文章目录
- 一、简单介绍
- 二、步骤流程
- 2.1 预处理
- 2.2 计数
- 2.3 分割
- 2.4 添加subword
- 三、代码实现
本篇内容主要介绍如何根据提供的文本内容创建 WordPiece vocabulary,代码来自谷歌;
一、简单介绍
wordpiece
的目的是:通过考虑单词内部构造,充分利用subwords
的优势,在把长word
转化为短word
提高文字的灵活性以及提高word
转化的效率这两处之间取得一个良好的平衡;
前者会增加词表大小,后者会减少词表大小
二、步骤流程
2.1 预处理
在读取所有的文本内容后,第一步便是对文本内容预处理;
- 对英文来说,我们可以把字符都转化为小写形式,去掉accents,á 变成 a,然后利用
whitespace
和punctuation
进行分割;也就是空格和标点符号; - 对中文来说,我们可以把繁体转化为简体,分割的方式就只有单个单个字词进行分割了,而优化的方式只有从外部引入
tokenizer
对文本内容做分词,然后进行后续步骤,不然单个中文字词无法进行分解,有人可能想通过偏旁部首来,但偏旁部首如何区分顺序呢?后续的内容将围绕英文展开;
在将文本切割成以word
为单位的小块后,我们进行下一步骤;
2.2 计数
在预处理这一阶段,我们得到了以word
为单位的小块,为了了解总体words
的情况,我们需要对word
进行计数处理,并按照数量从大到小排列;如果文本内容很大,我们可以在此做一个优化,过滤掉数量太大或者太小的word
以及过滤掉长度太长的word
;
由于word piece
的本质是subwords
,为了合理的把word
转化为subwords
,我们必须考虑word
的基本单元;因为如果词表中缺少组成word
的基本单元,那么该词的表示就无法实现或者不完整和其他词照成混淆;
所以在这里我们统计所有的词其单个字符出现的次数;同理,在这里我们可以优化一下,删除出现次数较少的字符,由于出现次数较少的字符删除了,哪包含这些字符的words
也就无法表示,所以我们同时要删除包含这些字符的words
;
2.3 分割
在这一环节,我们对计数字典的word
进行分割,其处理方式如下:
首先对word
设置一个首指针和一个尾指针,以指针之间的内容求匹配计数字典和字符字典的合集,若成功,则将首指针指向尾指针,然后尾指针重新指向最后的位置,若失败,则将尾指针向首指针移动一步;直到停止首尾位置一致,若首尾在尾部则返回output_tokens
,若在其他地方则说明不能分词,返回None
实现过程如图所示:
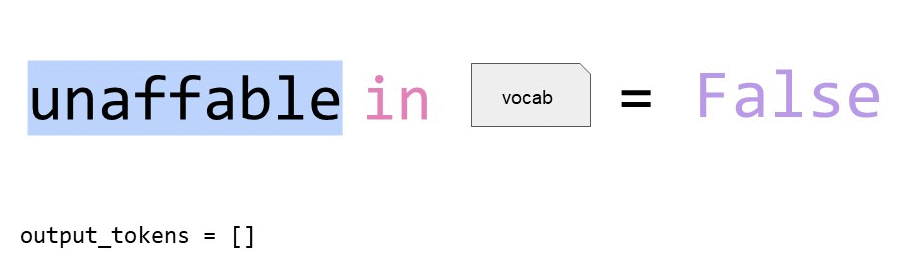
实现代码如下:
def get_split_indices(word, curr_tokens, include_joiner_token, joiner):
indices = []
start = 0
while start < len(word):
end = len(word)
while end > start:
subtoken = word[start:end]
# Subtoken includes the joiner token.
if include_joiner_token and start > 0:
