Day 21 Self- Attention
选修部分
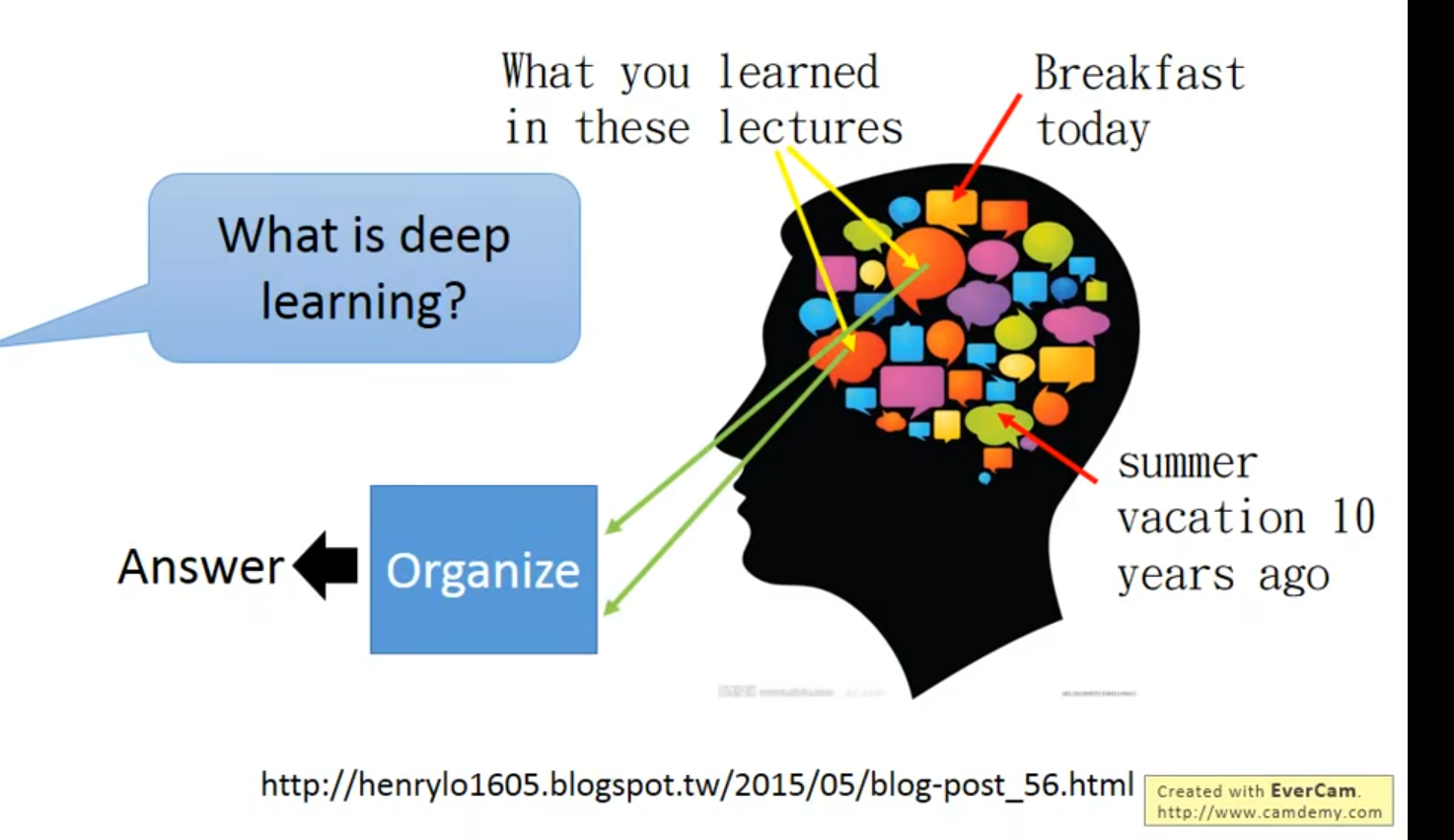
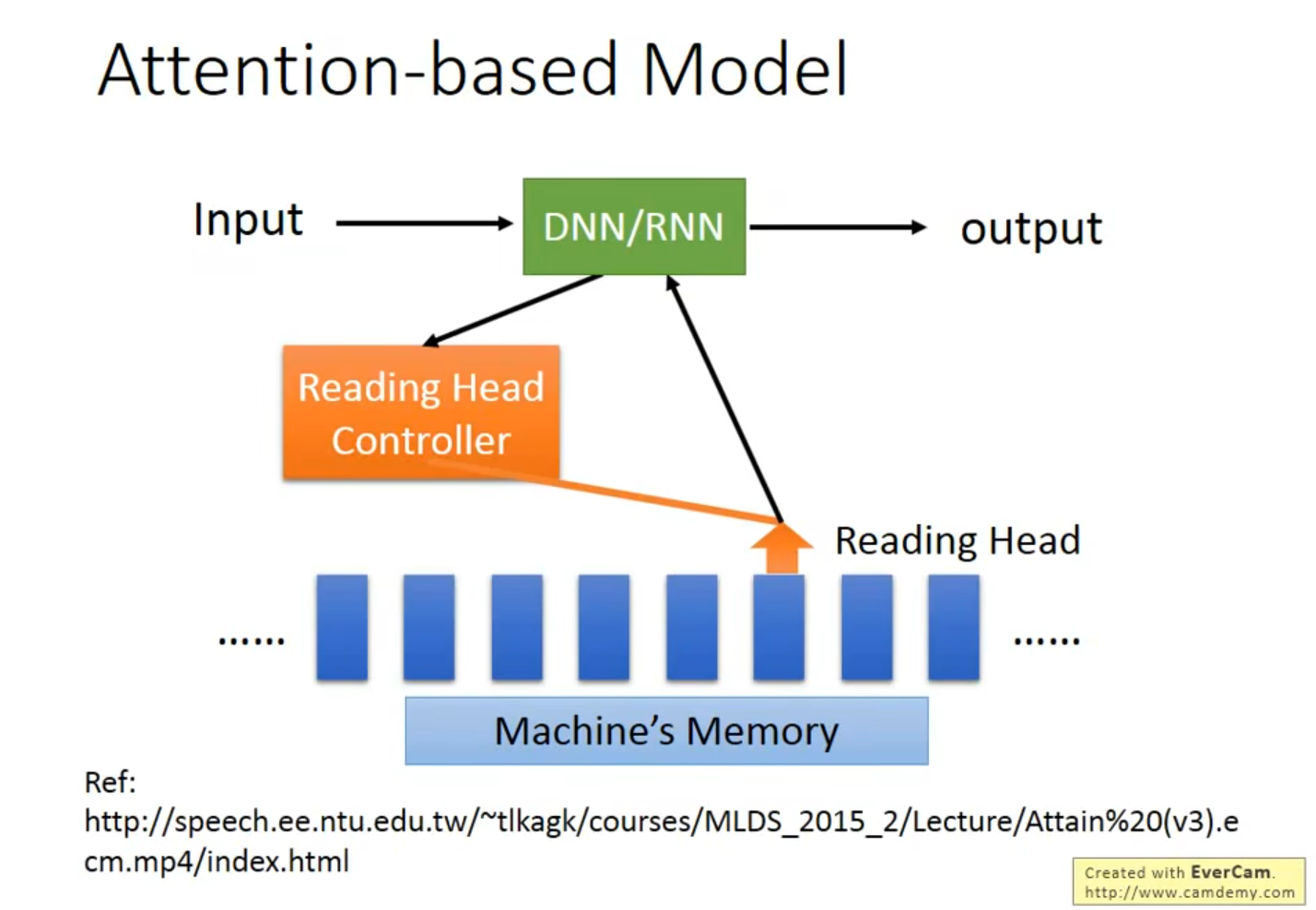
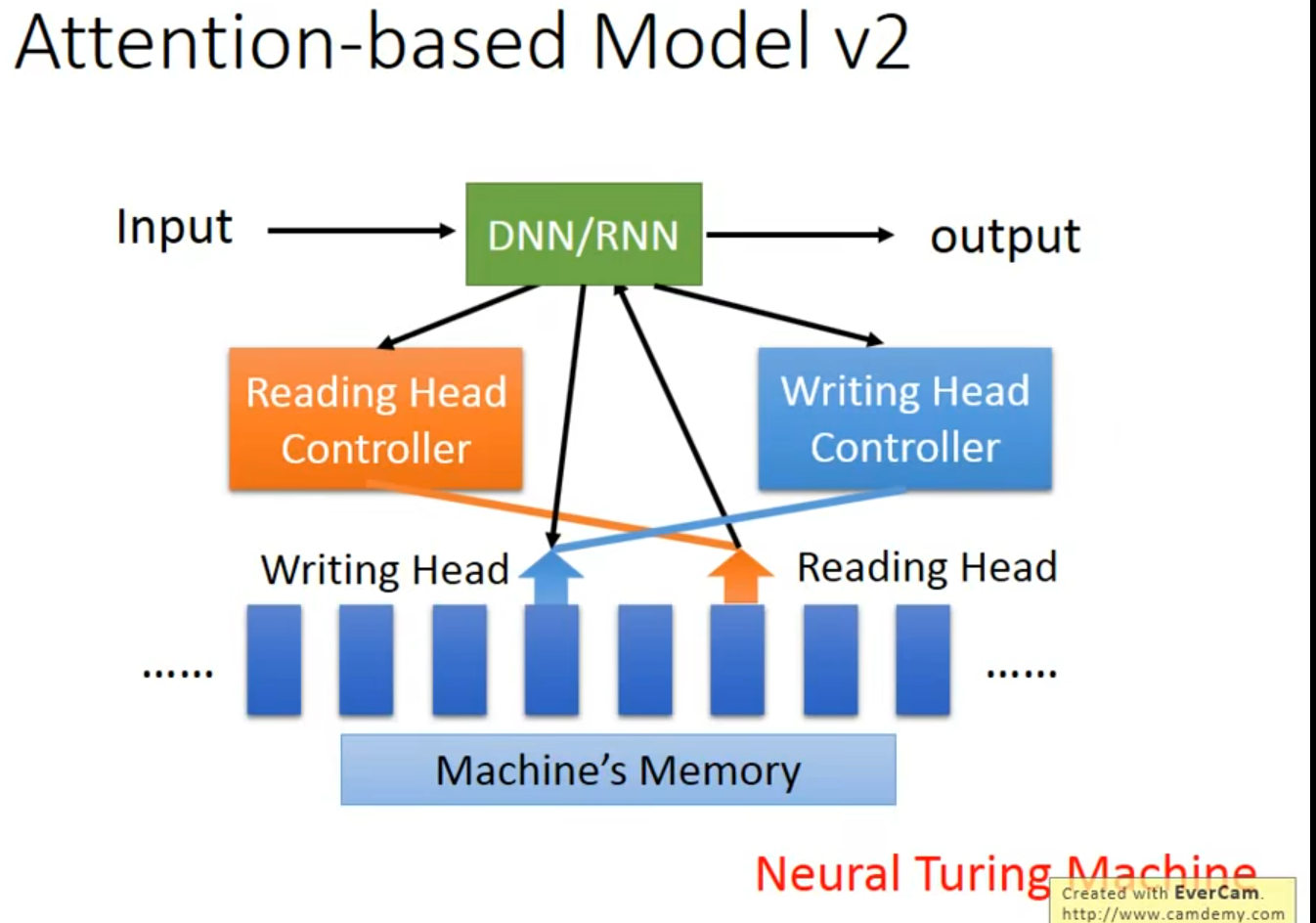
学完自适应 再回来看看
Sequence Labling
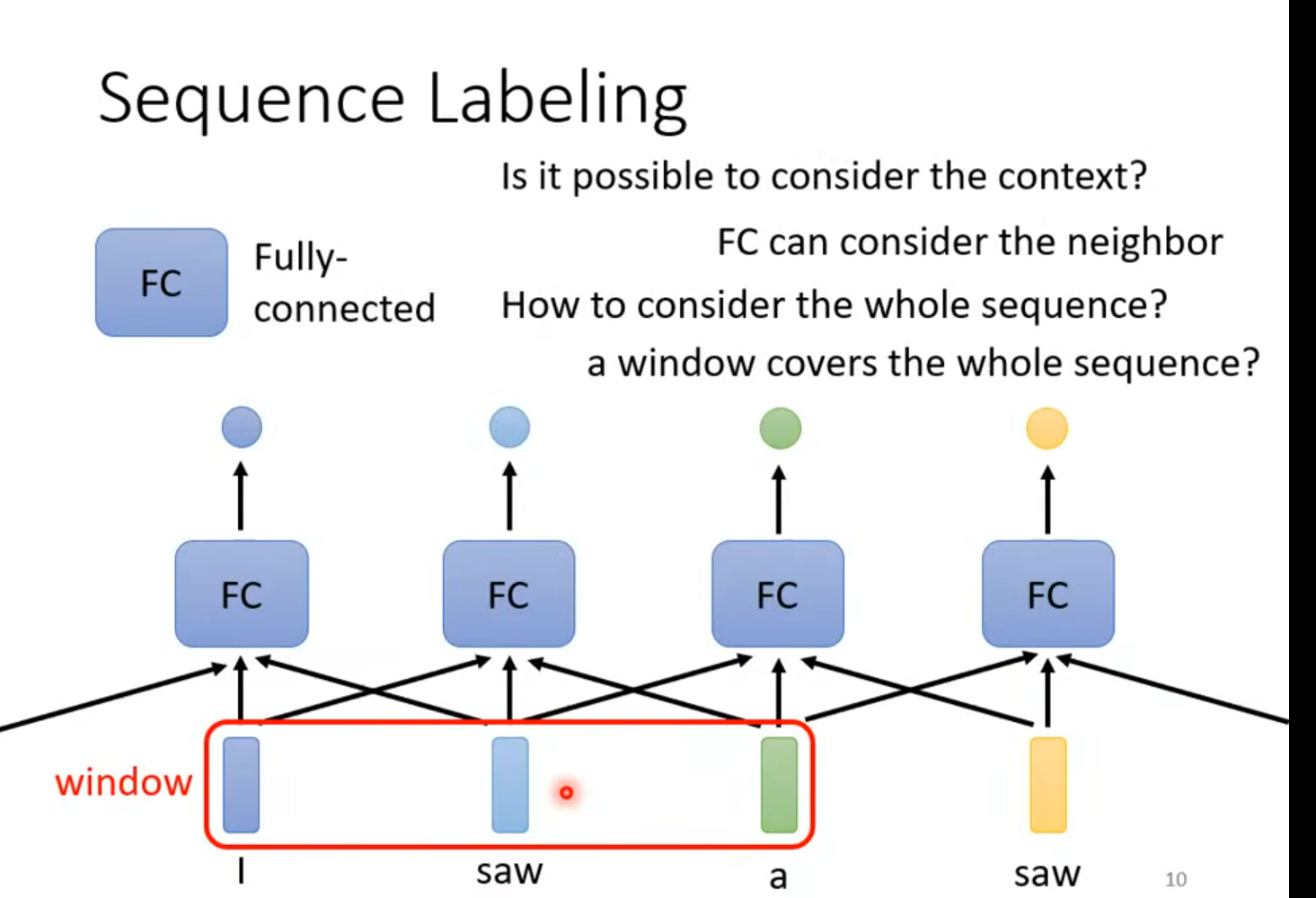
