学习SpringAI的记录情况
文章目录
前言
因公司需要故而学习SpringAI文档,故将自己所见所想写成文章,供大佬们参考
主要是为什么这么写呢,为何不抽出来呢,还是希望可以用的时候更加方便一点,如果大家有需求可以自行去优化。
SrpingAI入门
这里我用到的是智普AI,大家可以根据自己喜欢用的AI自由进行切换
至于API-KEY可自行去智谱AI开放平台可自行去找,这里新用户注册目前是还送2000万TOKEN的
引包
<!--智普AI-->
<dependency>
<groupId>org.springframework.ai</groupId>
<artifactId>spring-ai-zhipuai-spring-boot-starter</artifactId>
</dependency>
配置文件
至于API-KEY可自行去智谱AI开放平台可自行去找,这里新用户注册目前是还送2000万TOKEN的
配置类
获取API-KEY
@Component
public class ChatConfig {
//获取配置文件中的API-KEY
@Value("${spring.ai.zhipuai.api-key}")
private String apiKey;
public String getApiKey() {
return apiKey;
}
}
测试用例代码
//为啥用这个模型呢,因为免费
private static final String default_model = "GLM-4-Flash";
//temperature 是一个超参数,用于控制生成文本的多样性和随机性。
//低温度(例如:0.1 到 0.3):模型会生成更确定、常规和一致的输出。低温度通常会使模型产生更
加保守的回答,重复性较高,生成的内容更加准确、接近训练数据中的常见模式。
//高温度(例如:0.7 到 1.0):模型的输出会更加随机、多样、创意性强。高温度会导致生成的内容
更加多样化,可能包括一些不太常见或创新性的回答,但也可能带来不太准确或不太连贯的结果。
private static final double default_temperature = 0.7;
@GetMapping("/AIchat")
public BaseResponse<String> AIGeneration(
@RequestParam(value = "message", defaultValue = "你是谁呢") String message) {
// 创建ZhiPuAiChatOptions对象,设置模型和温度
ZhiPuAiChatOptions options = ZhiPuAiChatOptions.builder()
.withModel(default_model)
.withTemperature(default_temperature)
.build();
// 创建ZhiPuAiChatModel对象,传入ZhiPuAiApi和options
ChatModel chatModel = new ZhiPuAiChatModel(new
ZhiPuAiApi(chatConfig.getApiKey()), options);
// 创建ChatClient对象,传入chatModel
ChatClient build = ChatClient.builder(chatModel).build();
String substring = "";
String content = null;
try {
// 调用build.prompt()方法,传入用户消息,调用call()方法,获取返回内容
content = build.prompt()
.user(message)
.call()
.content();
} catch (Exception e) {
// 获取异常信息
String errorMessage = e.getMessage();
// 获取异常信息中冒号后面的内容
int i = errorMessage.lastIndexOf(":");
String newString = errorMessage.substring(i + 2);
// 去掉异常信息中的最后一个字符
substring = newString.substring(0, newString.length() - 3);
}
// 如果异常信息不为空,抛出BusinessException异常
if(!substring.isEmpty()){
throw new BusinessException(ErrorCode.PARAMS_ERROR,substring);
}
// 返回成功结果
return ResultUtils.success(content);
}
测试AI返回结果
全参数响应结果
这里是对token的消耗做了一个统计,查看用户的所剩token情况的一个返回结果展示
其实也就只是把String类型的返回结果转成了ChatResponse类型仅此而已
测试用例代码
@GetMapping("/AIchat")
public BaseResponse<ChatResponse> AIGeneration(
@RequestParam(value = "message", defaultValue = "你是谁呢") String message) {
// 创建ZhiPuAiChatOptions对象,设置模型和温度
ZhiPuAiChatOptions options = ZhiPuAiChatOptions.builder()