subtoken = joiner + subtoken
# If subtoken is part of vocab, 'end' is a valid start index.
if subtoken in curr_tokens:
indices.append(end)
break
end -= 1
if end == start:
return 1
start = end
return indices
if __name__ == '__main__':
res = get_split_indices('hello', ['h', '##e', '##llo', '##o'], True, '##')
# print(res) res: [1, 2, 5]
2.4 添加subword
上一步分割的作用实际上是在找最大的分词块,但是其采用的是一种贪婪算法,并不是最优解;在对word
进行分割找最大的分割块的indice
之后,我们可以更快的找到常常出现在一起的字符串;处理方式如下:获取每一个以indice
位置开始,长度依次增加的subword
,构建subword
字典并计数,其每次增加的数目应该是word.count
,
这种遍历方式产生的subword
的数目过于庞大,因此如果有需要,我们需要对其进行一些优化,比如删掉一些长度较长的subword
,删除一些次数比较小的subword
,这样添加subword
的这一步骤就算完成了;
但是要注意的是,这里的subword
出现了重复计数,我们考虑了长的字符串,那么短的字符串一定会被考虑,这里我们从长字符串到短字符开始遍历,当确定长字符串有一定数目确定为vocabulary
中的元素时,我们把所有有相同前缀的短字符串减去长字符串的数目避免影响;
与此同时,vocabulary
并不一定包含了字符字典,所以我们需要将其进行合并,最后得到的vocabulary
就是wordpiece vocabulary
三、代码实现
首先我们对word进行预处理,这里代码省略;
在这里我们传入一个iterable
迭代器,然后用collections
库中的Counter
,对每个词进行计数;
def count_words(iterable) -> collections.Counter:
"""Converts a iterable of arrays of words into a `Counter` of word counts."""
counts = collections.Counter()
for words in iterable:
# Convert a RaggedTensor to a flat/dense Tensor.
words = getattr(words, 'flat_values', words)
# Flatten any dense tensor
words = np.reshape(words, [-1])
counts.update(words)
# Decode the words if necessary.
example_word = next(iter(counts.keys()))
if isinstance(example_word, bytes):
counts = collections.Counter(
{word.decode('utf-8'): count for word, count in counts.items()})
return counts
根据当前词频以及upper_thresh
和lower_thresh
确定词频的界限;
def get_search_threshs(word_counts, upper_thresh, lower_thresh):
"""Clips the thresholds for binary search based on current word counts.
The upper threshold parameter typically has a large default value that can result in many iterations of unnecessary search. Thus we clip the upper and lower bounds of search to the maximum and the minimum wordcount values.
Args: word_counts: list of (string, int) tuples upper_thresh: int, upper threshold for binary search lower_thresh: int, lower threshold for binary search
Returns: upper_search: int, clipped upper threshold for binary search lower_search: int, clipped lower threshold for binary search """
counts = [count for _, count in word_counts]
max_count = max(counts)
min_count = min(counts)
if upper_thresh is None:
upper_search = max_count
else:
upper_search = max_count if max_count < upper_thresh else upper_thresh
if lower_thresh is None:
lower_search = min_count
else:
lower_search = min_count if min_count > lower_thresh else lower_thresh
return upper_search, lower_search
对单个的char的数量设置一个上限;
def get_allowed_chars(all_counts, max_unique_chars):
"""Get the top max_unique_chars characters within our wordcounts.
We want each character to be in the vocabulary so that we can keep splitting down to the character level if necessary. However, in order not to inflate our vocabulary with rare characters, we only keep the top max_unique_chars characters.
Args: all_counts: list of (string, int) tuples max_unique_chars: int, maximum number of unique single-character tokens
Returns: set of strings containing top max_unique_chars characters in all_counts """
char_counts = collections.defaultdict(int)
for word, count in all_counts:
for char in word:
char_counts[char] += count
# Sort by count, then alphabetically.
sorted_counts = sorted(sorted(char_counts.items(), key=lambda x: x[0]),
key=lambda x: x[1], reverse=True)
allowed_chars = set()
for i in range(min(len(sorted_counts), max_unique_chars)):
allowed_chars.add(sorted_counts[i][0])
return allowed_chars
结合all_counts
和allowed_chars
,删掉包含allowed_char
的字符,控制结果为max_input_tokens
个出现次数最大的word
def filter_input_words(all_counts, allowed_chars, max_input_tokens):
"""Filters out words with unallowed chars and limits words to max_input_tokens.
