Link of original Github repo
Link of personal made study case of HR-VITON
Content
- Pre
- 1、OpenPose(On colab, need GPU)
- 2、Human Parse
- Method 1: Colab
- Method 2: Local or Server
- 3、DensePose (On colab, GPU or CPU)
- 4、Cloth Mask (On colab, GPU or CPU)
- 5、Parse Agnostic (On colab)
- 6、Human Agnostic
- 7、Conclusion
Pre
According to explanation from authors: Preprocessing.md. At least a few steps are needed for getting all required inputs of model.
- OpenPose
- Human Parse
- DensePose
- Cloth Mask
- Parse Agnostic
- Human Agnostic
Most of those are reproduced on Colab, except Human Parse, which needs Tensorflow 1.15 and GPU is highly prefered.
1、OpenPose(On colab, need GPU)
(1) Install OpenPose, taking about 15 minutes
import os
from os.path import exists, join, basename, splitext
git_repo_url = 'https://github.com/CMU-Perceptual-Computing-Lab/openpose.git'
project_name = splitext(basename(git_repo_url))[0]
if not exists(project_name):
# see: https://github.com/CMU-Perceptual-Computing-Lab/openpose/issues/949
# install new CMake becaue of CUDA10
!wget -q https://cmake.org/files/v3.13/cmake-3.13.0-Linux-x86_64.tar.gz
!tar xfz cmake-3.13.0-Linux-x86_64.tar.gz --strip-components=1 -C /usr/local
# clone openpose
!git clone -q --depth 1 $git_repo_url
!sed -i 's/execute_process(COMMAND git checkout master WORKING_DIRECTORY ${CMAKE_SOURCE_DIR}\/3rdparty\/caffe)/execute_process(COMMAND git checkout f019d0dfe86f49d1140961f8c7dec22130c83154 WORKING_DIRECTORY ${CMAKE_SOURCE_DIR}\/3rdparty\/caffe)/g' openpose/CMakeLists.txt
# install system dependencies
!apt-get -qq install -y libatlas-base-dev libprotobuf-dev libleveldb-dev libsnappy-dev libhdf5-serial-dev protobuf-compiler libgflags-dev libgoogle-glog-dev liblmdb-dev opencl-headers ocl-icd-opencl-dev libviennacl-dev
# install python dependencies
!pip install -q youtube-dl
# build openpose
!cd openpose && rm -rf build || true && mkdir build && cd build && cmake .. && make -j`nproc`
Now, OpenPose will be installed under your current path.
(2) Get all needed models
!. ./openpose/models/getModels.sh
(3) Prepare your test data
# for storing input image
!mkdir ./image_path
# copy official provided data to image_path, you may need to download and unzip it in advance
!cp ./test/image/000* ./image_path/
# create directories for generated results of OpenPose
!mkdir ./json_path
!mkdir ./img_path
(4)Run
# go to openpose directory
%cd openpose
# run openpose.bin
!./build/examples/openpose/openpose.bin --image_dir ../image_path --hand --disable_blending --display 0 --write_json ../json_path --write_images ../img_path --num_gpu 1 --num_gpu_start 0
Then json files will be saved under …/json_path and images will be saved under …/img_path.
The image result looks like
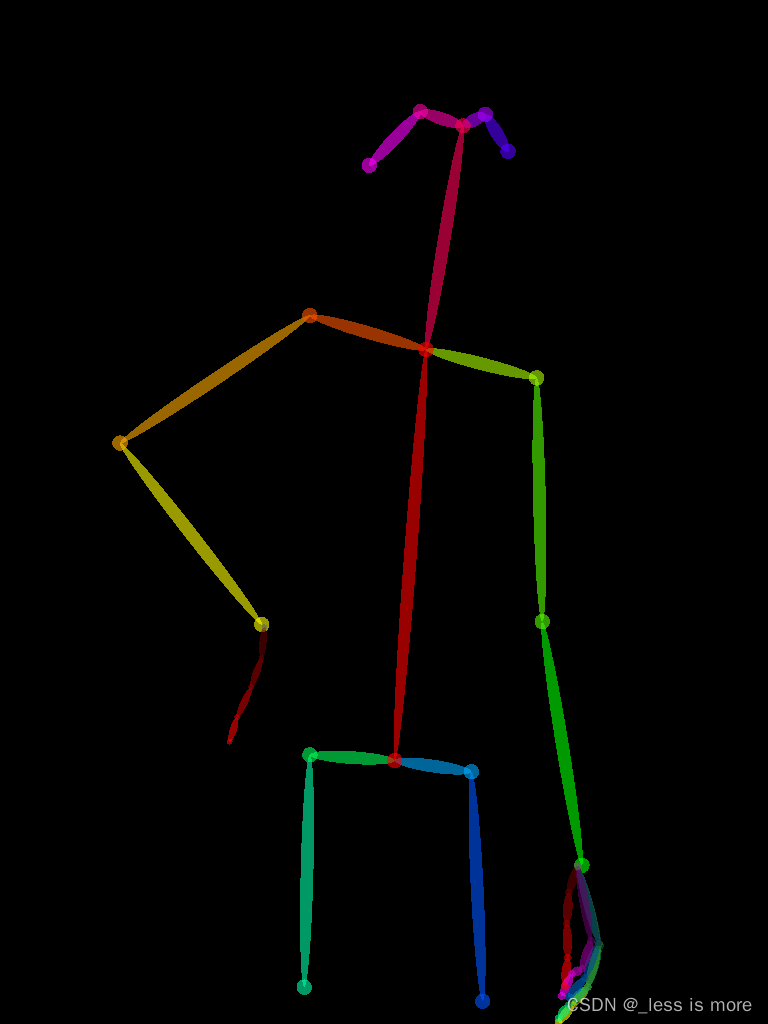
More details about results can be found at openpose
2、Human Parse
In this section, you can either do it on Colab, Cloud, or local. Unfortunately, I didn’t successfully make use of GPU on Colab, and I can only use CPU, which is super slow when image size at 768 × 1024 (about 13 minutes per image).