假如我们现在有一个需要读完全部句子才能解的问题, 那么red window 就需要变得是最大的(最长的句子);
其实这里大家有没有想过,这个玩意儿就是个卷积网络CNN,所谓的window 就是卷积核
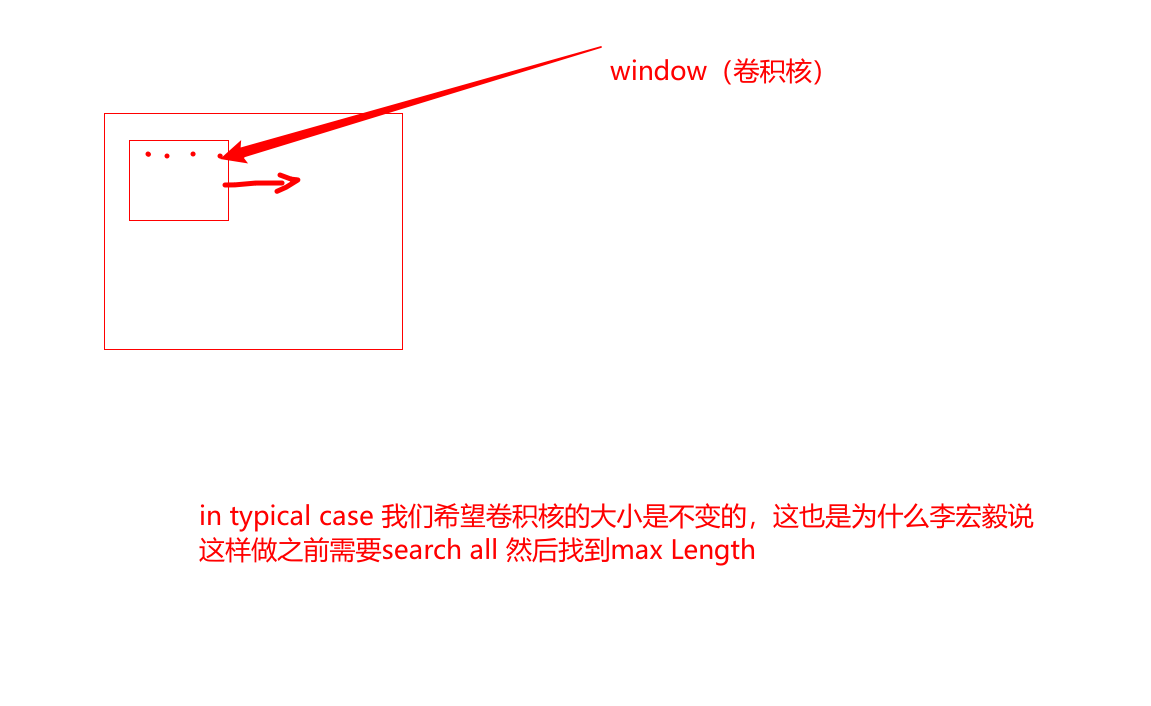
what is self Attention?
how self-attention work
主要考虑 Dot -product
实际操作自己也要做关联计算qk