Args: all_counts: list of (string, int) tuples allowed_chars: list of single-character strings max_input_tokens: int, maximum number of tokens accepted as input
Returns: list of (string, int) tuples of filtered wordcounts """ # Ensure that the input is sorted so that if `max_input_tokens` is reached
# the least common tokens are dropped. all_counts = sorted(
all_counts, key=lambda word_and_count: word_and_count[1], reverse=True)
filtered_counts = []
for word, count in all_counts:
if (max_input_tokens != -1 and
len(filtered_counts) >= max_input_tokens):
break
has_unallowed_chars = False
for char in word:
if char not in allowed_chars:
has_unallowed_chars = True
break if has_unallowed_chars:
continue
filtered_counts.append((word, count))
return filtered_counts
获得split
的index
,要保证curr_tokens可以对word进行分割;
def get_split_indices(word, curr_tokens, include_joiner_token, joiner):
"""Gets indices for valid substrings of word, for iterations > 0.
For iterations > 0, rather than considering every possible substring, we only want to consider starting points corresponding to the start of wordpieces in the current vocabulary.
Args: word: string we want to split into substrings curr_tokens: string to int dict of tokens in vocab (from previous iteration) include_joiner_token: bool whether to include joiner token joiner: string used to indicate suffixes
Returns: list of ints containing valid starting indices for word """
indices = []
start = 0
while start < len(word):
end = len(word)
while end > start:
subtoken = word[start:end]
# Subtoken includes the joiner token.
if include_joiner_token and start > 0:
subtoken = joiner + subtoken
# If subtoken is part of vocab, 'end' is a valid start index.
if subtoken in curr_tokens:
indices.append(end)
break
end -= 1
if end == start:
return None
start = end
return indices
进行最后的步骤;
import collections
from typing import List, Optional
Params = collections.namedtuple('Params', [
'upper_thresh', 'lower_thresh', 'num_iterations', 'max_input_tokens',
'max_token_length', 'max_unique_chars', 'vocab_size', 'slack_ratio',
'include_joiner_token', 'joiner', 'reserved_tokens'
])
def extract_char_tokens(word_counts):
"""Extracts all single-character tokens from word_counts.
Args: word_counts: list of (string, int) tuples
Returns: set of single-character strings contained within word_counts """
seen_chars = set()
for word, _ in word_counts:
for char in word:
seen_chars.add(char)
return seen_chars
def ensure_all_tokens_exist(input_tokens, output_tokens, include_joiner_token,
joiner):
"""Adds all tokens in input_tokens to output_tokens if not already present.
Args: input_tokens: set of strings (tokens) we want to include output_tokens: string to int dictionary mapping token to count include_joiner_token: bool whether to include joiner token joiner: string used to indicate suffixes
Returns: string to int dictionary with all tokens in input_tokens included """
for token in input_tokens:
if token not in output_tokens:
output_tokens[token] = 1
if include_joiner_token:
joined_token = joiner + token
if joined_token not in output_tokens:
output_tokens[joined_token] = 1
return output_tokens
def get_search_threshs(word_counts, upper_thresh, lower_thresh):
"""Clips the thresholds for binary search based on current word counts.
The upper threshold parameter typically has a large default value that can result in many iterations of unnecessary search. Thus we clip the upper and lower bounds of search to the maximum and the minimum wordcount values.