Method 1: Colab
If you can accept, then install Tensorflow 1.15, before which you have to change Python version to 3.7 or 3.6.
(1) Get pretrained model
%%bash
FILE_NAME='./CIHP_pgn.zip'
FILE_ID='1Mqpse5Gen4V4403wFEpv3w3JAsWw2uhk'
curl -sc /tmp/cookie "https://drive.google.com/uc?export=download&id=$FILE_ID" > /dev/null
CODE="$(awk '/_warning_/ {print $NF}' /tmp/cookie)"
curl -Lb /tmp/cookie "https://drive.google.com/uc?export=download&confirm=${CODE}&id=$FILE_ID" -o $FILE_NAME
unzip
!unzip CIHP_pgn.zip
(2) Get repo
!cp -r /content/drive/MyDrive/CIHP_PGN ./
%cd CIHP_PGN
Note: I just saved the repo and cleaned it for my own purpose, but you can use official provided code as well.
(3) Prepare data and model
!mkdir -p ./checkpoint
!mkdir -p ./datasets/images
# You also need to download dataset provided or use your own images
!mv ../CIHP_pgn ./checkpoint/CIHP_pgn
!cp ../test/image/0000* ./datasets/images
(4) Configuration
Change to Python 3.6
!sudo update-alternatives --config python3
Install dependencies (Tensorflow 1.15)
!sudo apt-get install python3-pip
!python -m pip install --upgrade pip
!pip install matplotlib opencv-python==4.2.0.32 Pillow scipy tensorflow==1.15
!pip install ipykernel
(5) Run
now you can run your code
!python ./inference_pgn.py
Note: In official repo, the file is named inf_pgn.py, which leads to the same result as mine.
Finally, you can get result looks like
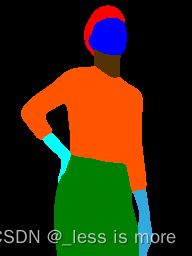
More details can be found at CIHP_PGN
Method 2: Local or Server
In this section, I will give more explanation about what we really need.
You need conda in this part, which is what I used at least.
(1) Create a new env for oldschool Tensorflow
conda create -n tf python=3.7
(2) Configuration
conda activate tf
install GPU dependencies: cudatoolkit=10.0 cudnn=7.6.5
conda install -c conda-forge cudatoolkit=10.0 cudnn=7.6.5
install Tensorflow 1.15 GPU
pip install tensorflow-gpu==1.15
You may need to install below in a new env
pip install scipy==1.7.3 opencv-python==4.5.5.62 protobuf==3.19.1 Pillow==9.0.1 matplotlib==3.5.1
More info about compatibility between Tensorflow and CUDA can be found here
(3) Prepare data, repo and model as mentioned before
A final dir looks like
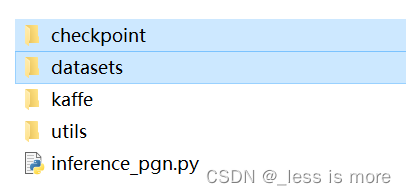
So you basically just put model under checkpoint/CIHP_pgn
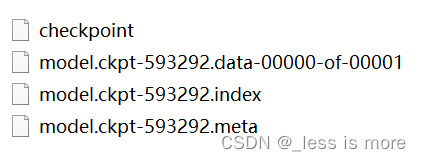
And put data under datasets/images
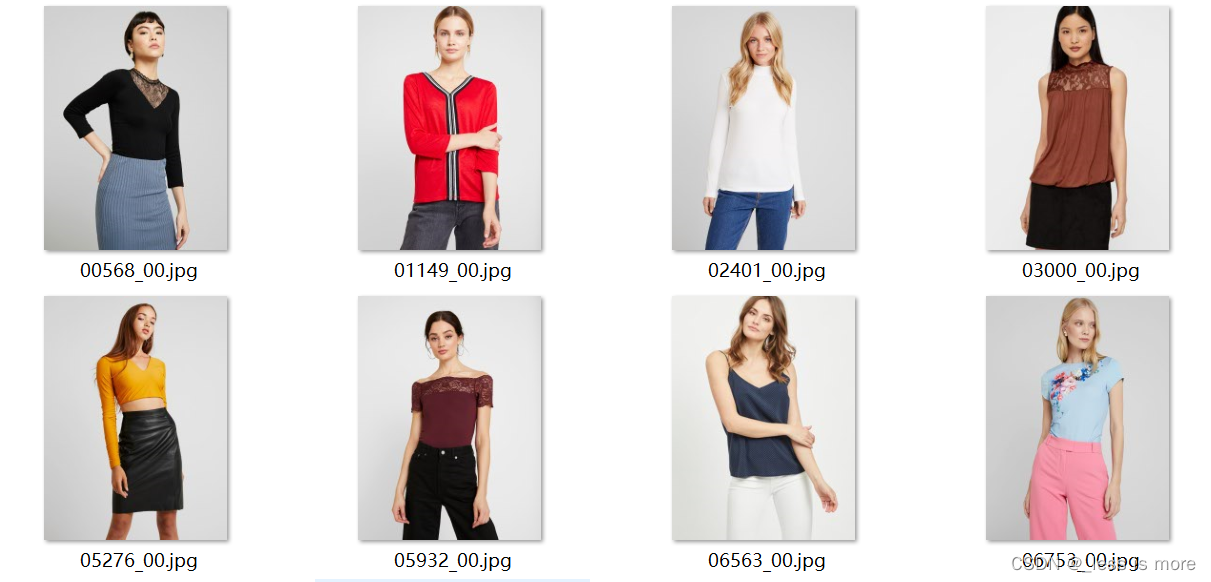
It can be just a few images of people. A repo of my cleaned version can be found at Google Drive. Feel free to download it. If you use official provided inf_pgn.py, same results will be generated.
