写在前面
手撕Pytorch源码系列目的:
通过手撕源码复习+了解高级python语法
熟悉对pytorch框架的掌握
在每一类完成源码分析后,会与常规深度学习训练脚本进行对照
本系列预计先手撕python层源码,再进一步手撕c源码
版本信息
python:3.6.13
pytorch:1.10.2
本博文涉及python语法点
@staticmethod修饰器
super类的全新理解【大概率有你闻所未闻的华点!】
bisect二分法搜索方法
@property修饰器
目录
[TOC]
零、流程图
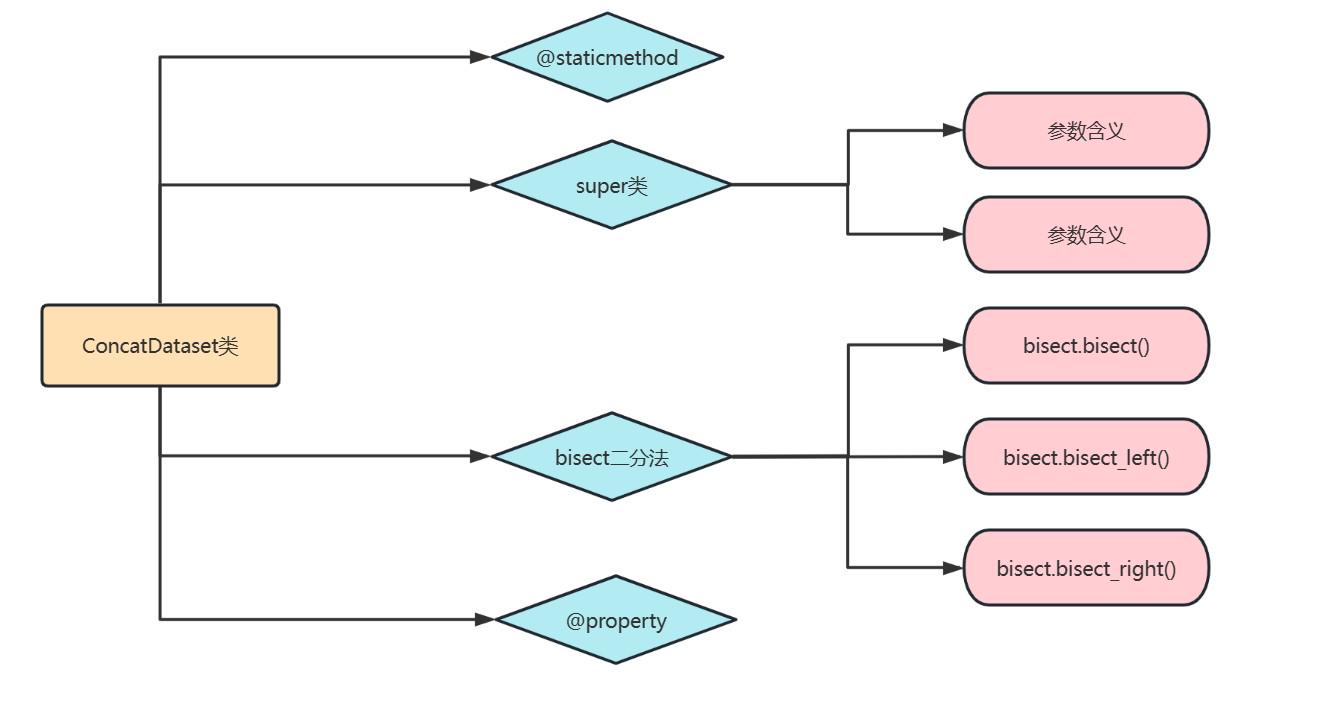
一、ConcatDataset类
在上篇博文中,Dataset类的__add__方法中使用了ConcatDataset类,因而本篇对其进行研究,并学习相关的python语法点
def __add__(self, other: 'Dataset[T_co]') -> 'ConcatDataset[T_co]':
return ConcatDataset([self, other])
Dataset[T_co],Type hint用法见上一篇博文
1.0 源代码
注:在第一部分未进行精讲的代码已在下方源代码处做了详细的注释!
