文章目录
- 数组图像:图像与像素
- 图像分类:机器如何学习?
- NMT:Neuron Machine Translation
- Transformer
- Vision Transformer
- 代码实战
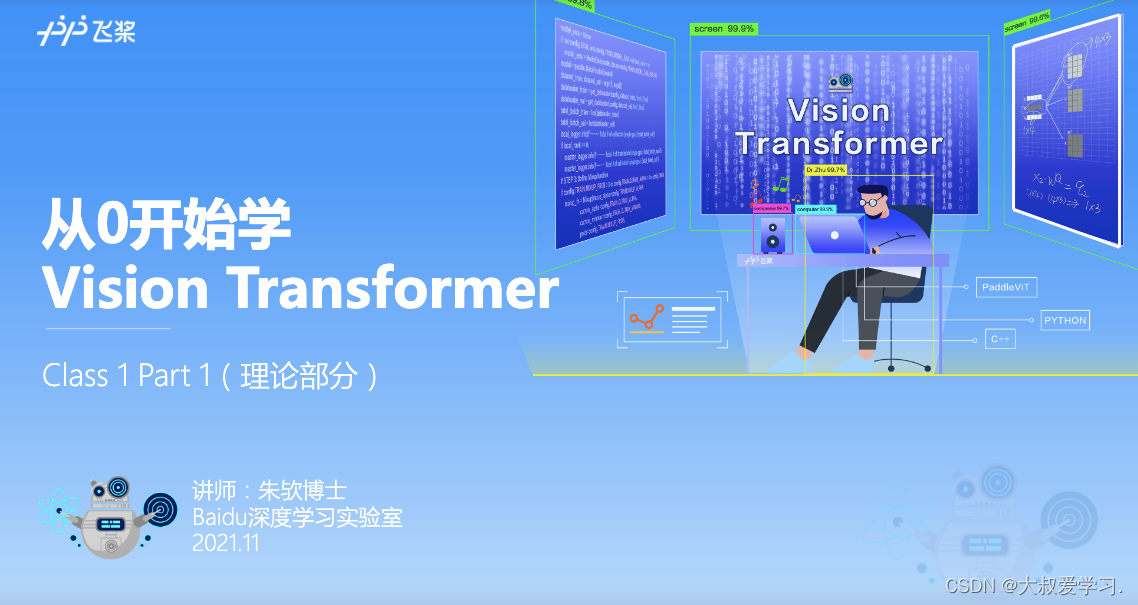
数组图像:图像与像素
什么是数字图像?在计算机图像的图像格式。每一个点叫pixel。type是uint8。
左边比右边清楚。
105可以用8位的二进制表示。24位的话,可以表示1600万个不同的颜色。HDR和杜比视界,用10位二进制表示一个颜色,所以是30位,1 billion个不同的颜色。
注意flatten是维度的展平,而Expand是一个维度的复制。
图像分类:机器如何学习?
NMT:Neuron Machine Translation
sequetial to sequential的。一个句子就是一个sequetial,一个序列,S2S,又是一个Encoder -> Decoder的过程。
Transformer
Transformer本质是一个Encoder+Decoder的过程。
Encoder和Decoder都不是一层,可能是多层。
FFN:Feed Forward Network
MSA:Multi head self-Attention
Vision Transformer
Vit 是没有Decoder的。Encoder就是实现每一层,然后resnet去跳连。
映射就是linear层,或者说可学习的层。
把图像分成块,就相当于NLP领域把一个句子分成分词。
Patch Embedding过程:一张[h, w, 3] img 按照window的大小,分成不同的patch,他们的维度变成[num_patch, Wh, Ww, 3]. 每一个patch我们讲他flatten成1维,然后过一个linear层,最后输出的就是一个token,所有token就是patch embedding。每一个token拉平就是embed_dim。
关于Linear Prejection是怎么操作的,可以看下图。
从卷积CNN的角度理解patch embedding。卷积里的参数值,其实就是Linear Project中的可学习参数w。embed_di的长度,其实就是卷积核的个数,比如我们有32个filter,那么embed_dim=32。
代码实战
import paddle
t = paddle.zeros([3, 3])
print(t)
t = paddle.randn([5, 3])
print(t)
from PIL import Image
import numpy as np
img = np.array(Image.open("img.png"))
print(img.shape)
# print(img)
t = paddle.to_tensor(img, dtype='float32')
print(type(t))
print(t.dtype)
t = t.transpose([2, 1, 0])
print(t.shape)
# chunk
t = paddle.randint(0, 10, [5, 15])
print(t)
qkv = t.chunk(3, -1)
print(qkv)
q, k, v = qkv
print(q)
# ViT Online Class
# Author: Dr. Zhu
# Project: PaddleViT (https://github.com/BR-IDL/PaddleViT)
# 2021.11
import paddle
import paddle.nn as nn
import numpy as np
from PIL import Image
# paddle.set_device('cpu')
class Identity(nn.Layer):
def __init__(self):
super().__init__()
def forward(self, x):
return x
class Mlp(nn.Layer):
def __init__(self, embed_dim, mlp_ratio=4.0, dropout=0.):
super().__init__()
self.fc1 = nn.Linear(embed_dim, int(embed_dim*mlp_ratio))
self.fc2 = nn.Linear(int(embed_dim*mlp_ratio), embed_dim)
self.act = nn.GELU()
self.dropout = nn.Dropout(dropout)
def forward(self, x):
x = self.fc1(x)
x = self.act(x)
x = self.dropout(x)
x = self.fc2(x)
x = self.dropout(x)
return x
class PatchEmbedding(nn.Layer):
def __init__(self, image_size, patch_size, in_channels, embed_dim, dropout=0.):
super().__init__()
self.patch_embedding = nn.Conv2D(in_channels,
embed_dim,
kernel_size=patch_size,
bias_attr=False)
self.dropout = nn.Dropout(dropout)
def forward(self, x):
# [n, c, h, w] [1, 1, 28, 28]
x = self.patch_embedding(x) # [n, c', h', w']
x = x.flatten(2) # [n, c', h'*w']
x = x.transpose([0, 2, 1]) # [n, h'*w', c'] h'*w'=num_patches
x = self.dropout(x)
return x
class Attention(nn.Layer):
def __init__(self):
super().__init__()
def forward(self, x):
return x
class EncoderLayer(nn.Layer):
def __init__(self, embed_dim):
super().__init__()
self.attn_norm = nn.LayerNorm(embed_dim)
self.attn = Attention()
self.mlp_norm = nn.LayerNorm(embed_dim)
self.mlp = Mlp(embed_dim)
def forward(self, x):
h = x
x = self.attn_norm(x)
x = self.attn(x)
x = x + h
h = x
x = self.mlp_norm(x)
x = self.mlp(x)
x = x + h
return x
class ViT(nn.Layer):
def __init__(self):
super().__init__()
self.patch_embed = PatchEmbedding(224, 7, 3, 16)
layer_list = [EncoderLayer(16) for i in range(5)]
self.encoders = nn.LayerList(layer_list)
self.head = nn.Linear(16, 10)
self.avgpool = nn.AdaptiveAvgPool1D(1)
self.norm = nn.LayerNorm(16)
def forward(self, x):
x = self.patch_embed(x) # [n, h*w, c]: 4, 1024, 16
for encoder in self.encoders:
x = encoder(x)
# avg
x = self.norm(x)
x = x.transpose([0, 2, 1])
x = self.avgpool(x)
x = x.flatten(1)
x = self.head(x)
return x
def main():
t = paddle.randn([4, 3, 224, 224])
model = ViT()
out = model(t)
print(out.shape)
if __name__ == "__main__":
main()