(4) Run
python inference_pgn.py
Then you should see the output. Unfortunately, I didn’t make it inference with GPU, no matter on server or local.
At local, my GPU is MX250 with 2G memory, which is not enough for inference.
At server, the GPU is RTX A5000, but for some unknown reason, probably something incompatible, the GPU is not invoked for inference. But model is successfully loaded into GPU though.
Fortunately, the server I used has 24 Cores and supports 2 threads per Core, which make it running still fast (20 to 30 seconds per 768×1024 image) even with CPU.
Final result looks like
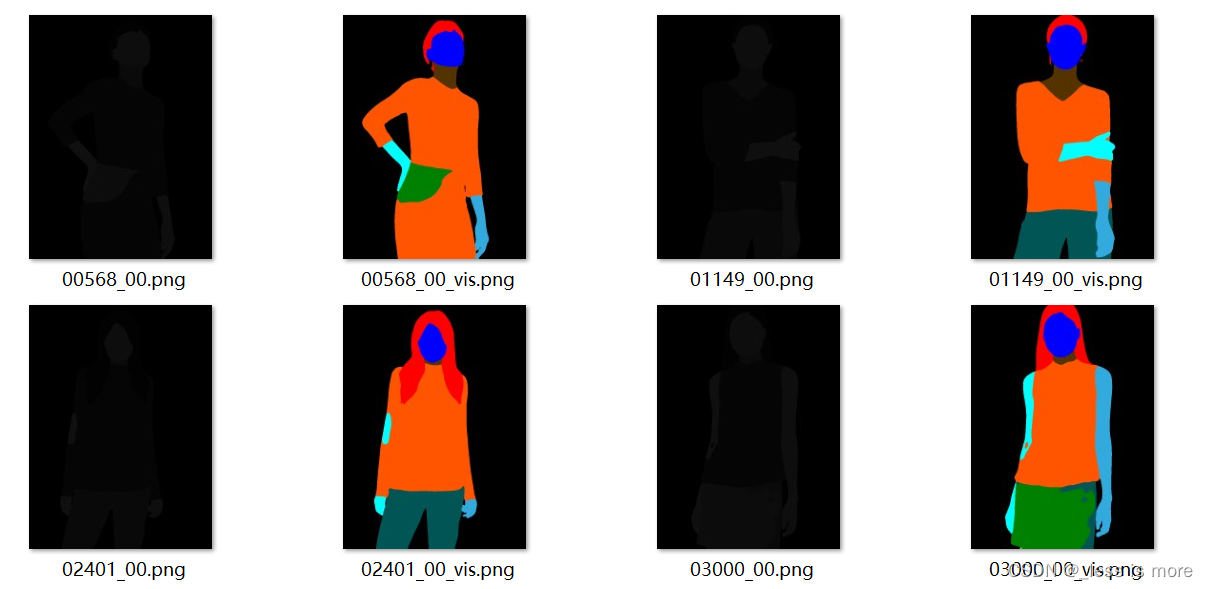
However, the result inferenced with input of 768×1024 is not the same as input of 192×256. The former looks worse as shown above.
Note: The black images are what we really need, because the values of colored one are for example 0, 51, 85, 128, 170, 221, 255, which are not from 0 - 20 and inconsistant with HR-VITON. The values of black one are for example 0, 2, 5, 10, 12, 13, 14, 15, which are needed as labels for getting agnostic images.
One thing to mention, the images provided by official dataset keep both visualization (colored) and label (0 - 20). I don’t know how they did that. I also tried P mode in PIL, but found nothing.