class ConcatDataset(Dataset[T_co]):
datasets: List[Dataset[T_co]]
cumulative_sizes: List[int]
@staticmethod
def cumsum(sequence):
r, s = [], 0
for e in sequence:
l = len(e)
r.append(l + s)
s += l
return r
def __init__(self, datasets: Iterable[Dataset]) -> None:
super(ConcatDataset, self).__init__()
# 将Iterable[Dataset]转化成为List(Dataset)
self.datasets = list(datasets)
# 对传入的datasets序列的长度进行合法性判断
assert len(self.datasets) > 0, 'datasets should not be an empty iterable' # type: ignore[arg-type]
# 仅接收Dataset类型,不接收IterableDataset类型
for d in self.datasets:
assert not isinstance(d, IterableDataset), "ConcatDataset does not support IterableDataset"
self.cumulative_sizes = self.cumsum(self.datasets)
def __len__(self):
# cumulative_size的最后一个元素就是整个数据集序列的总长度
return self.cumulative_sizes[-1]
def __getitem__(self, idx):
# 考虑的是用负数进行索引的情况
if idx < 0:
if -idx > len(self):
raise ValueError("absolute value of index should not exceed dataset length")
# 对负数的索引值进行正向化,只需要用总长度+负数索引值即可
# 例如:对最后一个元素的负数索引为-1,总长度为n,则n-1恰好为正向索引值
# len(self)相当于调用__len__方法,获取总长度
idx = len(self) + idx
dataset_idx = bisect.bisect_right(self.cumulative_sizes, idx)
# 如果判定就是第一个数据集,那么对数据集的索引就是总的索引
if dataset_idx == 0:
sample_idx = idx
# 如果判定不是第一个数据集,那么对数据集的索引就不是总的索引
# 例如,计算出来的cumulative_size为[100,300,600,1000],而总索引为569
# 那么可以判定出来索引位于第三个数据集上(数据集大小为300)
# 那么在第三个数据集上的索引应该是569-300=269
else:
sample_idx = idx - self.cumulative_sizes[dataset_idx - 1]
return self.datasets[dataset_idx][sample_idx]
@property
def cummulative_sizes(self):
warnings.warn("cummulative_sizes attribute is renamed to "
"cumulative_sizes", DeprecationWarning, stacklevel=2)
return self.cumulative_sizes
1.1 @staticmethod以及cumsum()函数
@符号表示修饰器,而staticmethod则声明了该函数为类的静态方法,具体解释见【2.1节@staticmethod】
cumsum(sequence)用于计算每个数据集累计长度的序列,为了方便__getitem__方法中,通过索引的序列号index定位序列号位于哪个数据集
假定传入的数据集序列为datasets = [ds1,ds2,ds3,ds4]共有四个数据集,其长度分别为[100,200,300,400],则cumcum(sequence)函数则会生成列表cumulative = [100,300,600,1000]。如果__getitem__方法传入的序列号为596,则可以用cumulative列表判断该序号的数据属于第三个数据集,进而可以从ds3中取得相应的数据
1.2 super(ConcatDataset, self).__init__()
上述语句的作用为调用ConcatDataset类的父类Dataset的初始化函数
super类的精讲见【2.2节super类】,关于其中涉及的mro链的精讲见下一篇博文
1.3 dataset_idx = bisect.bisect_right(self.cumulative_sizes, idx)
bisect_right函数的精讲见【2.3节bisect二分法搜索】
源代码利用bisect_right通过二分法找到目标索引值index在前文所述cumsum(sequence)函数生成的列表中的位置,并且通过该位置即可判定该索引位于数据集序列的第几个数据集中,从而计算出dataset_idx
1.4 @properpy以及cummulative_sizes(self)
@properpy用于是函数可以像属性一样被直接调用,精讲见【2.4节@property】
cummulative_sizes(self)函数其实为了版本兼容,通过warning.warn提示用户此方法已经改名,并将正确的值通过函数传递给该方法
warnings.warn(message, category=None, stacklevel=1, source=None)函数用于抛出警告,而上述代码中的DeprecationWarning是代码被弃用的警告
二、相应的Python语法补充
2.1 @staticmethod
@符号表示修饰器,而staticmethod则声明了该函数为类的静态方法,静态方法直接属于类,不需要传入self参数,正如外部函数一样进行定义,并且可以直接通过类或对象进行调用
class Static():
def __init__(self,x:int)->None:
self.x = x
@staticmethod
def static()->None:
print("Trying static")
Static.static()
s = Static(1)
s.static()
# 输出
# Trying static
# Trying static
2.2 super类