Args: word_counts: list of (string, int) tuples upper_thresh: int, upper threshold for binary search lower_thresh: int, lower threshold for binary search
Returns: upper_search: int, clipped upper threshold for binary search lower_search: int, clipped lower threshold for binary search """
counts = [count for _, count in word_counts]
max_count = max(counts)
min_count = min(counts)
if upper_thresh is None:
upper_search = max_count
else:
upper_search = max_count if max_count < upper_thresh else upper_thresh
if lower_thresh is None:
lower_search = min_count
else:
lower_search = min_count if min_count > lower_thresh else lower_thresh
return upper_search, lower_search
def get_input_words(word_counts, reserved_tokens, max_token_length):
"""Filters out words that are longer than max_token_length or are reserved.
Args: word_counts: list of (string, int) tuples reserved_tokens: list of strings max_token_length: int, maximum length of a token
Returns: list of (string, int) tuples of filtered wordcounts """
all_counts = []
for word, count in word_counts:
if len(word) > max_token_length or word in reserved_tokens:
continue
all_counts.append((word, count))
return all_counts
def generate_final_vocabulary(reserved_tokens, char_tokens, curr_tokens):
"""Generates final vocab given reserved, single-character, and current tokens.
Args: reserved_tokens: list of strings (tokens) that must be included in vocab char_tokens: set of single-character strings curr_tokens: string to int dict mapping token to count
Returns: list of strings representing final vocabulary """
sorted_char_tokens = sorted(list(char_tokens))
vocab_char_arrays = []
vocab_char_arrays.extend(reserved_tokens)
vocab_char_arrays.extend(sorted_char_tokens)
# Sort by count, then alphabetically.
sorted_tokens = sorted(sorted(curr_tokens.items(), key=lambda x: x[0]),
key=lambda x: x[1], reverse=True)
for token, _ in sorted_tokens:
vocab_char_arrays.append(token)
seen_tokens = set()
# Adding unique tokens to list to maintain sorted order.
vocab_words = []
for word in vocab_char_arrays:
if word in seen_tokens:
continue
seen_tokens.add(word)
vocab_words.append(word)
return vocab_words
def learn_with_thresh(word_counts, thresh, params):
"""Wordpiece learning algorithm to produce a vocab given frequency threshold.
Args: word_counts: list of (string, int) tuples thresh: int, frequency threshold for a token to be included in the vocab params: Params namedtuple, parameters for learning
Returns: list of strings, vocabulary generated for the given thresh """
# Set of single-character tokens.
char_tokens = extract_char_tokens(word_counts)
curr_tokens = ensure_all_tokens_exist(char_tokens, {},
params.include_joiner_token,
params.joiner)
for iteration in range(params.num_iterations):
subtokens = [dict() for _ in range(params.max_token_length + 1)]
# Populate array with counts of each subtoken.
for word, count in word_counts:
if iteration == 0:
split_indices = range(1, len(word) + 1)
else:
split_indices = get_split_indices(word, curr_tokens,
params.include_joiner_token,
params.joiner)
if not split_indices:
continue
start = 0
for index in split_indices:
for end in range(start + 1, len(word) + 1):
subtoken = word[start:end]
length = len(subtoken)
if params.include_joiner_token and start > 0:
subtoken = params.joiner + subtoken
if subtoken in subtokens[length]:
# Subtoken exists, increment count.
subtokens[length][subtoken] += count
else:
# New subtoken, add to dict.
subtokens[length][subtoken] = count
start = index
next_tokens = {}
# Get all tokens that have a count above the threshold.
for length in range(params.max_token_length, 0, -1):
for token, count in subtokens[length].items():
if count >= thresh:
next_tokens[token] = count
# Decrement the count of all prefixes.
if len(token) > length: # This token includes the joiner.
joiner_len = len(params.joiner)
for i in range(1 + joiner_len, length + joiner_len):
prefix = token[0:i]
if prefix in subtokens[i - joiner_len]:
subtokens[i - joiner_len][prefix] -= count
else:
for i in range(1, length):
prefix = token[0:i]
if prefix in subtokens[i]:
subtokens[i][prefix] -= count
# Add back single-character tokens.
curr_tokens = ensure_all_tokens_exist(char_tokens, next_tokens,
params.include_joiner_token,
params.joiner)
vocab_words = generate_final_vocabulary(params.reserved_tokens, char_tokens,
curr_tokens)
return vocab_words
def learn_binary_search(word_counts, lower, upper, params):
"""Performs binary search to find wordcount frequency threshold.
