目录
1.深度卷积神经网络(a deep convolutional neural network)
输入图像的维度是,如果
,计算输出图像维度公式:
。s表示步幅,p表示填充的层数。filters的通道数是和输入图像的通道数
保持一致的。
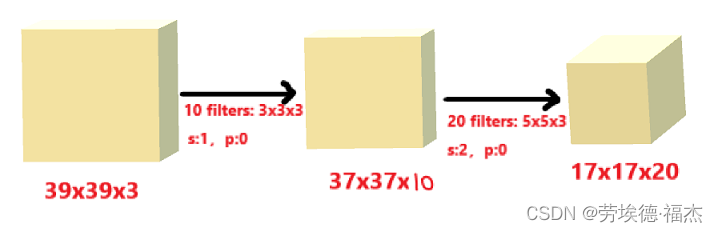
分析上图案例:
第一层卷积:(39+0-3)/1+1=37。即经过每一个filter卷积后,输出维度是37x37x1,由于有10个filters,所以最后输出为37x37x10。
第二层卷积:(37+0-5)/2+1=17。即经过每一个filter卷积后,输出维度是17x17x1,由于有20个filters,所以最后输出为17x17x20。
2.池化层(Pooling layer)
最大池化(Max pooling):用过滤器过滤出最大值。下图用的过滤器(filter)大小为2x2,步幅(stride)为2。
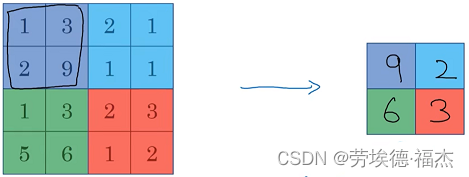
平均池化(Average pooling):用过滤器过滤出平均值。下图用的过滤器大小为2x2,步幅为2。
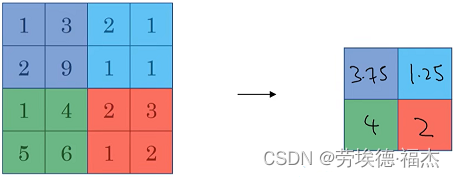
综上,池化层有两个超参数(hyperparameters):f(filter size过滤器大小)、s(stride,步幅)。这些参数不需要学习,都是手动设置或通过交叉验证设置的,都是静态属性。
3.