3、DensePose (On colab, GPU or CPU)
(1) get repo of detectron2
!git clone https://github.com/facebookresearch/detectron2
(2) install dependencies
!python -m pip install -e detectron2
(3) install packages for DensePose
%cd detectron2/projects/DensePose
!pip install av>=8.0.3 opencv-python-headless>=4.5.3.56 scipy>=1.5.4
(4) Prepare your images
!mkdir ./image_path
!cp /content/test/image/0000* ./image_path/
(5) Modify code
At the time I used DensePose, there are some bugs, I have to modify some code to make it work as I want it to. When you follow this tutorial, situation may change.
- For getting same input as HR-VITON, change ./densepose/vis/densepose_results.py in line 320
alpha=0.7 to 1
inplace=True to False
- change ./densepose/vis/base.py, line 38
This modification is because above change is not enough, image_target_bgr = image_bgr * 0 made a copy instead of a reference and lost our result.
image_target_bgr = image_bgr * 0
to
image_target_bgr = image_bgr
image_target_bgr *= 0
- To save file with name kept and in directory, change apply_net.py, line 286 and 287 to below
out_fname = './image-densepose/' + image_fpath.split('/')[-1]
out_dir = './image-densepose'
(6) Run
If you are using CPU, add --opts MODEL.DEVICE cpu
to end of below command.
!python apply_net.py show configs/densepose_rcnn_R_50_FPN_s1x.yaml \
https://dl.fbaipublicfiles.com/densepose/densepose_rcnn_R_50_FPN_s1x/165712039/model_final_162be9.pkl \
image_path dp_segm -v
Then you can get results look like
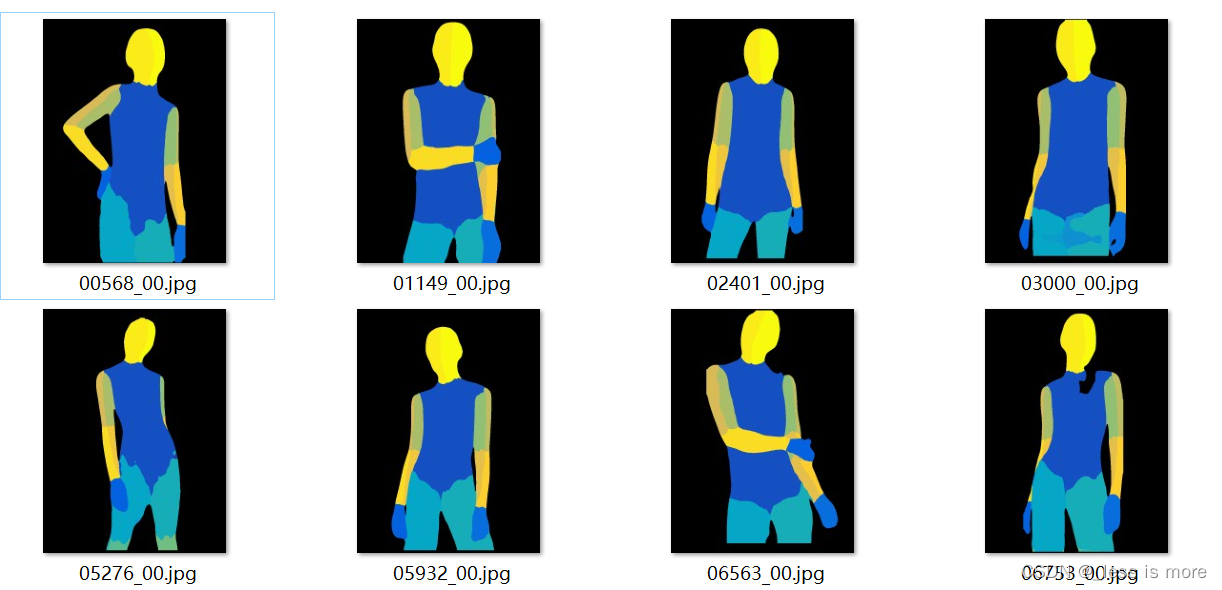
4、Cloth Mask (On colab, GPU or CPU)
This is a lot easier.