Given upper and lower bounds and a list of (word, count) tuples, performs binary search to find the threshold closest to producing a vocabulary of size vocab_size.
Args: word_counts: list of (string, int) tuples lower: int, lower bound for binary search upper: int, upper bound for binary search params: Params namedtuple, parameters for learning
Returns: list of strings, vocab that is closest to target vocab_size """ thresh = (upper + lower) // 2
current_vocab = learn_with_thresh(word_counts, thresh, params)
current_vocab_size = len(current_vocab)
# Allow count to be within k% of the target count, where k is slack ratio.
slack_count = params.slack_ratio * params.vocab_size
if slack_count < 0:
slack_count = 0
is_within_slack = (current_vocab_size <= params.vocab_size) and (
params.vocab_size - current_vocab_size <= slack_count)
# We've created a vocab within our goal range (or, ran out of search space).
if is_within_slack or lower >= upper or thresh <= 1:
return current_vocab
current_vocab = None
if current_vocab_size > params.vocab_size:
return learn_binary_search(word_counts, thresh + 1, upper, params)
else:
return learn_binary_search(word_counts, lower, thresh - 1, params)
整合:
def learn(word_counts,
vocab_size: int,
reserved_tokens: List[str],
upper_thresh: Optional[int] = int(1e7),
lower_thresh: Optional[int] = 10,
num_iterations: int = 4,
max_input_tokens: Optional[int] = int(5e6),
max_token_length: int = 50,
max_unique_chars: int = 1000,
slack_ratio: float = 0.05,
include_joiner_token: bool = True,
joiner: str = '##') -> List[str]:
"""Takes in wordcounts and returns wordpiece vocabulary.
Args: word_counts: (word, count) pairs as a dictionary, or list of tuples. vocab_size: The target vocabulary size. This is the maximum size. reserved_tokens: A list of tokens that must be included in the vocabulary. upper_thresh: Initial upper bound on the token frequency threshold. lower_thresh: Initial lower bound on the token frequency threchold. num_iterations: Number of iterations to run. max_input_tokens: The maximum number of words in the initial vocabulary. The words with the lowest counts are discarded. Use `None` or `-1` for "no maximum". max_token_length: The maximum token length. Counts for longer words are discarded. max_unique_chars: The maximum alphabet size. This prevents rare characters from inflating the vocabulary. Counts for words containing characters ouside of the selected alphabet are discarded. slack_ratio: The maximum deviation acceptable from `vocab_size` for an acceptable vocabulary. The acceptable range of vocabulary sizes is from `vocab_size*(1-slack_ratio)` to `vocab_size`. include_joiner_token: If true, include the `joiner` token in the output vocabulary. joiner: The prefix to include on suffix tokens in the output vocabulary. Usually "##". For example 'places' could be tokenized as `['place', '##s']`.
Returns: string, final vocabulary with each word separated by newline """ if isinstance(word_counts, dict):
word_counts = word_counts.items()
params = Params(upper_thresh, lower_thresh, num_iterations, max_input_tokens,
max_token_length, max_unique_chars, vocab_size, slack_ratio,
include_joiner_token, joiner, reserved_tokens)
upper_search, lower_search = get_search_threshs(word_counts,
params.upper_thresh,
params.lower_thresh)
all_counts = get_input_words(word_counts, params.reserved_tokens,
params.max_token_length)
allowed_chars = get_allowed_chars(all_counts, params.max_unique_chars)
filtered_counts = filter_input_words(all_counts, allowed_chars,
params.max_input_tokens)
vocab = learn_binary_search(filtered_counts, lower_search, upper_search,
params)
return vocab