.withModel(default_model)
.withTemperature(default_temperature)
.build();
// 创建ZhiPuAiChatModel对象,传入ZhiPuAiApi和options
ChatModel chatModel = new ZhiPuAiChatModel(new ZhiPuAiApi(chatConfig.getApiKey()), options);
// 创建ChatClient对象,传入chatModel
ChatClient build = ChatClient.builder(chatModel).build();
String substring = "";
ChatResponse chatResponse = null;
try {
chatResponse = build.prompt()
.user(message)
.call()
.chatResponse();
} catch (Exception e) {
// 获取异常信息
String errorMessage = e.getMessage();
// 获取异常信息中冒号后面的内容
int i = errorMessage.lastIndexOf(":");
String newString = errorMessage.substring(i + 2);
// 去掉异常信息中的最后一个字符
substring = newString.substring(0, newString.length() - 3);
}
// 如果异常信息不为空,抛出BusinessException异常
if(!substring.isEmpty()){
throw new BusinessException(ErrorCode.PARAMS_ERROR,substring);
}
// 返回成功结果
return ResultUtils.success(chatResponse);
}
返回结果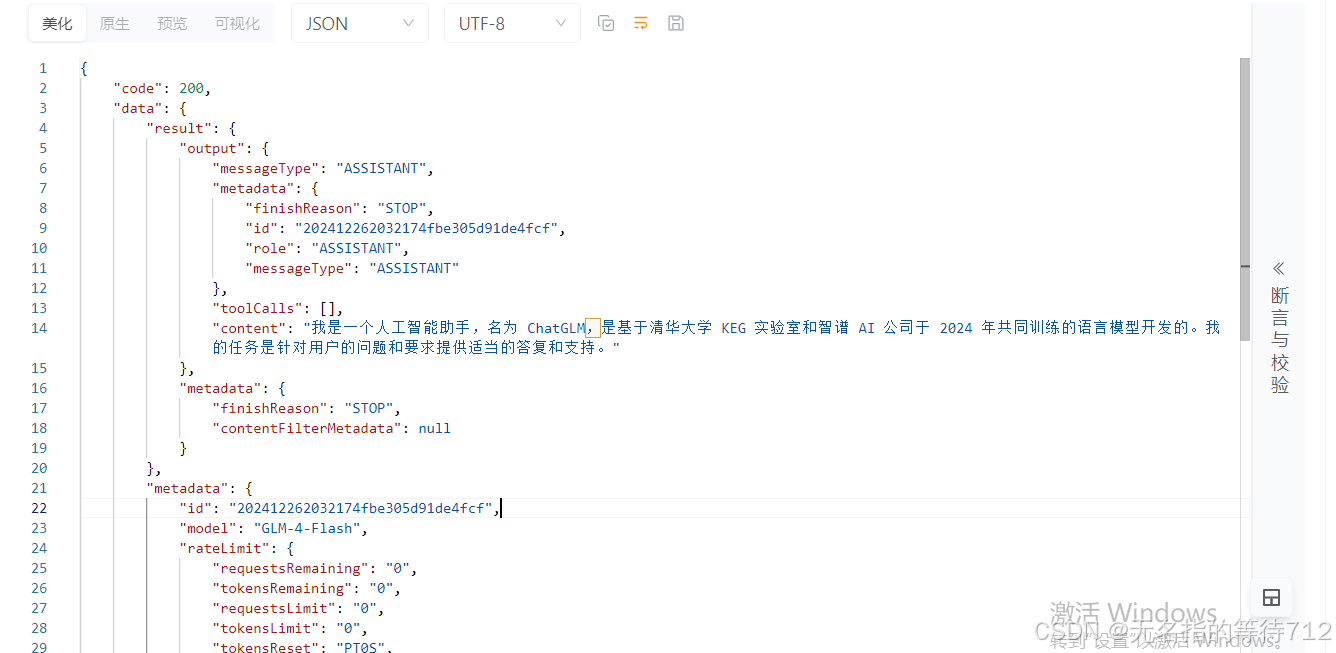
前端传递不同的模型测试方法
测试用例代码
@GetMapping("/chat")
public BaseResponse<String> generation(
@RequestParam(value = "message", defaultValue = "你是谁呢") String message,
@RequestParam(value = "model", defaultValue = "GLM-4-Flash") String model,
@RequestParam(value = "temperature", defaultValue = "0.7") double temperature) {
// 创建新的 ZhiPuAiChatOptions 实例,根据请求的参数动态设置
ZhiPuAiChatOptions options = ZhiPuAiChatOptions.builder()
.withModel(model)
.withTemperature(temperature)
.build();
// 获取 ChatModel 实例,使用新的配置
ChatModel chatModel = new ZhiPuAiChatModel(new
ZhiPuAiApi(chatConfig.getApiKey()), options);
ChatClient build = ChatClient.builder(chatModel).build();
String substring = "";
// 使用新的 ChatModel 进行对话生成
String content = null;
try {
content = build.prompt()
.user(message)
.call()
.content();
} catch (Exception e) {
// 获取错误消息
String errorMessage = e.getMessage();
int i = errorMessage.lastIndexOf(":");
String newString = errorMessage.substring(i + 2);
substring = newString.substring(0, newString.length() - 3);
}
if(!substring.isEmpty()){
throw new BusinessException(ErrorCode.PARAMS_ERROR,substring);
}
return ResultUtils.success(content);
}
其实跟上面的代码差不多,只不过是从前端传递过来一个模型,当然这里我并没有对于上传上来的模型做校验,如果感兴趣的话,可自行查看,并解决
测试结果
这里可以看到我们的异常返回起到效果了,原谅我是一个穷鬼,不想花钱在测试这个上面
测试到这里,相信你已经知道了点什么,就是如果你问的问题较长的话,实际使用GET传输是有问题的,因为默认GET请求的长度是有限制的,建议自行换成POST请求,然后再继续往下
流式响应
测试用例代码
@GetMapping(value = "/AIStream",produces = MediaType.TEXT_EVENT_STREAM_VALUE)
public Flux<String> AIStream(