(1) Install
!pip install carvekit_colab
(2) Download models
from carvekit.ml.files.models_loc import download_all
download_all();
(3) Prepare cloth images
!mkdir ./cloth
!cp ./test/cloth/0000* ./cloth/
prepare dir for results
!mkdir ./cloth_mask
(4) Run
#title Upload images from your computer
#markdown Description of parameters
#markdown - `SHOW_FULLSIZE` - Shows image in full size (may take a long time to load)
#markdown - `PREPROCESSING_METHOD` - Preprocessing method
#markdown - `SEGMENTATION_NETWORK` - Segmentation network. Use `u2net` for hairs-like objects and `tracer_b7` for objects
#markdown - `POSTPROCESSING_METHOD` - Postprocessing method
#markdown - `SEGMENTATION_MASK_SIZE` - Segmentation mask size. Use 640 for Tracer B7 and 320 for U2Net
#markdown - `TRIMAP_DILATION` - The size of the offset radius from the object mask in pixels when forming an unknown area
#markdown - `TRIMAP_EROSION` - The number of iterations of erosion that the object's mask will be subjected to before forming an unknown area
import os
import numpy as np
from PIL import Image, ImageOps
from carvekit.web.schemas.config import MLConfig
from carvekit.web.utils.init_utils import init_interface
SHOW_FULLSIZE = False #param {type:"boolean"}
PREPROCESSING_METHOD = "none" #param ["stub", "none"]
SEGMENTATION_NETWORK = "tracer_b7" #param ["u2net", "deeplabv3", "basnet", "tracer_b7"]
POSTPROCESSING_METHOD = "fba" #param ["fba", "none"]
SEGMENTATION_MASK_SIZE = 640 #param ["640", "320"] {type:"raw", allow-input: true}
TRIMAP_DILATION = 30 #param {type:"integer"}
TRIMAP_EROSION = 5 #param {type:"integer"}
DEVICE = 'cpu' # 'cuda'
config = MLConfig(segmentation_network=SEGMENTATION_NETWORK,
preprocessing_method=PREPROCESSING_METHOD,
postprocessing_method=POSTPROCESSING_METHOD,
seg_mask_size=SEGMENTATION_MASK_SIZE,
trimap_dilation=TRIMAP_DILATION,
trimap_erosion=TRIMAP_EROSION,
device=DEVICE)
interface = init_interface(config)
imgs = []
root = '/content/cloth'
for name in os.listdir(root):
imgs.append(root + '/' + name)
images = interface(imgs)
for i, im in enumerate(images):
img = np.array(im)
img = img[...,:3] # no transparency
idx = (img[...,0]==0)&(img[...,1]==0)&(img[...,2]==0) # background 0 or 130, just try it
img = np.ones(idx.shape)*255
img[idx] = 0
im = Image.fromarray(np.uint8(img), 'L')
im.save(f'./cloth_mask/{imgs[i].split("/")[-1].split(".")[0]}.jpg')
Make sure your cloth mask results are the same size with input cloth image (768×1024). And looks like
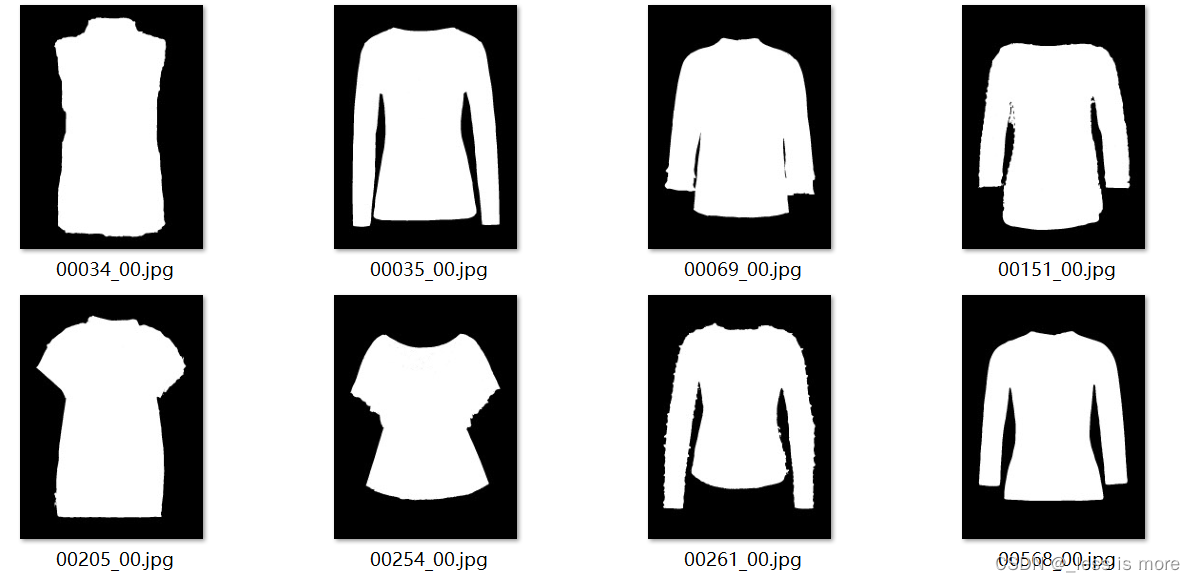
Note: you may have to change above code to get the right results, because sometimes the generated results are different, and I didn’t investigate to much about this tool. Especially the line of idx = (img[...,0]==0)&(img[...,1]==0)&(img[...,2]==0)
, you may get results of 0 or 130 as background depending on the model you use and settings.
5、Parse Agnostic (On colab)
Here is the parse label and corresponding body parts. You may need or not.
0 - 20
Background
Hat
Hair
Glove
Sunglasses
Upper-clothes
Dress
Coat
Socks
Pants
tosor-skin
Scarf
Skirt
Face
Left-arm
Right-arm
Left-leg
Right-leg
Left-shoe
Right-shoe
(1) Install packages
!pip install Pillow tqdm
(2) Prepare data
After all above steps, now you should have a data structure like this, they are under directory of test. If you are not sure which results locate in which dir, check out official dataset structure, you can download it from here.
