srt
分别为sigma_tt
,sigma_rt
,那么噪声计算如下:noise_theta = sampleGaussian(sigma_tt * |delta.theta| + sigma_rt * sqrt(delta.x^2 + delta.y^2)) noisypoint.theta = delta.theta + noise_theta
-
-
确保角度在正确范围内:
noisypoint.theta = fmod(noisypoint.theta, 2 * M_PI); if (noisypoint.theta > M_PI) noisypoint.theta -= 2 * M_PI;
这一步确保角度在 -π 到 π 之间。
-
计算最终位点:
return absoluteSum(p, noisypoint);
假设
p
为(x_p, y_p, theta_p)
,那么最终位点计算如下:final_point.x = x_p + noisypoint.x final_point.y = y_p + noisypoint.y final_point.theta = theta_p + noisypoint.theta
通过这些步骤,代码实现了在给定位移变化的基础上添加高斯噪声,并确保角度在合理范围内,最终得到一个带有噪声的位点。
OrientedPoint
MotionModel::drawFromMotion(const OrientedPoint& p, const OrientedPoint& pnew, const OrientedPoint& pold) const{
// 定义一个与位点变化无关的噪声参数
double sxy=0.3*srr;
// 计算新旧位点之间的变化量
OrientedPoint delta=absoluteDifference(pnew, pold);
// 创建一个基于变化量的噪声位点
OrientedPoint noisypoint(delta);
// 为x坐标添加高斯噪声
noisypoint.x+=sampleGaussian(srr*fabs(delta.x)+str*fabs(delta.theta)+sxy*fabs(delta.y));
// 为y坐标添加高斯噪声
noisypoint.y+=sampleGaussian(srr*fabs(delta.y)+str*fabs(delta.theta)+sxy*fabs(delta.x));
// 为角度添加高斯噪声
noisypoint.theta+=sampleGaussian(stt*fabs(delta.theta)+srt*sqrt(delta.x*delta.x+delta.y*delta.y));
// 确保角度在正确的范围内
noisypoint.theta=fmod(noisypoint.theta, 2*M_PI);
if (noisypoint.theta>M_PI)
noisypoint.theta-=2*M_PI;
// 将噪声位点与原始位点相加,得到最终结果
return absoluteSum(p,noisypoint);
}
scanMatch
1. 初始化得分总和
double sumScore = 0;
初始化一个变量 sumScore
用于存储所有粒子的扫描匹配得分总和。
2. 遍历所有粒子
for (ParticleVector::iterator it = m_particles.begin(); it != m_particles.end(); it++) {
遍历 m_particles
容器中的每一个粒子。
3. 存储校正后的位姿
OrientedPoint corrected;
声明一个 OrientedPoint
类型的变量 corrected
,用于存储优化后的粒子位姿。
4. 存储匹配得分、似然和分数
double score, l, s;
声明三个 double
类型的变量 score
、l
和 s
,分别用于存储匹配得分、似然和分数。
5. 优化校正后的位姿,并返回匹配得分
score = m_matcher.optimize(corrected, it->map, it->pose, plainReading);
调用 m_matcher.optimize
方法,优化当前粒子的位姿,并将优化后的位姿存储在 corrected
中,同时返回匹配得分 score
。
6. 如果匹配得分高于最小得分阈值,则更新粒子的位姿
if (score > m_minimumScore) {
it->pose = corrected;
} else {
// 匹配失败时,使用里程计数据,并记录相关信息
if (m_infoStream) {
m_infoStream << "Scan Matching Failed, using odometry. Likelihood=" << l << std::endl;
m_infoStream << "lp:" << m_lastPartPose.x << " " << m_lastPartPose.y << " " << m_lastPartPose.theta << std::endl;
m_infoStream << "op:" << m_odoPose.x << " " << m_odoPose.y << " " << m_odoPose.theta << std::endl;
}
}
如果匹配得分 score
高于预设的最小得分阈值 m_minimumScore
,则更新粒子的位姿为优化后的位姿 corrected
。否则,记录扫描匹配失败的信息,并使用里程计数据。
7. 计算粒子的似然和分数
m_matcher.likelihoodAndScore(s, l, it->map, it->pose, plainReading);
调用 m_matcher.likelihoodAndScore
方法,计算当前粒子的似然 l
和分数 s
。
8. 更新得分总和和粒子的权重
sumScore += score;
it->weight += l;
it->weightSum += l;
将当前粒子的匹配得分 score
累加到 sumScore
中,并更新粒子的权重 weight
和权重总和 weightSum
。
9. 为选择性复制活跃区域做准备,通过分离将被更新的区域
m_matcher.invalidateActiveArea();
m_matcher.computeActiveArea(it->map, it->pose, plainReading);
调用 m_matcher.invalidateActiveArea
和 m_matcher.computeActiveArea
方法,为选择性复制活跃区域做准备。
10. 记录平均扫描匹配得分
if (m_infoStream)
m_infoStream << "Average Scan Matching Score=" << sumScore / m_particles.size() << std::endl;
如果 m_infoStream
存在,记录并输出平均扫描匹配得分。
inline void GridSlamProcessor::scanMatch(const double* plainReading){
// 初始化得分总和
double sumScore=0;
// 遍历所有粒子
for (ParticleVector::iterator it=m_particles.begin(); it!=m_particles.end(); it++){
// 存储校正后的位姿
OrientedPoint corrected;
// 存储匹配得分、似然和分数
double score, l, s;
// 优化校正后的位姿,并返回匹配得分