@RequestParam(value = "message", defaultValue = "你是谁呢") String message) {
// 创建ZhiPuAiChatOptions对象,设置模型和温度
ZhiPuAiChatOptions options = ZhiPuAiChatOptions.builder()
.withModel(default_model)
.withTemperature(default_temperature)
.build();
// 创建ZhiPuAiChatModel对象,传入ZhiPuAiApi和options
ChatModel chatModel = new ZhiPuAiChatModel(new ZhiPuAiApi(chatConfig.getApiKey()), options);
// 创建ChatClient对象,传入chatModel
ChatClient chatClient = ChatClient.builder(chatModel).build();
Flux<String> content = chatClient.prompt()
.user(message)
.stream()
.content();
// 返回成功结果
return content;
}
响应结果
这里使用的是SSE,SSE是一种服务端向客户端推送的一种技术,需要的可自行去浏览这个技术
流式响应全参数
测试用例代码
// 使用GetMapping注解,指定请求路径为/AIChatResponseStream,返回类型为TEXT_EVENT_STREAM_VALUE
@GetMapping(value = "/AIChatResponseStream",produces = MediaType.TEXT_EVENT_STREAM_VALUE)
// 定义一个方法,返回类型为Flux<ChatResponse>,参数为String类型的message,默认值为"你是谁呢"
public Flux<ChatResponse> AIChatResponseStream(
@RequestParam(value = "message", defaultValue = "你是谁呢") String message) {
// 创建ZhiPuAiChatOptions对象,设置模型和温度
ZhiPuAiChatOptions options = ZhiPuAiChatOptions.builder()
.withModel(default_model)
.withTemperature(default_temperature)
.build();
// 创建ChatModel对象,传入ZhiPuAiApi和options
ChatModel chatModel = new ZhiPuAiChatModel(new ZhiPuAiApi(chatConfig.getApiKey()), options);
// 创建ChatClient对象,传入chatModel
ChatClient chatClient = ChatClient.builder(chatModel).build();
// 调用chatClient的prompt方法,传入message,返回一个Flux<ChatResponse>对象
Flux<ChatResponse> chatResponseFlux = chatClient.prompt()
.user(message)
.stream()
.chatResponse();
// 返回Flux<ChatResponse>对象
return chatResponseFlux;
}
响应结果
至于这里如何查看内容流式响应全参数输出完毕,有一个STOP的停止参数可以使用
位置贴到下面
//全参数流失响应地址接口停止标志--------------当然这里如果你要配合前端可以通过这个STOP来观察结果是否输出完毕,可以通过这个标志来停掉SSE连接 parsedData.results[0].metadata.finishReason === "STOP"
默认角色回复实现
测试用例代码
// 使用GetMapping注解,指定请求路径为/AIChatDefaultStream,返回类型为文本流
@GetMapping(value = "/AIChatDefaultStream",produces = MediaType.TEXT_EVENT_STREAM_VALUE)
public Flux<String> AIChatDefaultStream(
// 使用@RequestParam注解,指定请求参数名为message,默认值为"你是谁呢"
@RequestParam(value = "message", defaultValue = "你是谁呢") String message) {
// 创建ZhiPuAiChatOptions对象,设置模型和温度
ZhiPuAiChatOptions options = ZhiPuAiChatOptions.builder()
.withModel(default_model)
.withTemperature(default_temperature)
.build();
// 创建ChatModel对象,使用ZhiPuAiChatModel和ZhiPuAiApi
ChatModel chatModel = new ZhiPuAiChatModel(new ZhiPuAiApi(chatConfig.getApiKey()), options);
