⭐欢迎大家订阅我的专栏一起学习⭐
🚀🚀🚀订阅专栏,更新及时查看不迷路🚀🚀🚀
YOLOv5涨点专栏:http://t.csdnimg.cn/QdCj6
YOLOv7专栏: http://t.csdnimg.cn/dygOj
YOLOv8涨点专栏:http://t.csdnimg.cn/Avu8g
💡魔改网络、复现论文、优化创新💡
目录
主要想法
GSConv
GSConv代码实现
slim-neck
slim-neck代码实现
yaml文件
完整代码分享
总结
目标检测是计算机视觉中重要的下游任务。对于车载边缘计算平台来说,巨大的模型很难达到实时检测的要求。而且,由大量深度可分离卷积层构建的轻量级模型无法达到足够的精度。我们引入了一种新的轻量级卷积技术 GSConv,以减轻模型重量但保持准确性。 GSConv 在模型的准确性和速度之间实现了出色的权衡。并且,我们提供了一种设计范例,细颈,以实现探测器更高的计算成本效益。我们的方法的有效性在二十多组比较实验中得到了强有力的证明。特别是,与原始检测器相比,通过我们的方法改进的检测器获得了最先进的结果(例如,在公开数据集的Tesla T4 GPU 上以100FPS 的速度获得 70.9% mAP0.5)。
主要想法
生物大脑处理信息的强大能力和低能耗远远超出了计算机。简单地无休止地增加模型参数的数量并不能建立强大的模型。轻量化设计可以有效缓解现阶段高昂的计算成本。这个目的主要是通过深度可分离卷积(DSC)运算来减少参数量和浮点运算(FLOP)来实现的,效果很明显。然而DSC的缺点也很明显:在计算过程中输入图像的通道信息被分离。这一缺陷导致 DSC 的特征提取和融合能力比标准卷积 (SC) 低得多。
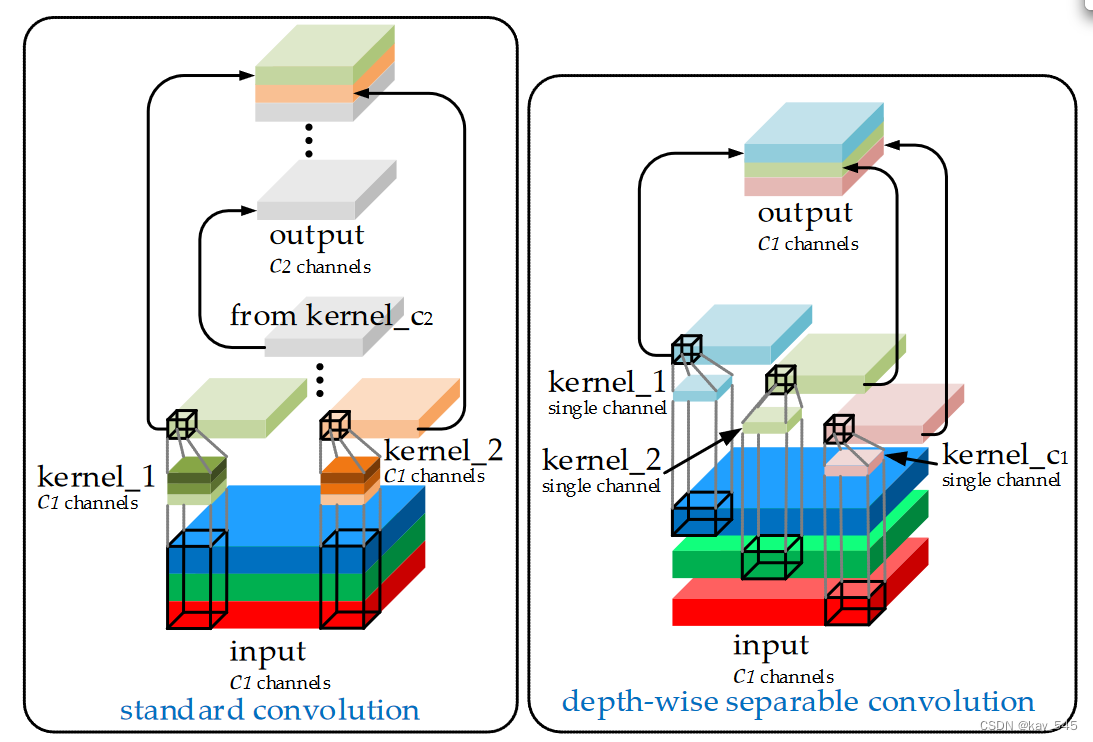
GSConv
尽管DSC有一定的优点,但DSC 的缺陷在主干中直接被放大,无论是用于图像分类还是检测。我们相信SC和DSC可以合作。我们注意到,仅通过混洗 DSC 输出通道生成的特征图仍然是“深度分离”。为了使DSC的输出尽可能接近SC,我们引入了一种新方法——SC、DSC和shuffle的混合卷积,命名为GSConv。如图所示,我们使用shuffle将SC(通道密集卷积运算)生成的信息渗透到DSC生成的信息的每个部分中。shuffle是一种统一的混合策略。该方法通过在不同通道上统一交换局部特征信息,可以将来自 SC 的信息完全混合到 DSC 的输出中,而无需任何附加功能。
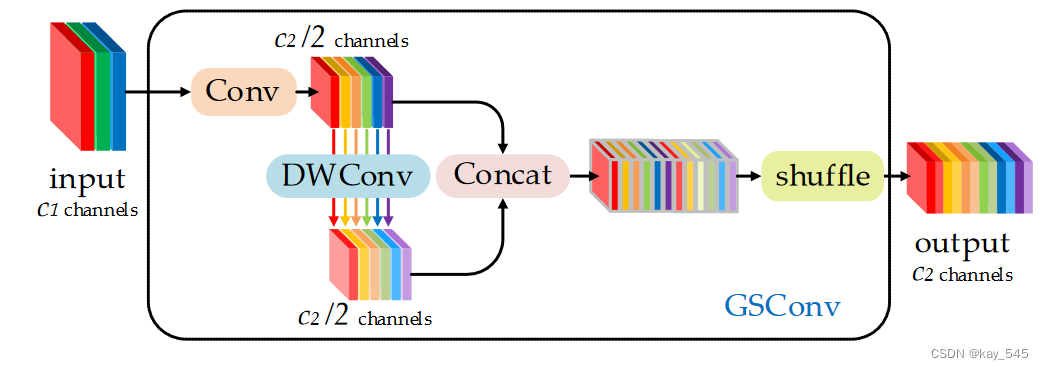
GSConv代码实现
import torch
import torch.nn as nn
import math
# GSConvE test
class GSConvE(nn.Module):
'''
GSConv enhancement for representation learning: generate various receptive-fields and
texture-features only in one Conv module
https://github.com/AlanLi1997/slim-neck-by-gsconv
'''
def __init__(self, c1, c2, k=1, s=1, g=1, act=True):
super().__init__()
c_ = c2 // 4