冷知识:super()其实并不是一个函数,更不是一个关键字,而是一个类,因此在程序中使用super()是创建了一个对象,而super的原型则是super(type,type or object)
super类传入的两个参数分别代表什么:
第一个type为一个类名,如上代码中的ConcatDataset,而第二个object则往往是一个M object,决定一个mro chain【mro链精讲见下一篇博文】
而第一个type决定了在mro chain中的取值位置,即从第一个type类下一个类开始,在第二个object决定的mro链上寻找最近的函数进行调用
当然,光说很抽象,直接上代码
from objprint import op
class Animal():
def __init__(self,age):
self.age = age
class Person(Animal):
def __init__(self, age,name):
super(Person,self).__init__(age)
self.name = name
class Male(Person):
def __init__(self,age,name):
super(Person,self).__init__(age)
self.gender = 'male'
class Female(Person):
def __init__(self,age,name):
super(Female,self).__init__(age,name)
self.gender = 'female'
m = Male(50,"Tim")
fm = Female(40,"Lily")
op(m)
op(fm)
# 输出结果:
# <Male 0x166c47a5d90
# .age = 50,
# .gender = 'male'
# >
# <Female 0x166c47a5c10
# .age = 40,
# .gender = 'female',
# .name = 'Lily'
# >
根据上述代码结果可以发现:Male类与Female中super()的第二个参数均为各自类别的self,因此Male类与Female类对应的mro链为Male:Male->Person->Animal,Female:Female->Person->Animal
Male:而在Male类中super()传入的第一个参数为Person因此从mro链中Person的下一个类,即Animal类开始查找__init__初始化函数,因此最终Male类的初始化函数相当于仅调用了Animal.__init__函数,因而无法对name属性进行初始化定义
Female:而Female类中super()传入的第一个参数为Female因此从mro链中Female的下一个类,即Person类开始查找__init__初始化函数,因此首先调用Person.__init__对name属性进行初始化
Female:接着,对于Person类,其super()的第二个参数为Person类比的self,因此其mro链为Person:Person->Animal,故从Animal类开始寻找__init__函数进行初始化。最终Female类的初始化函数相当于同时调用了Person.__init__函数以及Animal.__init__函数,因而对name和age属性都进行了初始化定义
super类可能存在的黑魔法
首先上代码看例子
class A():
def display(self):
print("A")
class B(A):
def display(self):
super(B,self).display()
class C(A):
def display(self):
print("C")
class D(B,C):
def display(self):
super(B,self).display()
b = B()
d = D()
b.display()
d.display()
# 输出结果为:
# A
# C
或者对上述代码中的D类换一种写法
class D(B,C):
def display(self):
B.display(self)
d = D()
d.display()
# 输出结果为:
# C
那么为什么调用B.display函数最后会输出C,而C则和B没有任何的继承与被继承关系呢?
首先,由于D继承了B和C两个类,因此其mro链为D:D->B->C->A,而super()类输入的第一个参数为B,即从B的下一个类开始寻找display()函数,进而调用C的display()函数最终输出了C
因此,虽然B与C类没有任何的继承与被继承关系,但两类通过D类的mro链被联系在一起
2.3 bisect二分法搜索
python的bisect库有三种二分法搜索的API分别是:bisect.bisect(Sequence,x),bisect.bisect_left(Sequence,x),bisect.bisect_right(Sequence,x)
其中,在二分法搜索上bisect.bisect(Sequence,x)与bisect.bisect_right(Sequence,x)是完全等效的
bisect.bisect(Sequence,x)与bisect.bisect_right(Sequence,x)是找到>传入值x的最小下标值
而bisect.bisect_left(Sequence,x)则是找到≥传入值x的最小下标值
话不多说,直接上代码:
import bisect
l = [100,255,512,1036]
print(bisect.bisect(l,500))
print(bisect.bisect_right(l,500))
print(bisect.bisect_left(l,500))
print(bisect.bisect(l,512))
print(bisect.bisect_right(l,512))
print(bisect.bisect_left(l,512))
# 输出值为:
# 2
# 2
# 2
# 3
# 3
# 2
2.4 @property
@property目的是可以让函数像属性一样被调用
而将属性变化成函数则是可以避免类调用时,对象的属性被非法修改
如一个长方形类,其属性为长,宽与面积,而如果将面积作为属性进行定义,那么在类外,可以直接利用self.area对面积值进行修改,从而使得长宽与面积值的不匹配,而用@property修饰器则可以解决该问题
代码示例如下:
class Property():
def __init__(self,height,width) -> None:
self.height = height
self.width = width
@property
def area(self):
return self.height*self.width
p = Property(2,4)
print(p.area)
# 输出结果为:
# 8