score=m_matcher.optimize(corrected, it->map, it->pose, plainReading);
// 如果匹配得分高于最小得分阈值,则更新粒子的位姿
if (score>m_minimumScore){
it->pose=corrected;
} else {
// 匹配失败时,使用里程计数据,并记录相关信息
if (m_infoStream){
m_infoStream << "Scan Matching Failed, using odometry. Likelihood=" << l <<std::endl;
m_infoStream << "lp:" << m_lastPartPose.x << " " << m_lastPartPose.y << " "<< m_lastPartPose.theta <<std::endl;
m_infoStream << "op:" << m_odoPose.x << " " << m_odoPose.y << " "<< m_odoPose.theta <<std::endl;
}
}
// 计算粒子的似然和分数
m_matcher.likelihoodAndScore(s, l, it->map, it->pose, plainReading);
// 更新得分总和和粒子的权重
sumScore+=score;
it->weight+=l;
it->weightSum+=l;
// 为选择性复制活跃区域做准备,通过分离将被更新的区域
m_matcher.invalidateActiveArea();
m_matcher.computeActiveArea(it->map, it->pose, plainReading);
}
// 记录平均扫描匹配得分
if (m_infoStream)
m_infoStream << "Average Scan Matching Score=" << sumScore/m_particles.size() << std::endl;
}
resample函数
1. 初始化
bool hasResampled = false;
标记是否已经进行了重采样,初始值为false
。
2. 存储旧一代节点
TNodeVector oldGeneration;
for (unsigned int i=0; i<m_particles.size(); i++){
oldGeneration.push_back(m_particles[i].node);
}
遍历所有粒子,将每个粒子的节点存储到oldGeneration
中,以便后续使用。
3. 判断是否需要重采样
if (m_neff < m_resampleThreshold * m_particles.size()){
如果有效粒子数(m_neff
)小于重采样阈值(m_resampleThreshold * m_particles.size()
),则进行重采样。
4. 输出重采样信息
if (m_infoStream)
m_infoStream << "*************RESAMPLE***************" << std::endl;
如果信息流(m_infoStream
)存在,则输出重采样信息。
5. 创建并使用均匀重采样器
uniform_resampler<double, double> resampler;
m_indexes = resampler.resampleIndexes(m_weights, adaptSize);
创建均匀重采样器,并使用它对权重进行重采样,得到重采样索引m_indexes
。
6. 记录重采样信息
if (m_outputStream.is_open()){
m_outputStream << "RESAMPLE " << m_indexes.size() << " ";
for (std::vector<unsigned int>::const_iterator it = m_indexes.begin(); it != m_indexes.end(); it++){
m_outputStream << *it << " ";
}
m_outputStream << std::endl;
}
如果输出流(m_outputStream
)打开,则记录重采样信息。
7. 执行重采样更新
onResampleUpdate();
调用重采样更新函数。
8. 开始构建粒子树
ParticleVector temp;
unsigned int j = 0;
std::vector<unsigned int> deletedParticles;
初始化临时粒子向量temp
,索引j
和被删除粒子索引向量deletedParticles
。
9. 遍历重采样索引,构建新的粒子树
for (unsigned int i = 0; i < m_indexes.size(); i++){
while (j < m_indexes[i]){
deletedParticles.push_back(j);
j++;
}
if (j == m_indexes[i])
j++;
Particle & p = m_particles[m_indexes[i]];
TNode* node = 0;
TNode* oldNode = oldGeneration[m_indexes[i]];
node = new TNode(p.pose, 0, oldNode, 0);
node->reading = 0;
temp.push_back(p);
temp.back().node = node;
temp.back().previousIndex = m_indexes[i];
}
while (j < m_indexes.size()){
deletedParticles.push_back(j);
j++;
}
遍历重采样索引,构建新的粒子树,并记录被删除的粒子索引。
10. 删除不再需要的粒子节点
std::cerr << "Deleting Nodes:";
for (unsigned int i = 0; i < deletedParticles.size(); i++){
std::cerr << " " << deletedParticles[i];
delete m_particles[deletedParticles[i]].node;
m_particles[deletedParticles[i]].node = 0;
}
std::cerr << " Done" << std::endl;
删除不再需要的粒子节点。
11. 复制临时粒子,并重新注册扫描
std::cerr << "Deleting old particles...";
m_particles.clear();
std::cerr << "Done" << std::endl;
std::cerr << "Copying Particles and Registering scans...";
for (ParticleVector::iterator it = temp.begin(); it != temp.end(); it++){
it->setWeight(0);
m_matcher.invalidateActiveArea();
m_matcher.registerScan(it->map, it->pose, plainReading);
m_particles.push_back(*it);
}
std::cerr << " Done" << std::endl;
hasResampled = true;
清空旧粒子,复制临时粒子,并重新注册扫描,标记已重采样。
12. 如果不需要重采样,只需注册扫描
int index = 0;