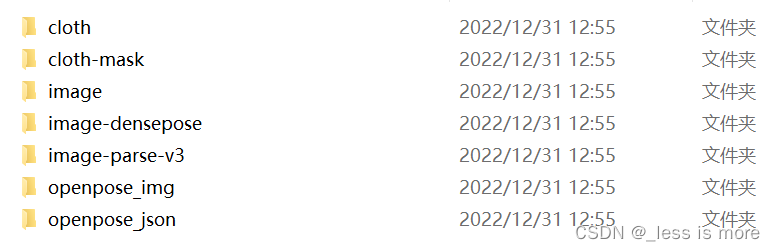
You can zip them into test.zip and unzip them on Colab with !unzip test.zip
.
Note: the images under image-parse-v3 (black images with label) are not looking the same as official data (colored images with label), the reason has been mentioned before.
(3) Run
import json
from os import path as osp
import os
import numpy as np
from PIL import Image, ImageDraw
from tqdm import tqdm
def get_im_parse_agnostic(im_parse, pose_data, w=768, h=1024):
label_array = np.array(im_parse)
parse_upper = ((label_array == 5).astype(np.float32) +
(label_array == 6).astype(np.float32) +
(label_array == 7).astype(np.float32))
parse_neck = (label_array == 10).astype(np.float32)
r = 10
agnostic = im_parse.copy()
# mask arms
for parse_id, pose_ids in [(14, [2, 5, 6, 7]), (15, [5, 2, 3, 4])]:
mask_arm = Image.new('L', (w, h), 'black')
mask_arm_draw = ImageDraw.Draw(mask_arm)
i_prev = pose_ids[0]
for i in pose_ids[1:]:
if (pose_data[i_prev, 0] == 0.0 and pose_data[i_prev, 1] == 0.0) or (pose_data[i, 0] == 0.0 and pose_data[i, 1] == 0.0):
continue
mask_arm_draw.line([tuple(pose_data[j]) for j in [i_prev, i]], 'white', width=r*10)
pointx, pointy = pose_data[i]
radius = r*4 if i == pose_ids[-1] else r*15
mask_arm_draw.ellipse((pointx-radius, pointy-radius, pointx+radius, pointy+radius), 'white', 'white')
i_prev = i
parse_arm = (np.array(mask_arm) / 255) * (label_array == parse_id).astype(np.float32)
agnostic.paste(0, None, Image.fromarray(np.uint8(parse_arm * 255), 'L'))
# mask torso & neck
agnostic.paste(0, None, Image.fromarray(np.uint8(parse_upper * 255), 'L'))
agnostic.paste(0, None, Image.fromarray(np.uint8(parse_neck * 255), 'L'))
return agnostic
if __name__ =="__main__":
data_path = './test'
output_path = './test/parse'
os.makedirs(output_path, exist_ok=True)
for im_name in tqdm(os.listdir(osp.join(data_path, 'image'))):
# load pose image
pose_name = im_name.replace('.jpg', '_keypoints.json')
try:
with open(osp.join(data_path, 'openpose_json', pose_name), 'r') as f:
pose_label = json.load(f)
pose_data = pose_label['people'][0]['pose_keypoints_2d']
pose_data = np.array(pose_data)
pose_data = pose_data.reshape((-1, 3))[:, :2]
except IndexError:
print(pose_name)
continue
# load parsing image
parse_name = im_name.replace('.jpg', '.png')
im_parse = Image.open(osp.join(data_path, 'image-parse-v3', parse_name))
agnostic = get_im_parse_agnostic(im_parse, pose_data)
agnostic.save(osp.join(output_path, parse_name))
You can check results under ./test/parse. But it’s all black as well. To ensure you are getting the right agnostic parse images, do below
import numpy as np
from PIL import Image
im_ori = Image.open('./test/image-parse-v3/06868_00.png')
im = Image.open('./test/parse/06868_00.png')
print(np.unique(np.array(im_ori)))
print(np.unique(np.array(im)))
The output may look like
[ 0 2 5 9 10 13 14 15]
[ 0 2 9 13 14 15]
The first row is longer than the second row.
