1、获取数据, 图像分割该数据有50行100列,每个数字占据20*20个像素点,可以进行切分,划分出训练集和测试集。
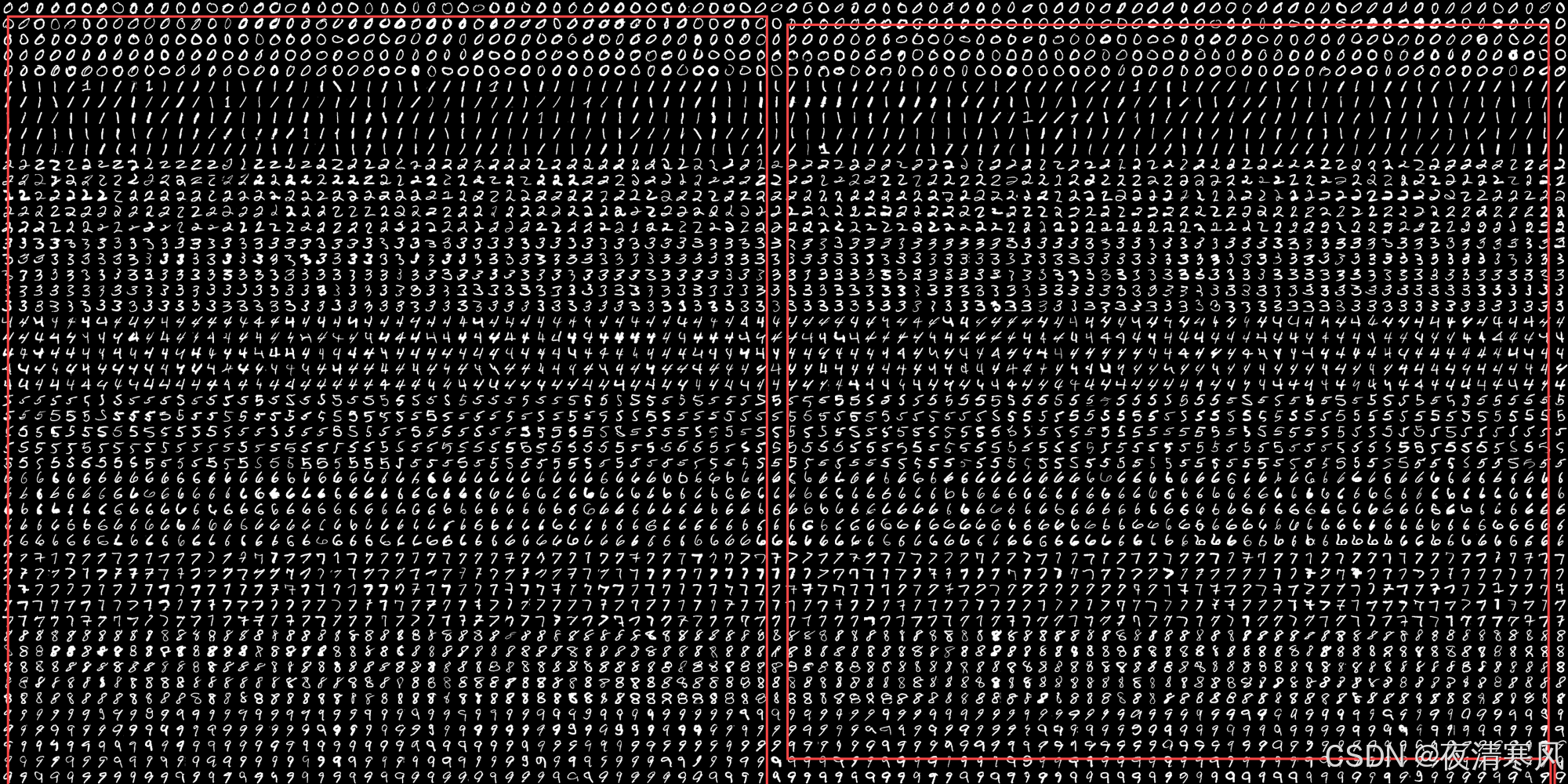
import numpy as np
import pandas as pd
import cv2
img=cv2.imread("digits.png")#读取文件
gray=cv2.cvtColor(img,cv2.COLOR_BGR2GRAY)#变成灰度图
#切分数据
x=np.array([np.hsplit(i,100) for i in np.vsplit(gray,50)])
train=x[:,:50]
test=x[:,50:100]
2、每个数据的像素点为20*20,将其全部变成一列1*400格式,转换成数值特征
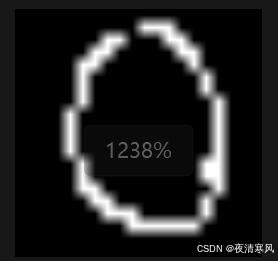
train_new=train.reshape(-1,400).astype(np.float32)
test_new=test.reshape(-1,400).astype(np.float32)
3、总共有2500行特征对应着2500个标签,从0到9每个数字有250个
k=np.arange(10)
train_labels=np.repeat(k,250)[:,np.newaxis].ravel()
test_labels=np.repeat(k,250)[:,np.newaxis].ravel()
4、导入逻辑回归库,采用交叉验证的方法找到最佳C值
#导入逻辑回归和交叉验证库
from sklearn.linear_model import LogisticRegression
from sklearn.model_selection import cross_val_score
scores=[]
# 设置C的值进行交叉验证,找到最佳C
c_param_range=[0.01,0.1,1,10,100]
for i in c_param_range:
lr = LogisticRegression(C=i, penalty='l2', solver='lbfgs', max_iter=10000)
score = cross_val_score(lr, train_new, train_labels, cv=10, scoring='recall_macro')
score_mean = sum(score) / len(score)
scores.append(score_mean)
# 选择使得平均分数最高的C值
best_c = c_param_range[np.argmax(scores)]
lr = LogisticRegression(C=best_c, penalty='l2', max_iter=10000)
#使用最佳C值初始化逻辑回归模型并训练
lr.fit(train_new, train_labels)
5、使用训练好的模型对测试集进行预测
from sklearn import metrics
train_predicted=lr.predict(test_new)
print(metrics.classification_report(test_labels,train_predicted))
6、打印的分类报告
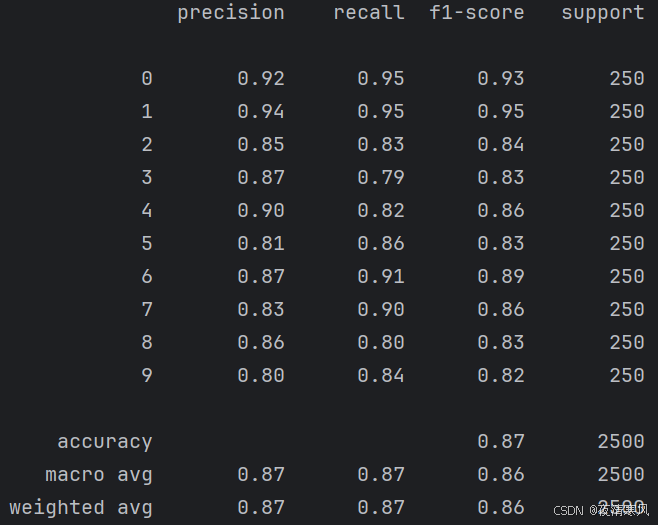
7、读取手写数字图像,并进行预测
p1=cv2.imread("p1.png")
gray_p1=cv2.cvtColor(p1,cv2.COLOR_BGR2GRAY)