如果b1 和 v2 比较接近的话,那么我们就说这a1 和a2 比较像
b1 --b4 是同时产生的
矩阵运算的角度
你也可以不做softmax(Relu 也行)
(小bug是 a_head 换成 ')
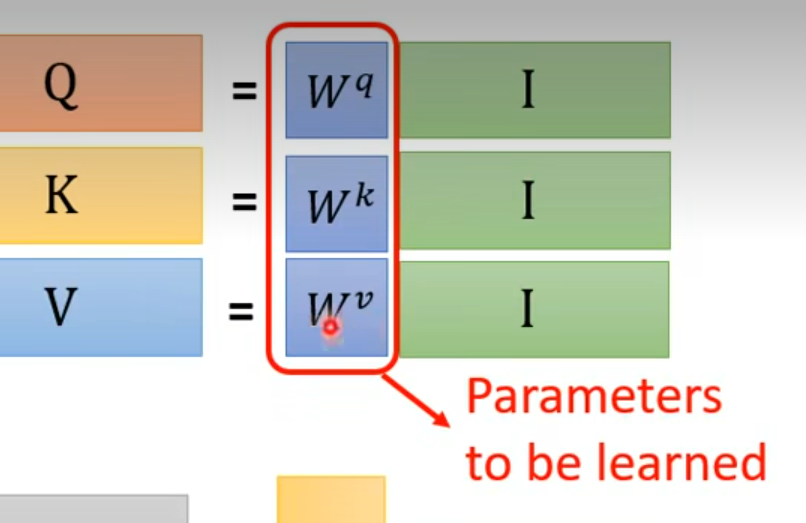
Multi-head -self-attention
Positional Encoding
hand - crafted (s to s 的规则使得不会超过位置信息)
can learned from data
这里感觉不到数学的巧妙,只是感到了工程的流水线的简洁和高效
Applicantions
Self -attention vs CNN
弹性较大,数据较小的时候容易过拟合
提问:
- 我们知道 fc 和cnn差不多(无非是fc更宽一些,如果你把cnn当初fc做的话有可能丢失位置信息,或可能需要postion encode),那么问你为什么不把windows变得很大去卷积呢?
- 如果说像老师说的
- 无法得知最长的sequerence
- 参数量大(这里不太明白参数量大在什么地方)
Self-Attention vs RNN
- 这里和我理解的差不多,就是特征彼此离得太远有点记不住了
- RNN 无法进行并行计算
Self - Attention for Graph
可以做智能知识图谱哎,相关性度量;this is one type of Graph Neural Network(GNN)