You can also visualize it by
np_im = np.array(im)
np_im[np_im==2] = 151
np_im[np_im==9] = 178
np_im[np_im==13] = 191
np_im[np_im==14] = 221
np_im[np_im==15] = 246
Image.fromarray(np_im)
result may be like, which is cloth-agnostic
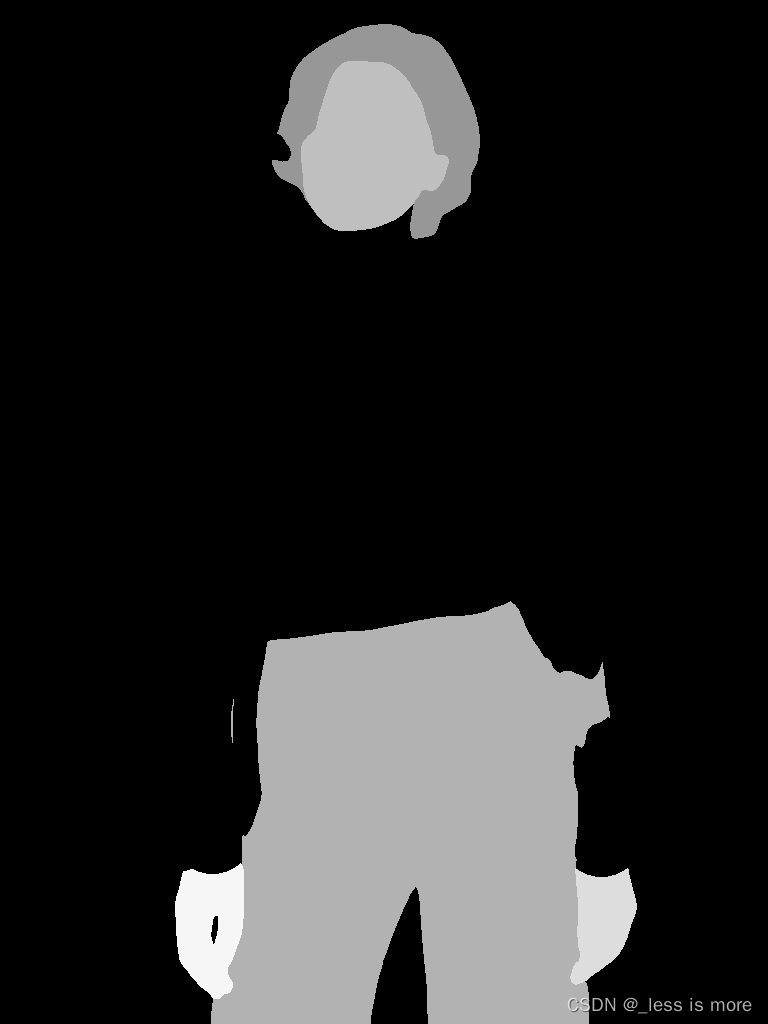
Save all the images under parse to image-parse-agnostic-v3.2
6、Human Agnostic
Steps are almost the same as above section.
(1) install
!pip install Pillow tqdm
(2) Prepare data
Now it looks like
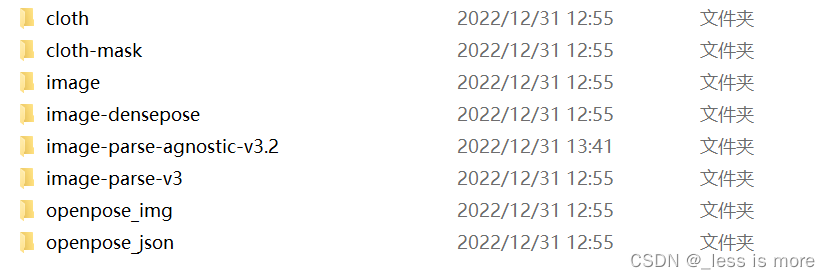
(3) Run
import json
from os import path as osp
import os
import numpy as np
from PIL import Image, ImageDraw
from tqdm import tqdm
def get_img_agnostic(img, parse, pose_data):
parse_array = np.array(parse)
parse_head = ((parse_array == 4).astype(np.float32) +
(parse_array == 13).astype(np.float32))
parse_lower = ((parse_array == 9).astype(np.float32) +
(parse_array == 12).astype(np.float32) +
(parse_array == 16).astype(np.float32) +
(parse_array == 17).astype(np.float32) +
(parse_array == 18).astype(np.float32) +
(parse_array == 19).astype(np.float32))
agnostic = img.copy()
agnostic_draw = ImageDraw.Draw(agnostic)
length_a = np.linalg.norm(pose_data[5] - pose_data[2])
length_b = np.linalg.norm(pose_data[12] - pose_data[9])
point = (pose_data[9] + pose_data[12]) / 2
pose_data[9] = point + (pose_data[9] - point) / length_b * length_a
pose_data[12] = point + (pose_data[12] - point) / length_b * length_a
r = int(length_a / 16) + 1
# mask arms
agnostic_draw.line([tuple(pose_data[i]) for i in [2, 5]], 'gray', width=r*10)
for i in [2, 5]:
pointx, pointy = pose_data[i]
agnostic_draw.ellipse((pointx-r*5, pointy-r*5, pointx+r*5, pointy+r*5), 'gray', 'gray')
for i in [3, 4, 6, 7]:
if (pose_data[i - 1, 0] == 0.0 and pose_data[i - 1, 1] == 0.0) or (pose_data[i, 0] == 0.0 and pose_data[i, 1] == 0.0):
continue
agnostic_draw.line([tuple(pose_data[j]) for j in [i - 1, i]], 'gray', width=r*10)
pointx, pointy = pose_data[i]
agnostic_draw.ellipse((pointx-r*5, pointy-r*5, pointx+r*5, pointy+r*5), 'gray', 'gray')
# mask torso
for i in [9, 12]:
pointx, pointy = pose_data[i]
agnostic_draw.ellipse((pointx-r*3, pointy-r*6, pointx+r*3, pointy+r*6), 'gray', 'gray')
agnostic_draw.line([tuple(pose_data[i]) for i in [2, 9]], 'gray', width=r*6)