// 创建ChatClient对象,设置默认系统
ChatClient chatClient = ChatClient.builder(chatModel).defaultSystem("你现在的身份是一个星轨会议系统的客服助手,请以友好、乐于助人且愉快的方式来回复。").build();
// 调用chatClient的prompt方法,设置用户消息,并返回内容流
Flux<String> content = chatClient.prompt()
.user(message)
.stream()
.content();
// 返回内容流
return content;
}
响应结果
这里就可以看到响应结果他已经换成了星轨会议系统的客服助手,如果你希望做某一个角色的内容,你可以通过预训练这个参数,使得这个AI客服助手的回复更加友好。
AI知晓日期
测试用例代码
// 使用GetMapping注解,指定请求路径为/AIChatDefaultStream,返回类型为文本流
@GetMapping(value = "/AIChatDefaultStream",produces = MediaType.TEXT_EVENT_STREAM_VALUE)
public Flux<String> AIChatDefaultStream(
// 使用@RequestParam注解,指定请求参数名为message,默认值为"你是谁呢"
@RequestParam(value = "message", defaultValue = "你是谁呢") String message) {
// 创建ZhiPuAiChatOptions对象,设置模型和温度
ZhiPuAiChatOptions options = ZhiPuAiChatOptions.builder()
.withModel(default_model)
.withTemperature(default_temperature)
.build();
// 创建ChatModel对象,使用ZhiPuAiChatModel和ZhiPuAiApi
ChatModel chatModel = new ZhiPuAiChatModel(new ZhiPuAiApi(chatConfig.getApiKey()), options);
// 创建ChatClient对象,设置默认系统
ChatClient chatClient = ChatClient.builder(chatModel).defaultSystem("你现在的身份是一个星轨会议系统的客服助手,请以友好、乐于助人且愉快的方式来回复。我希望你可以以中文的方式给我回答。今天的日期是 {current_date}.")
.build();
// 调用chatClient的prompt方法,设置用户消息,并返回内容流
Flux<String> content = chatClient.prompt()
.user(message)
.system(s->s.param("current_date", LocalDate.now().toString()))
.stream()
.content();
// 返回内容流
return content;
}
响应结果
这样的话AI就知道当前的日期
记忆对话
测试用例代码
创建一个配置类引入ChatMemory
@Configuration
public class ZhiPuChatMemoryConfig {
@Bean
@Qualifier("customMemory")
public ChatMemory chatMemory() {
return new InMemoryChatMemory();
}
}
引入ChatMemory
// 使用GetMapping注解,指定请求路径为/AIChatDefaultStream,返回类型为文本流
@GetMapping(value = "/AIChatDefaultStream",produces = MediaType.TEXT_EVENT_STREAM_VALUE)
public Flux<String> AIChatDefaultStream(
// 使用RequestParam注解,指定请求参数名为message,类型为String
@RequestParam(value = "message") String message) {
// 创建ZhiPuAiChatOptions对象,设置模型和温度
ZhiPuAiChatOptions options = ZhiPuAiChatOptions.builder()
.withModel(default_model)
.withTemperature(default_temperature)
.build();
// 创建ChatModel对象,传入ZhiPuAiApi和options
ChatModel chatModel = new ZhiPuAiChatModel(new ZhiPuAiApi(chatConfig.getApiKey()), options);
// 创建ChatClient对象,传入chatModel,设置默认系统消息和默认顾问
ChatClient chatClient = ChatClient.builder(chatModel).defaultSystem("你现在的身份是一个星轨会议系统的客服助手,请以友好、乐于助人且愉快的方式来回复。我希望你可以以中文的方式给我回答。这里我问你日期的话再回答,否则的话你不要回答,今天的日期是 {current_date}.")