tess=np.array(gray_p1)
tess_new=tess.reshape(-1,400).astype(np.float32)
# 使用训练好的模型进行预测
predicted_shouxie=lr.predict(tess_new)
print(predicted_shouxie)
8、书写预测结果

完整代码
import numpy as np
import pandas as pd
import cv2
img=cv2.imread("digits.png")#读取文件
gray=cv2.cvtColor(img,cv2.COLOR_BGR2GRAY)#变成灰度图
#切分数据
x=np.array([np.hsplit(i,100) for i in np.vsplit(gray,50)])
train=x[:,:50]
test=x[:,50:100]
train_new=train.reshape(-1,400).astype(np.float32)
test_new=test.reshape(-1,400).astype(np.float32)
k=np.arange(10)
train_labels=np.repeat(k,250)[:,np.newaxis].ravel()
test_labels=np.repeat(k,250)[:,np.newaxis].ravel()
#导入逻辑回归和交叉验证库
from sklearn.linear_model import LogisticRegression
from sklearn.model_selection import cross_val_score
scores=[]
# 设置C的值进行交叉验证,找到最佳C
c_param_range=[0.01,0.1,1,10,100]
for i in c_param_range:
lr = LogisticRegression(C=i, penalty='l2', solver='lbfgs', max_iter=10000)
score = cross_val_score(lr, train_new, train_labels, cv=10, scoring='recall_macro')
score_mean = sum(score) / len(score)
scores.append(score_mean)
# 选择使得平均分数最高的C值
best_c = c_param_range[np.argmax(scores)]
lr = LogisticRegression(C=best_c, penalty='l2', max_iter=10000)
lr.fit(train_new, train_labels)
#使用最佳C值初始化逻辑回归模型并训练
from sklearn import metrics
train_predicted=lr.predict(test_new)
print(metrics.classification_report(test_labels,train_predicted))
# 读取新的手写数字图像,并进行预测
p1=cv2.imread("p1.png")
gray_p1=cv2.cvtColor(p1,cv2.COLOR_BGR2GRAY)
tess=np.array(gray_p1)
tess_new=tess.reshape(-1,400).astype(np.float32)
# 使用训练好的模型进行预测
predicted_shouxie=lr.predict(tess_new)
print(predicted_shouxie)