agnostic_draw.line([tuple(pose_data[i]) for i in [5, 12]], 'gray', width=r*6)
agnostic_draw.line([tuple(pose_data[i]) for i in [9, 12]], 'gray', width=r*12)
agnostic_draw.polygon([tuple(pose_data[i]) for i in [2, 5, 12, 9]], 'gray', 'gray')
# mask neck
pointx, pointy = pose_data[1]
agnostic_draw.rectangle((pointx-r*7, pointy-r*7, pointx+r*7, pointy+r*7), 'gray', 'gray')
agnostic.paste(img, None, Image.fromarray(np.uint8(parse_head * 255), 'L'))
agnostic.paste(img, None, Image.fromarray(np.uint8(parse_lower * 255), 'L'))
return agnostic
if __name__ =="__main__":
data_path = './test'
output_path = './test/parse'
os.makedirs(output_path, exist_ok=True)
for im_name in tqdm(os.listdir(osp.join(data_path, 'image'))):
# load pose image
pose_name = im_name.replace('.jpg', '_keypoints.json')
try:
with open(osp.join(data_path, 'openpose_json', pose_name), 'r') as f:
pose_label = json.load(f)
pose_data = pose_label['people'][0]['pose_keypoints_2d']
pose_data = np.array(pose_data)
pose_data = pose_data.reshape((-1, 3))[:, :2]
except IndexError:
print(pose_name)
continue
# load parsing image
im = Image.open(osp.join(data_path, 'image', im_name))
label_name = im_name.replace('.jpg', '.png')
im_label = Image.open(osp.join(data_path, 'image-parse-v3', label_name))
agnostic = get_img_agnostic(im, im_label, pose_data)
agnostic.save(osp.join(output_path, im_name))
Results look like
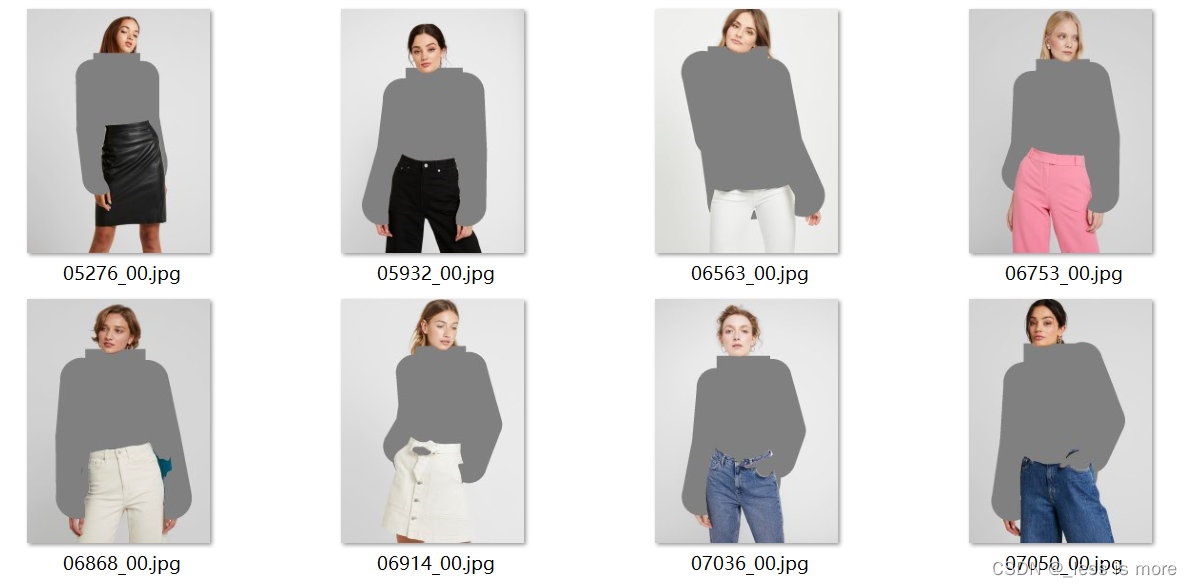
Save them to dir of agnostic-v3.2. Now you are almost done. The final structure of preprocessing results are
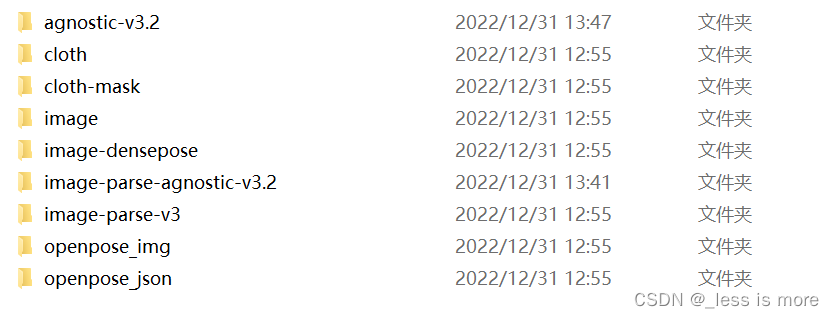
7、Conclusion
Thanks for reading. It’s not easy to get all this done. Before you run HR-VITON with you preprocessed dataset, note that each person image need a corresponding cloth image even though it’s not used while inference. If you don’t want this behavior, you can either change the source code manually
or just add some random images with the same name of person images
. After all done, suppose you are testing 5 people images and 3 cloth images, which are all unpaired, you should end up with 3 images under cloth
dir and 3 images under cloth-mask
; and 5 images under each other dirs: agnostic-v3.2
, image
, image-densepose
, image-parse-agnostic-v3.2
, image-parse-v3
, openpose_img
, and openpose_json
.
Final test result