//.defaultAdvisors(new PromptChatMemoryAdvisor(chatMemory))
.defaultAdvisors(new MessageChatMemoryAdvisor(chatMemory))
.build();
// 调用chatClient的prompt方法,设置用户消息、系统消息参数、顾问参数,返回内容流
Flux<String> content = chatClient.prompt()
.user(message)
.system(s->s.param("current_date", LocalDate.now().toString()))
.advisors(a -> a.param(CHAT_MEMORY_RETRIEVE_SIZE_KEY, 100))
.stream()
.content();
// 返回内容流
return content;
}
响应结果
这样的话就会记住你最近的100条对话,当然这里你也可以自行实现ChatMemory,使用Redis去进行获取
上面两种记忆是不同的,具体详情,请看下表
特性 | MessageChatMemoryAdvisor | PromptChatMemoryAdvisor |
---|---|---|
记忆管理粒度 | 基于消息(Message)级别的记忆 | 基于提示(Prompt)级别的记忆 |
记忆的存储方式 | 将每一条消息存储到记忆中 | 生成合适的提示,将历史对话作为提示提供给模型 |
适用场景 | 适用于需要精细管理每一条消息的场景 | 适用于需要生成合适提示来为模型提供上下文的场景 |
如何影响对话 | 直接影响聊天记录,确保每一条消息都被记住 | 影响提示构造,确保生成的提示与上下文一致 |
内存管理的灵活性 | 依赖于每个消息的存储 | 提示的构造和上下文的动态管理 |
打印日志功能
测试用例代码
其实也就是再次添加一个Advisors,再控制台打印一下就可以
public class LoggingAdvisors implements RequestResponseAdvisor {
@Override
public AdvisedRequest adviseRequest(AdvisedRequest request, Map<String, Object> adviseContext) {
System.out.println("Request"+request);
return request;
}
@Override
public int getOrder() {
return 0;
}
}
// 使用GetMapping注解,指定请求路径为/AIChatDefaultStream,返回类型为文本流
@GetMapping(value = "/AIChatDefaultLoggingStream",produces = MediaType.TEXT_EVENT_STREAM_VALUE)
public Flux<String> AIChatDefaultLoggingStream(
// 使用RequestParam注解,指定请求参数名为message,类型为String
@RequestParam(value = "message") String message) {
// 创建ZhiPuAiChatOptions对象,设置模型和温度
ZhiPuAiChatOptions options = ZhiPuAiChatOptions.builder()
.withModel(default_model)
.withTemperature(default_temperature)
.build();
// 创建ChatModel对象,传入ZhiPuAiApi和options
ChatModel chatModel = new ZhiPuAiChatModel(new ZhiPuAiApi(chatConfig.getApiKey()), options);
// 创建ChatClient对象,传入chatModel,设置默认系统消息和默认顾问
ChatClient chatClient = ChatClient.builder(chatModel).defaultSystem("你现在的身份是一个星轨会议系统的客服助手,请以友好、乐于助人且愉快的方式来回复。我希望你可以以中文的方式给我回答。这里我问你日期的话再回答,否则的话你不要回答,今天的日期是 {current_date}.")
//.defaultAdvisors(new PromptChatMemoryAdvisor(chatMemory))
.defaultAdvisors(new MessageChatMemoryAdvisor(chatMemory),new LoggingAdvisors())
.build();
// 调用chatClient的prompt方法,设置用户消息、系统消息参数、顾问参数,返回内容流
Flux<String> content = chatClient.prompt()
.user(message)
.system(s->s.param("current_date", LocalDate.now().toString()))
.advisors(a -> a.param(CHAT_MEMORY_RETRIEVE_SIZE_KEY, 100))
.stream()
.content();
// 返回内容流
return content;
}
响应结果
FunctionCall回调
测试用例代码
package com.hhh.springai_test.Client;
import com.hhh.springai_test.config.ChatConfig;
import jakarta.annotation.Resource;
import org.springframework.ai.chat.client.ChatClient;
import org.springframework.ai.chat.client.advisor.MessageChatMemoryAdvisor;
import org.springframework.ai.chat.memory.ChatMemory;
import org.springframework.ai.chat.model.ChatModel;
import org.springframework.ai.zhipuai.ZhiPuAiChatModel;
import org.springframework.ai.zhipuai.ZhiPuAiChatOptions;
import org.springframework.ai.zhipuai.api.ZhiPuAiApi;
import org.springframework.beans.factory.annotation.Autowired;
import org.springframework.beans.factory.annotation.Qualifier;
import org.springframework.stereotype.Component;
@Component
public class ZhipuChatClient {
@Resource
private ChatConfig chatConfig;
@Autowired
@Qualifier("customMemory") // 明确指定使用你自定义的 ChatMemory 实现
private ChatMemory chatMemory;
public ChatClient getZhipuChatClient() {
ZhiPuAiChatOptions options = ZhiPuAiChatOptions.builder()
.withModel("GLM-4-Flash")
.withTemperature(0.95F)
.build();