self.cv1 = Conv(c1, c_, k, s, None, g, act)
self.cv2 = Conv(c_, c_, 9, 1, None, c_, act)
self.cv3 = Conv(c_, c_, 13, 1, None, c_, act)
self.cv4 = Conv(c_, c_, 17, 1, None, c_, act)
def forward(self, x):
x1 = self.cv1(x)
x2 = self.cv2(x1)
x3 = self.cv3(x1)
x4 = self.cv4(x1)
y = torch.cat((x1, x2, x3, x4), dim=1)
# shuffle
y = y.reshape(y.shape[0], 2, y.shape[1] // 2, y.shape[2], y.shape[3])
y = y.permute(0, 2, 1, 3, 4)
return y.reshape(y.shape[0], -1, y.shape[3], y.shape[4])
def autopad(k, p=None): # kernel, padding
# Pad to 'same'
if p is None:
p = k // 2 if isinstance(k, int) else [x // 2 for x in k] # auto-pad
return p
class Conv(nn.Module):
# C_B_M
def __init__(self, c1, c2, k=1, s=1, p=None, g=1, act=True):
super().__init__()
self.conv = nn.Conv2d(c1, c2, k, s, autopad(k, p), groups=g, bias=False)
self.bn = nn.BatchNorm2d(c2)
self.act = nn.Mish() if act else (act if isinstance(act, nn.Module) else nn.Identity())
def forward(self, x):
return self.act(self.bn(self.conv(x)))
def forward_fuse(self, x):
return self.act(self.conv(x))
class GSConv(nn.Module):
# GSConv https://github.com/AlanLi1997/slim-neck-by-gsconv
def __init__(self, c1, c2, k=1, s=1, g=1, act=True):
super().__init__()
c_ = c2 // 2
self.cv1 = Conv(c1, c_, k, s, None, g, act)
self.cv2 = Conv(c_, c_, 5, 1, None, c_, act)
def forward(self, x):
x1 = self.cv1(x)
x2 = torch.cat((x1, self.cv2(x1)), 1)
# shuffle
y = x2.reshape(x2.shape[0], 2, x2.shape[1] // 2, x2.shape[2], x2.shape[3])