std::cerr << "Registering Scans:";
TNodeVector::iterator node_it = oldGeneration.begin();
for (ParticleVector::iterator it = m_particles.begin(); it != m_particles.end(); it++){
TNode* node = 0;
node = new TNode(it->pose, 0.0, *node_it, 0);
node->reading = 0;
it->node = node;
m_matcher.invalidateActiveArea();
m_matcher.registerScan(it->map, it->pose, plainReading);
it->previousIndex = index;
index++;
node_it++;
}
std::cerr << "Done" << std::endl;
如果不需要重采样,只需注册扫描。
13. 返回是否进行了重采样
return hasResampled;
返回是否进行了重采样。
总结
该代码的主要功能是实现粒子滤波器中的重采样步骤。具体来说,它首先判断是否需要进行重采样,如果需要,则使用均匀重采样器对粒子进行重采样,构建新的粒子树,并删除不再需要的粒子节点。如果不需要重采样,则只需注册扫描。最终返回是否进行了重采样。
inline bool GridSlamProcessor::resample(const double* plainReading, int adaptSize, const RangeReading* ){
// 标记是否已经重采样
bool hasResampled = false;
// 存储旧一代节点
TNodeVector oldGeneration;
for (unsigned int i=0; i<m_particles.size(); i++){
oldGeneration.push_back(m_particles[i].node);
}
// 如果有效粒子数小于重采样阈值
if (m_neff<m_resampleThreshold*m_particles.size()){
// 输出重采样信息
if (m_infoStream)
m_infoStream << "*************RESAMPLE***************" << std::endl;
// 创建并使用均匀重采样器
uniform_resampler<double, double> resampler;
m_indexes=resampler.resampleIndexes(m_weights, adaptSize);
// 记录重采样信息
if (m_outputStream.is_open()){
m_outputStream << "RESAMPLE "<< m_indexes.size() << " ";
for (std::vector<unsigned int>::const_iterator it=m_indexes.begin(); it!=m_indexes.end(); it++){
m_outputStream << *it << " ";
}
m_outputStream << std::endl;
}
// 执行重采样更新
onResampleUpdate();
// 开始构建粒子树
ParticleVector temp;
unsigned int j=0;
std::vector<unsigned int> deletedParticles; // 存储被重采样移除的粒子索引,以便删除它们。
// 遍历重采样索引,构建新的粒子树
for (unsigned int i=0; i<m_indexes.size(); i++){
while(j<m_indexes[i]){
deletedParticles.push_back(j);
j++;
}
if (j==m_indexes[i])
j++;
Particle & p=m_particles[m_indexes[i]];
TNode* node=0;
TNode* oldNode=oldGeneration[m_indexes[i]];
node=new TNode(p.pose, 0, oldNode, 0);
node->reading=0;
temp.push_back(p);
temp.back().node=node;
temp.back().previousIndex=m_indexes[i];
}
while(j<m_indexes.size()){
deletedParticles.push_back(j);
j++;
}
// 删除不再需要的粒子节点
std::cerr << "Deleting Nodes:";
for (unsigned int i=0; i<deletedParticles.size(); i++){
std::cerr <<" " << deletedParticles[i];
delete m_particles[deletedParticles[i]].node;
m_particles[deletedParticles[i]].node=0;
}
std::cerr << " Done" <<std::endl;
// 结束构建粒子树
std::cerr << "Deleting old particles..." ;
m_particles.clear();
std::cerr << "Done" << std::endl;
std::cerr << "Copying Particles and Registering scans...";
// 复制临时粒子,并重新注册扫描
for (ParticleVector::iterator it=temp.begin(); it!=temp.end(); it++){
it->setWeight(0);
m_matcher.invalidateActiveArea();
m_matcher.registerScan(it->map, it->pose, plainReading);
m_particles.push_back(*it);
}
std::cerr << " Done" <<std::endl;
hasResampled = true;
} else {
// 如果不需要重采样,只需注册扫描
int index=0;
std::cerr << "Registering Scans:";
TNodeVector::iterator node_it=oldGeneration.begin();
for (ParticleVector::iterator it=m_particles.begin(); it!=m_particles.end(); it++){
// 在粒子树中创建新节点,并将其添加到旧树中
TNode* node=0;
node=new TNode(it->pose, 0.0, *node_it, 0);
node->reading=0;
it->node=node;
// 结束构建粒子树
m_matcher.invalidateActiveArea();
m_matcher.registerScan(it->map, it->pose, plainReading);
it->previousIndex=index;
index++;
node_it++;
}
std::cerr << "Done" <<std::endl;
}
// 结束构建粒子树
// 返回是否进行了重采样
return hasResampled;
}
下一篇:scanMatch函数核心部分