// 创建ChatModel对象,传入ZhiPuAiApi和options
ChatModel chatModel = new ZhiPuAiChatModel(new ZhiPuAiApi(chatConfig.getApiKey()), options);
// 创建ChatClient对象,传入chatModel,设置默认系统消息和默认顾问
ChatClient chatClient = ChatClient.builder(chatModel).defaultSystem("你现在的身份是一个星轨会议系统的客服助手,请以友好、乐于助人且愉快的方式来回复。我希望你可以以中文的方式给我回答。这里我问你日期的话再回答,否则的话你不要回答")
.defaultAdvisors(new MessageChatMemoryAdvisor(chatMemory))
.build();
return chatClient;
}
}
@GetMapping(value = "/AIChatDefaultLoggingStream", produces = MediaType.TEXT_EVENT_STREAM_VALUE)
public Flux<String> AIChatDefaultLoggingStream(@RequestParam(value = "message") String message) {
Flux<String> content = zhipuChatClient.getZhipuChatClient().prompt()
.user(message)
.function("report", "举报", new Function<MyController.Request, Flux<String>>() {
@Override
public Flux<String> apply(MyController.Request request) {
System.out.println("举报名字是" + request.name);
return Flux.just("星轨");
}
})
.stream()
.content();
return content;
}
测试用例结果
RAG知识库
测试用例代码
package com.hhh.springai_test.Advisor;
import org.springframework.ai.document.Document;
import org.springframework.ai.reader.tika.TikaDocumentReader;
import org.springframework.ai.vectorstore.SearchRequest;
import org.springframework.ai.vectorstore.VectorStore;
import org.springframework.core.io.FileSystemResource;
import org.springframework.stereotype.Component;
import java.util.ArrayList;
import java.util.List;
import java.util.Optional;
@Component
public class ZhiPuVectorStore implements VectorStore {
private final List<Document> documentList = new ArrayList<>();
@Override
public void add(List<Document> documents) {
if(documents != null && !documents.isEmpty()){
if (documents != null && !documents.isEmpty()) {
documentList.addAll(documents);
documentList.forEach(document -> System.out.println("Document added: " + document.getContent()));
System.out.println("Documents added successfully: " + documents.size());
} else {
System.out.println("No documents to add.");
}
}
}
@Override
public Optional<Boolean> delete(List<String> idList) {
if (idList == null || idList.isEmpty()) {
return Optional.of(false);
}
boolean deleted = documentList.removeIf(doc -> idList.contains(doc.getId()));
return Optional.of(deleted);
}
@Override
public List<Document> similaritySearch(SearchRequest request) {
System.out.println("Performing similarity search: " + request.getQuery());
return new ArrayList<>();
}
public void readAndAddDocuments(String filePath) {
try {
FileSystemResource resource = new FileSystemResource(filePath);
List<Document> documents = new TikaDocumentReader(resource).read();
this.add(documents);
} catch (Exception e) {
System.err.println("Error reading and adding documents: " + e.getMessage());
}
}
}
private final ZhiPuVectorStore zhiPuVectorStore;
public MyController(ZhiPuVectorStore zhiPuVectorStore) {
this.zhiPuVectorStore = zhiPuVectorStore;
}
@GetMapping(value = "/AIChatRagStream", produces = MediaType.TEXT_EVENT_STREAM_VALUE)
public Flux<String> AIChatRagStream(@RequestParam(value = "message") String message) {
String path = "src/main/resources/static/jubao.txt";
zhiPuVectorStore.readAndAddDocuments(path);
Flux<String> content = zhipuChatClient.getZhipuChatClient().prompt()
.user(message)
.advisors(new QuestionAnswerAdvisor(zhiPuVectorStore, SearchRequest.defaults()))
.stream()
.content();
return content;
}
测试用例结果
可以看到日志也已经解决了,在这里遇到了整整两天的bug,一直在尝试使用functions,通过传递functionBean来进行解决,但是一直是有问题的,但是一直报错,只能使用这种方式来解决,当然,如果大佬们有好的文章,记得可以推荐我使用一下,谢谢各位大佬们