y = y.permute(0, 2, 1, 3, 4)
return y.reshape(y.shape[0], -1, y.shape[3], y.shape[4])
class GSConvns(GSConv):
# GSConv with a normative-shuffle https://github.com/AlanLi1997/slim-neck-by-gsconv
def __init__(self, c1, c2, k=1, s=1, g=1, act=True):
super().__init__(c1, c2, k=1, s=1, g=1, act=True)
c_ = c2 // 2
self.shuf = nn.Conv2d(c_ * 2, c2, 1, 1, 0, bias=False)
def forward(self, x):
x1 = self.cv1(x)
x2 = torch.cat((x1, self.cv2(x1)), 1)
# normative-shuffle, TRT supported
return nn.ReLU(self.shuf(x2))
class GSBottleneck(nn.Module):
# GS Bottleneck https://github.com/AlanLi1997/slim-neck-by-gsconv
def __init__(self, c1, c2, k=3, s=1):
super().__init__()
c_ = c2 // 2
# for lighting
self.conv_lighting = nn.Sequential(
GSConv(c1, c_, 1, 1),
GSConv(c_, c2, 3, 1, act=False))
self.shortcut = Conv(c1, c2, 1, 1, act=False)
def forward(self, x):
return self.conv_lighting(x) + self.shortcut(x)
class DWConv(Conv):
# Depth-wise convolution class
def __init__(self, c1, c2, k=1, s=1, act=True): # ch_in, ch_out, kernel, stride, padding, groups
super().__init__(c1, c2, k, s, g=math.gcd(c1, c2), act=act)
class GSBottleneckC(GSBottleneck):
# cheap GS Bottleneck https://github.com/AlanLi1997/slim-neck-by-gsconv
def __init__(self, c1, c2, k=3, s=1):
super().__init__(c1, c2, k, s)
self.shortcut = DWConv(c1, c2, 3, 1, act=False)
class VoVGSCSP(nn.Module):
# VoVGSCSP module with GSBottleneck
def __init__(self, c1, c2, n=1, shortcut=True, g=1, e=0.5):
super().__init__()
c_ = int(c2 * e) # hidden channels
self.cv1 = Conv(c1, c_, 1, 1)
self.cv2 = Conv(c1, c_, 1, 1)
# self.gc1 = GSConv(c_, c_, 1, 1)
# self.gc2 = GSConv(c_, c_, 1, 1)
self.gsb = GSBottleneck(c_, c_, 1, 1)
self.res = Conv(c_, c_, 3, 1, act=False)
self.cv3 = Conv(2*c_, c2, 1) #
def forward(self, x):
x1 = self.gsb(self.cv1(x))
y = self.cv2(x)
return self.cv3(torch.cat((y, x1), dim=1))
slim-neck
此外,还研究了增强 CNN 学习能力的通用方法,例如 DensNet 、VoVNet 和 CSPNet ,然后根据这些方法的理论设计 slim-neck 的结构。我们设计了细长的颈部,以降低检测器的计算复杂性和推理时间,但保持精度。 GSConv完成了降低计算复杂度的任务,而减少推理时间并保持精度的任务需要新的模型。
GSConv的计算成本约为SC的50%(0.5+0.5C1,C1值越大,比例越接近50%),但其对模型学习能力的贡献与后者相当。基于GSConv,我们在GSConv的基础上继续引入GS瓶颈,下图(a)展示了GS瓶颈模块的结构。然后,我们使用一次性聚合方法设计跨阶段部分网络(GSCSP)模块VoV-GSCSP。图(b)(c)和(d)分别显示了我们为VoV-GSCSP提供的三种设计方案,其中(b)简单直接且推理速度更快,(c)和(d)具有功能的重用率更高。事实上,结构越简单的模块由于硬件友好而更容易被使用。下表也详细报告了VoV-GSCSP1、2、3三种结构的消融研究结果,事实上,VoVGSCSP1表现出更高的性价比。最后,我们需要灵活地使用 GSConv、GS 瓶颈和 VoV-GSCSP 这四个模块。
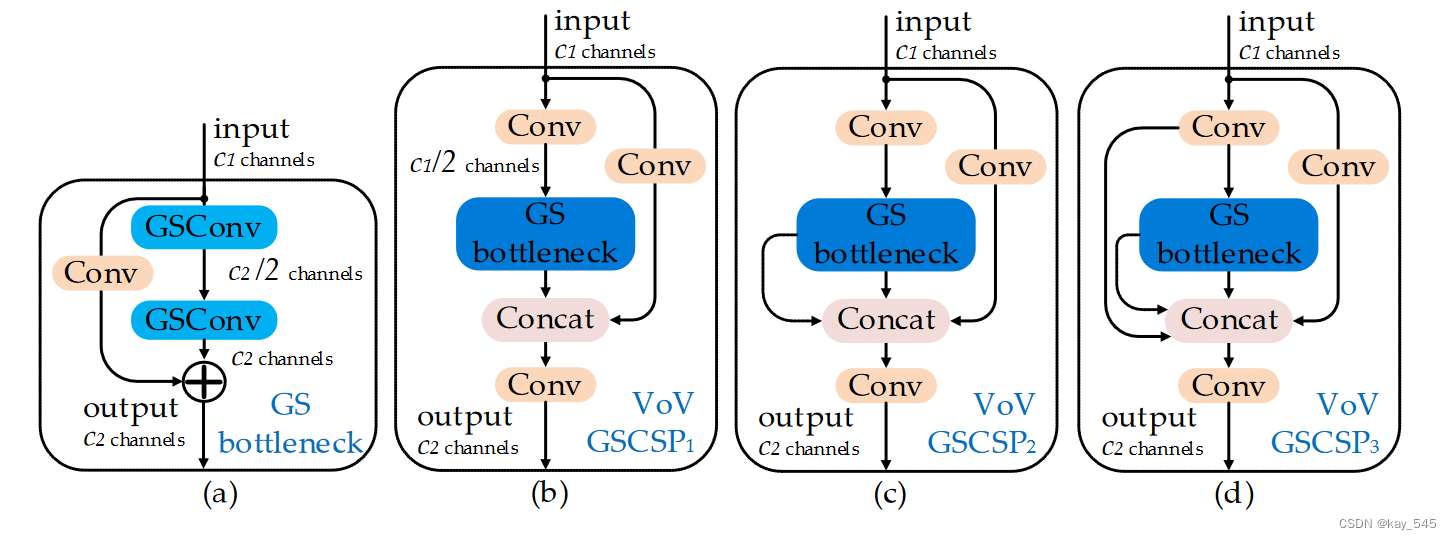

slim-neck代码实现
class VoVGSCSPC(VoVGSCSP):
# cheap VoVGSCSP module with GSBottleneck
def __init__(self, c1, c2, n=1, shortcut=True, g=1, e=0.5):
super().__init__(c1, c2, e)
c_ = int(c2 * e) # hidden channels
self.gsb = GSBottleneckC(c_, c_, 3, 1)
代码都添加在common.py中
yaml文件
# YOLOv5 🚀 by Ultralytics, GPL-3.0 license
# Slim-neck by GSConv: A better design paradigm of detector architectures for autonomous vehicle
# Parameters
nc: 80 # number of classes
depth_multiple: 0.33 # model depth multiple
width_multiple: 0.25 # layer channel multiple
anchors:
- [10,13, 16,30, 33,23] # P3/8
- [30,61, 62,45, 59,119] # P4/16
- [116,90, 156,198, 373,326] # P5/32
# YOLOv5 v6.0 backbone
backbone:
# [from, number, module, args]
[[-1, 1, Conv, [64, 6, 2, 2]], # 0-P1/2
[-1, 1, Conv, [128, 3, 2]], # 1-P2/4
[-1, 3, C3, [128]],
[-1, 1, Conv, [256, 3, 2]], # 3-P3/8
[-1, 6, C3, [256]],
[-1, 1, Conv, [512, 3, 2]], # 5-P4/16
[-1, 9, C3, [512]],
[-1, 1, Conv, [1024, 3, 2]], # 7-P5/32
[-1, 3, C3, [1024]],
[-1, 1, SPPF, [1024, 5]], # 9
]
# YOLOv5 v6.0 head
head:
[[-1, 1, GSConv, [512, 1, 1]],
[-1, 1, nn.Upsample, [None, 2, 'nearest']],
[[-1, 6], 1, Concat, [1]], # cat backbone P4
[-1, 3, VoVGSCSP, [512, False]], # 13
[-1, 1, GSConv, [256, 1, 1]],
[-1, 1, nn.Upsample, [None, 2, 'nearest']],
[[-1, 4], 1, Concat, [1]], # cat backbone P3
[-1, 3, VoVGSCSP, [256, False]], # 17 (P3/8-small)
[-1, 1, GSConv, [256, 3, 2]],
[[-1, 14], 1, Concat, [1]], # cat head P4
[-1, 3, VoVGSCSP, [512, False]], # 20 (P4/16-medium)
[-1, 1, GSConv, [512, 3, 2]],
[[-1, 10], 1, Concat, [1]], # cat head P5
[-1, 3, VoVGSCSP, [1024, False]], # 23 (P5/32-large)
[[17, 20, 23], 1, Detect, [nc, anchors]], # Detect(P3, P4, P5)
]
完整代码分享
本代码结合了YOLOv7的官方仓库进行改进,实现了YOLOv7 + GSconv
完整代码链接如下:
链接: https://pan.baidu.com/s/1zQgPu1lxZ4Sm3HYiCW3awg?pwd=v4m4 提取码: v4m4
如果执行代码出现如下面的样例则代表替换卷积模块成功。
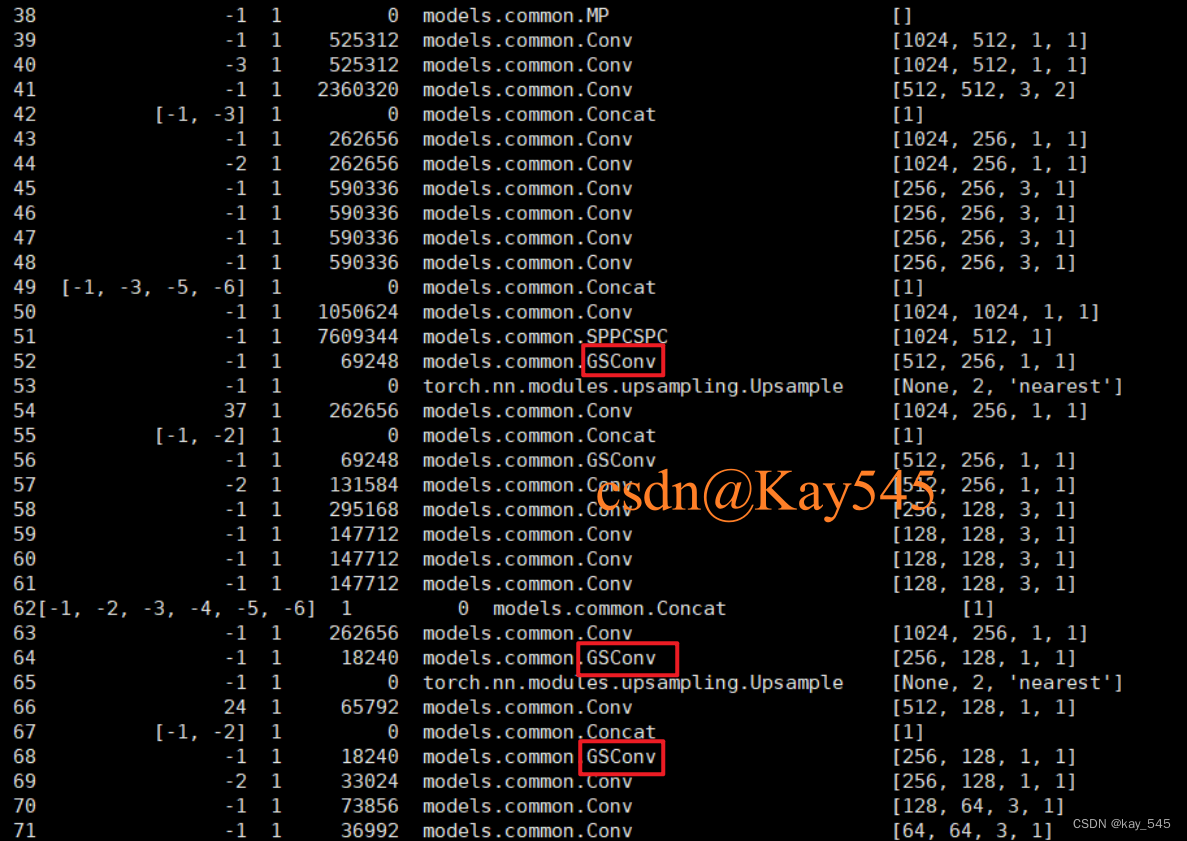
总结
本实验引入了一种新的轻量级卷积方法 GSConv,使深度可分离卷积达到接近普通卷积的效果并且更加高效。设计了一次性聚合模块 VoV-GSCSP 来代替普通的瓶颈模块以加速推理。此外,我们还提供轻量化的细颈设计范例。在我们的实验中,与其他轻量级卷积方法相比,GSConv 显示出更好的性能。